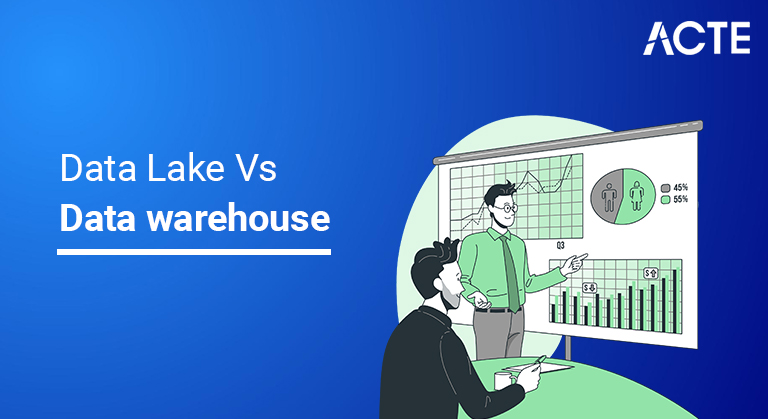
What is a Data Warehouse?
A Data Warehouse is a huge storehouse of hierarchical information collected from a wide scope of functional and outside information sources. The information is organized, separated, and right now handled for a particular reason. Information stockrooms intermittently pull handled information from different inward applications and outside accomplice frameworks for cutting edge questioning and examination. Medium and huge size organizations use information stockroom fundamentals to share information and content across office explicit data sets. The motivation behind an information distribution center can be to store data about items, orders, clients, stock, representatives, and so on
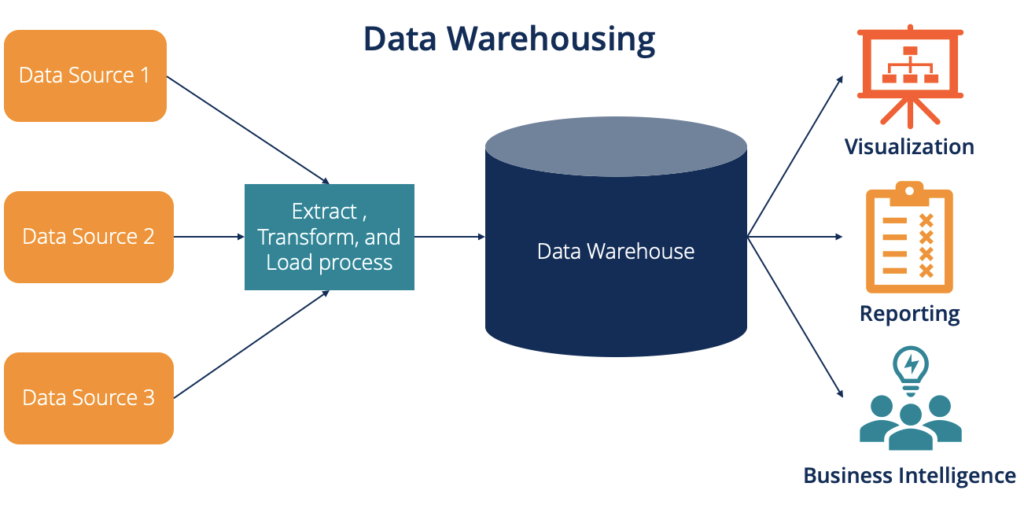
What is a Data Lake?
An information lake definition clarifies it as a profoundly adaptable information stockpiling region to store a lot of crude information in its unique arrangement until it is needed for use. An information lake can store a wide range of information with no proper impediment on account size or record and with no particular reason characterized at this point. The information comes from divergent sources and can be organized, semi-organized, or even unstructured. Information lake information can be questioned on a case by case basis. Organizations that need to gather and store a huge volume of information — without expecting to process or dissect every last bit of it promptly — utilize the information lake idea for fast stockpiling without change.
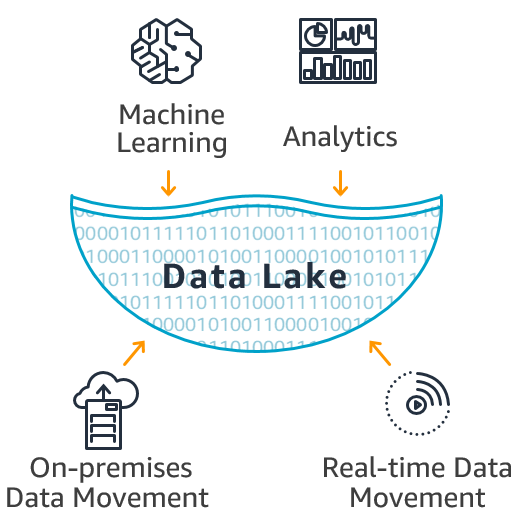
Top 6 Difference Between Data Lake and Data Warehouse :-
Information Structure : An information stockroom can store information that has been handled and refined. Information lakes, then again, store crude information that has not been handled for a reason yet. Subsequently, information lakes require a lot bigger capacity limit than information stockrooms; the information is adaptable, immediately broke down, and ideal for AI.
