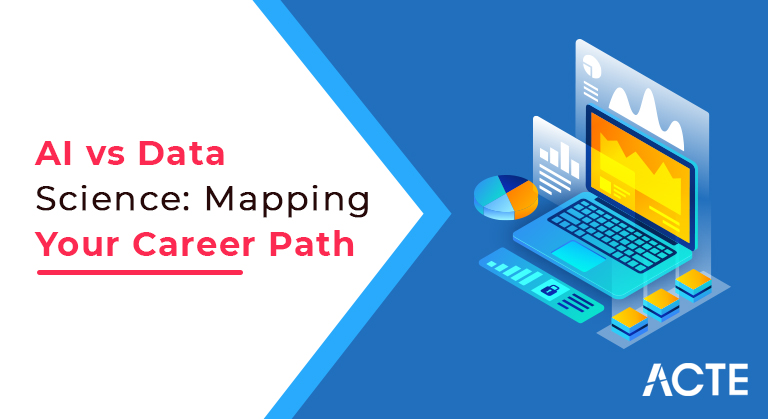
Data Science is a comprehensive process that involves pre-processing, analysis, visualization and prediction. On the other hand, AI is the implementation of a predictive model to forecast future events. Data Science comprises of various statistical techniques whereas AI makes use of computer algorithms.
- Introduction to AI vs Data Science
- Leading AI vs Data Science Jobs and Skill Requirements
- Considering the Stops Along the Road
- Your Career Path Starts Here
- Clearing Up the Data Science vs. AI Confusion
- Industry Demand Creates Career Choices
- Map Your Learning Path to Your Desired Career
- How to choose the right career path
- Technical Skills required for AI-ML Roles
- Conclusion
- When it comes to Data Science and Artificial Intelligence (AI), you will often find a lot of intersections between the two skill paths. There are many subsets of AI, such as machine learning and deep learning, and data science uses these techniques to interpret and analyse data, find patterns, make predictions, and generate insights. Therefore, it can be difficult to decide between AI vs Data Science.
- On the other hand, technologies like ML rely on strong data science practises to ensure clean, high quality, and relevant data is training ML algorithms and systems. Not to mention that data science is an interdisciplinary field that often includes knowledge of AI and ML, and like an AI engineer, many AI careers require data scientist skills.
- So it’s easy to start wondering—where to begin? This is an especially pressing question for those who understand that the demand for data science and AI skills is skyrocketing and want to join the board. There is no right or wrong answer or fundamental hierarchy. But the significant difference in knowledge and skills required for some job roles will ultimately shape your competencies and your career projection journey.
Introduction to AI vs Data Science:
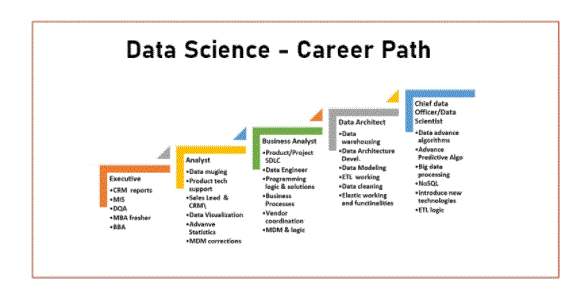
Leading AI vs Data Science Jobs and Skill Requirements:
A good place to start is deciding what kind of career is best for you, and then trying to meet the respective needs and skill sets of that particular career path. Also, examine the qualifications required by the occupation for specific positions, such as the type of degree or “soft” skills such as being a great communicator. If you are unsure of the career path you want to take – AI vs Data Science – work backwards, looking at the respective skill sets required for each path, and then decide on the career option that best suits you. fulfils. or is interested in development.
The following are three of the most sought-after job positions in the field of AI vs. Data Science. By understanding the job requirements and the organisation’s essential qualifications, you can pinpoint your skills and interests in these particular areas to better visualise your unique career path.
Machine learning engineer
Requires a strong understanding of programming languages, mathematics, analytical skills, understanding of data sets and development tools. Also, most organisations require you to have a master’s or doctorate in computer science or mathematics.
Statistics scientist
Statistical analysis, an understanding of big data platforms like Hadoop, programming languages as well as strong communication, analytical skills and business knowledge are important.
Business intelligence developer
Great communication and problem-solving skills, ability to analyse complex data sets to identify market trends, knowledge of BI technologies, and certification in data science are recommended.
- When deciding between AI vs data science skills, first of all, you need to consider what your career goals are and whether you want to expand your competencies, improve your existing knowledge base, or expand your business. Want to help take us in a new direction through digital. Change.