Handling : An information stockroom utilizes a pattern on-compose way to deal with handled information to give it shape and construction. An information lake utilizes pattern on-read on crude information to handle it.
Cost : Putting away in an information stockroom can be exorbitant, especially on the off chance that there is an enormous volume of information. An information lake is a less expensive choice intended for minimal expense information stockpiling. This clarifies why information lake is liked by many organizations.
Reason : Information distribution centers just hold handled information that has been utilized for a particular reason. One of the advantages of an information stockroom is that extra room isn’t squandered on information that may not be utilized. Information lake stores crude information that can some of the time have a particular future use and now and again only for accumulating. Subsequently, information is less coordinated and separated in the information lake.
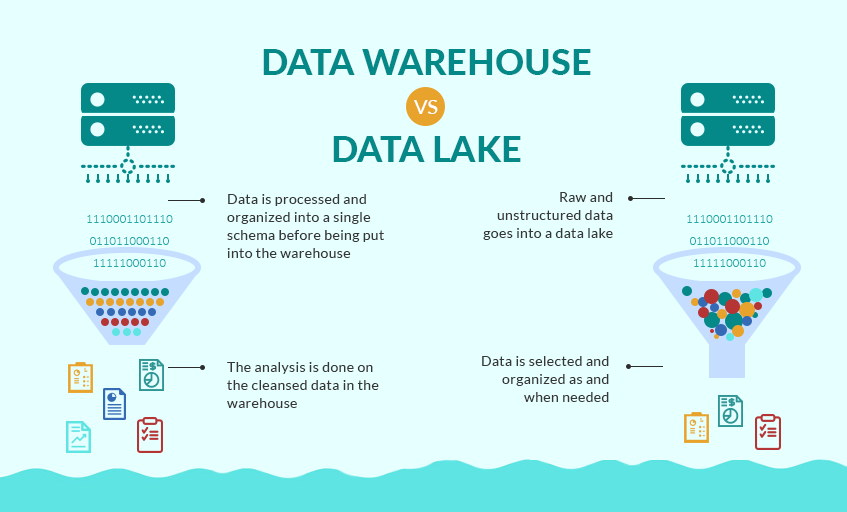
Clients : Information stockrooms are utilized generally by IT or business experts who know about the point addressed in the handled information utilized. The unstructured information in information lakes typically require information researchers or architects for getting sorted out information lakes prior to putting the information to utilize.
Availability : Information distribution centers are organized by configuration, making them hard to get to and control. Conversely, information lakes have not many constraints and are not difficult to access and change. Information can be refreshed rapidly. This considers one of the key information lake benefits.
Factors that Drive the Data Warehouse vs Data Lake Decision :-
1) Data Warehouse versus Data Lake: Data Shape
Information Lakes contain data in normal and natural arrangement. This information can either be organized or unstructured. While, Data Warehouses contain organized and semi-organized information that have been cleaned and handled, prepared for vital investigation dependent on predefined business needs.
2) Data Warehouse versus Data Lake: Data Quality
Since information put away in Data Lakes is in its local structure, the nature of the information is low. Information Lakes can comprise of key-esteem record designs, succession records (.seq) that comprise of double key-esteem sets, sound, video, and reports. These crude and natural information are generally unsuitable for Data Analysis and Machine Learning models.
Yet, by utilizing ETL strategies, Data Warehouses accomplish top notch organized or semi-organized information through accumulation, speculation, and standardization. The information components in the Data Warehouse include quantitative measurements and the traits that portray them, making them reasonable for creating experiences right away.
3) Data Warehouse versus Data Lake: Schema
Information Lakes support different configurations of information, yet this bothered information typically doesn’t have any blueprint. An outline can be seen as an organized association of different tables and their fields, information types, requirements, and connections. Be that as it may, in Data Lakes, the blueprint is characterized, created, and custom-made according to client needs at the hour of perusing. All in all, Data Lakes follow the Schema-on-Read approach. This methodology offers shape and design to the information just when required.
Dissimilar to Data Lakes, Data Warehouses influence the Schema-on-Write approach. In this, the pattern is characterized prior to putting away information utilizing forthright Data Modeling. Distinguishing every one of the segments and columns ahead of time is critical to guaranteeing that organized information is set precisely where it should be.
Therefore, information put away in the Data Warehouse as of now has a predefined design and outline. Since the information is coordinated with a legitimate construction, clients can undoubtedly explore and decipher the information in Warehouses. In any case, clients should know about the outline definition utilized while getting ready Data Visualization and announcing. Not after the mapping can bring about accumulating off base bits of knowledge.
4) Data Warehouse versus Data Lake: Modeling
Information Lakes will quite often have a compliment model. This implies there might be different data about a substance yet may not be connected with each other. Consequently, any progressions made in one would not reflect in the other. For instance, consider a business profile record. The record might contain data about deals made, current and completed ventures, market projections, business devices created, and so on While every one of this data might be essential to the entrepreneur, they may not be connected.
For Data Warehouses, a few Data Modeling procedures like layered and social are carried out for smoothing out the information examination processes. Therefore, it is normal for Data Warehouses to be developed with a few tables that can be combined to give experiences.
5) Data Warehouse versus Data Lake: Users
Information Scientists for the most part use Data Lakes to remove unstructured information to distinguish designs and helpful data that can be utilized for improving Artificial Intelligence-based items and administrations. In the mean time, Data Warehouses are essentially utilized by Business Analysts to make representations and reports. It is liked by organizations searching for all around organized and reason fabricated framework for Data Analytics. Indeed, even non-specialists can use information for settling on educated choices with the assistance regarding simplified information examination devices.
Specialties like Healthcare, Marketing, Education manage information where the volume of organized information is extremely low. Here Data Lake will be an ideal fit. In any case, this doesn’t imply that these associations just utilized Data Lakes. Information Warehouses are still broadly utilized in these associations for examination with organized information.
6) Data Warehouse versus Data Lake: Pricing
Information Lakes lie on the minimal expense end of the evaluating range since it isn’t execution driven. The thought behind sending a Data Lake is to store a goliath measure of information whose reason for existing is not really set in stone. Information in Data Lakes can consume the space for quite a long time before it is utilized for Machine Learning or Data Warehousing. Subsequently, Data Lakes are streamlined to decrease the expense of putting away crude data.
Then again, Data Warehouses are intended to help different Analytics needs inside associations. They need to help serious execution for working with quality information for investigation and bits of knowledge age. This makes Data Warehouses costlier than Data Lakes. In any case, the Return On Investment from Data Warehouses can be monstrous on the off chance that associations devise an ideal arrangement. A few organizations send a manual labor force for ETL works on, expanding the expense of keeping up with Data Warehouses. Yet, with no-code ETL arrangements, associations can smooth out the whole course of Data Warehousing to lessen functional expenses.
7) Data Warehouse versus Data Lake: Security
The Database administrators construct models that award Data Warehouse consent to just approved faculty. These security models additionally ensure Databases, Schemas from any maverick changes to stay away from breakdowns in the information stream. Security and organization of Data Warehouses are key for associations to consent to a few Data Privacy rules from across the world. The equivalent can’t be said with regards to a Data Lake.
Numerous clients, applications, or even outsiders expect admittance to Data Lakes, accordingly compromising security. On the more brilliant side, as the significance of consistence prerequisites for each sort of information is expanding, better safety efforts may be implemented on Data Lake frameworks.
8) Data Warehouse versus Data Lake: Accessibility
The availability of Data Repositories is estimated by the way that well they can be utilized overall, not by the information inside. The absence of outline makes Data Lake more open and adaptable for getting information for complex examination. Notwithstanding, no one but specialists can pull Raw Data and pre-process it. By and by, it upholds a wide scope of Data Analysis that goes past the standard extent of associations’ functional requirements. Dissimilar to Data Lakes, the Data Architecture in Data Warehouses is exceptionally organized, making it more confounded and requiring more expense to roll out any improvements.
Conclusion :-
This article gave a complete examination of the 2 well known Database Storages in the market today: Data Warehouses and Data Lakes. It likewise gave a short outline of the two strategies. It additionally gave the boundaries to pass judgment on every one of the capacity methods. By and large, the Data Warehouse versus Data Lake decision exclusively relies upon the objective of the organization and the assets it has.