- For those who aren’t sure where to start, data science and AI both have similar skills and knowledge baselines: statistics, maths, and programming. These are solid foundational learning paths that leave the door open to a data science or AI-focused career path.
- Then start exploring some different AI and Machine Learning courses. If this interests you, start looking into AI-related courses like programming languages, coding, data modelling, algorithms, or visualisation.
- If you find yourself more interested in the analysis and business side of learning, the data science educational path is your starting point. Start shaping your skills in data mining or wrangling, data modelling, database management and programming languages such as Python and R.
Considering the Stops Along the Road:
- Understanding where you want to be with your career and what you want to use your skills for will help you decide which educational path – AI vs. Data Science – is right for you. Choose a career that best suits your skills, interests, and potential for industry-leading, in-demand positions, so you know how to start down the right career path for you.
- ACTE is a leading educational provider for all disciplines in the fields of AI, ML, Data Science and Analytics, and provides comprehensive resources and education programs for industry professionals at all levels of their career journey.
Your Career Path Starts Here:
- Ronald comments on the confusion that is common among learners who are considering careers in data science, AI, and machine learning. It’s not always clear where to start with the best foundation for a career in these fields. Ronald pointed out that a Gartner study predicts that by 2021 (in other words, now), 80 percent of emerging technologies will have an AI foundation, and IDC predicts that 75 percent of commercial applications will have an AI component. As far as data science is concerned, 45 percent of firms have preferred data science and analytics even in the post-pandemic era. He said AI and related technologies such as machine learning (ML), virtual reality (VR), and augmented reality (AR) all depend on data.
- Are you considering a career in AI and Data Science? So get certified with an Artificial Intelligence and Data Science course today!This is the reason why both the sectors are experiencing rapid employment growth. Jobs for data scientists and engineers have grown by more than 35 percent over the past five years, and these jobs have topped the LinkedIn emerging job list for three years. AI hiring has grown even more rapidly – 74 percent over the past four years. Ronald saw that you can’t go wrong choosing a career in any field. Cyber security has also seen a growth of over 30 percent in the last year. This growth has only been reinforced by the shift to digital operations due to the pandemic.
- For all the overlap between AI and Data Science, there are significant differences. Data science supports making inferences and predictions from data, and it drives insights through statistical methods, pattern recognition, and data visualisation. AI adds a robust scientific processing component that allows systems to make inferences and predictions with machines using algorithms to directly use the products of data science, rather than interpreting the data products themselves.
Clearing Up the Data Science vs. AI Confusion:
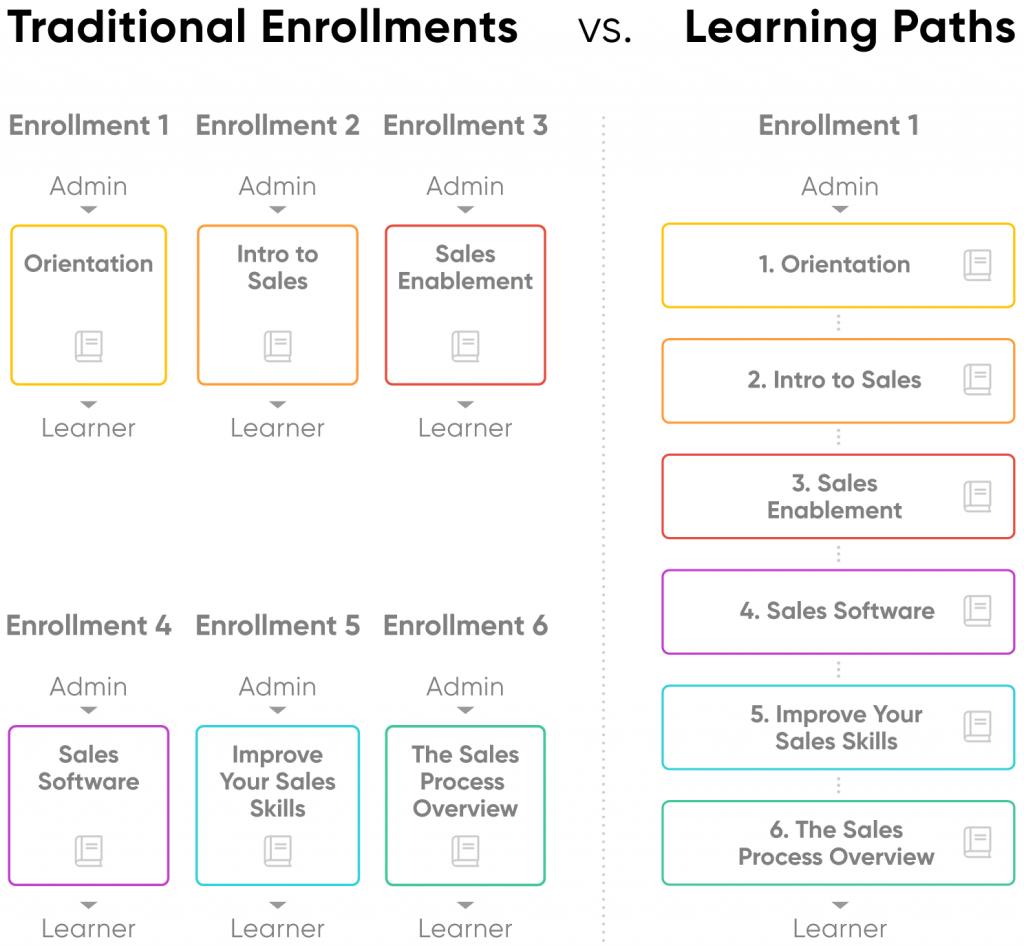
- Production
- Energy
- Finance
- E-commerce
- Healthtech
- Education
- Technology
- Statistics scientist
- BI developer
- Research scientist
- Business Analyst
- Data architect
- Machine learning engineer
- AI Architect
- Robotics engineer
- Computer vision engineer
- Full stack engineer
- Neural network developer
- Cloud engineer
Industry Demand Creates Career Choices:
Ronald observed that almost every industry demands data science and AI talent for emerging applications. He cited examples:
These examples range from AI and data science to predict potential failures of manufacturing machines and power distribution networks to schedule preventive maintenance, to Pokémon Go using AR to enable game play in the real world, to new applications. To discover are for drug discovery “in silico”. Pharmaceutical compounds through AI simulation.
This has given rise to many different careers in data science and AI, including:
With options like this, it is important to choose a good starting point to build a foundation for a career in data science and AI. Data Science and AI both require a foundation in mathematics, statistics, and programming. With that groundwork done, you can choose to branch in your preferred direction. For those more interested in analytics and business, shape your skills in data mining, data wrangling, data modelling, database management, and programming languages. such as Python and R. For those more interested in AI and ML, explore various machine learning courses and branch out. From there courses related to AI like coding, data modelling, programming languages, algorithms and visualisation.
- Your learning path should support the career path you wish to pursue. One way to map your learning path is to work backwards from career to career to see the skill sets you need for each of those careers. Then assess your abilities and interests: What skills are you best suited for, and what skills are you most interested in learning? Look for careers that have the necessary skills to match your qualifications and interests.
- Then look for educational programs that will give you those skills. Consider what kind of education and how much education employers require for these jobs: will you need a college degree, advanced degree, or even a doctorate? Or will you be able to demonstrate the skills and training required through certification programs?
- Ronald reminded the audience that soft skills are also very important for a career in data science and AI. He recommends that you develop communication skills, storytelling abilities and business acumen so that you can explain to your managers and executives the importance of your models and analysis and understand their business needs.
- Ronald cited specific learning paths for two careers. An aspiring data scientist will take data science courses, statistics, analytics, computer science and electrical engineering. The learner will then gain proficiency in coding skills and experience in Python, R, and/or other programming languages. The next step is to refine skills in SQL and ML techniques such as classification or neural networks. A data scientist typically needs a BA or higher degree in statistics, computer science, or maths, and will need lifelong ongoing skills training and education.
- On the AI side, a machine learning engineer will begin with courses in programming skills such as Python, R, C++, Octave, and mathematics such as calculus and linear algebra, and data modelling. The learner will then gain proficiency in computer science and programming, such as computer architecture, data structures, algorithms, and software engineering and systems design. Again, the ML engineer typically needs a higher education degree (BA, master’s, PhD), and will need lifelong ongoing skills training and education.
- Ronald took several questions from the live audience. Watch the webinar replay above to hear these questions and Ronald’s answers. For more simple digital marketing resources, including articles and eBooks, look here. And if you are ready to start gaining specific skills and certification for your career, then Courses and Master Programs SimpleLearn Offers in the areas of Data Science and AI and Machine Learning with PG Programs in Data Science and AI and Machine Learning Courses See.
Map Your Learning Path to Your Desired Career:
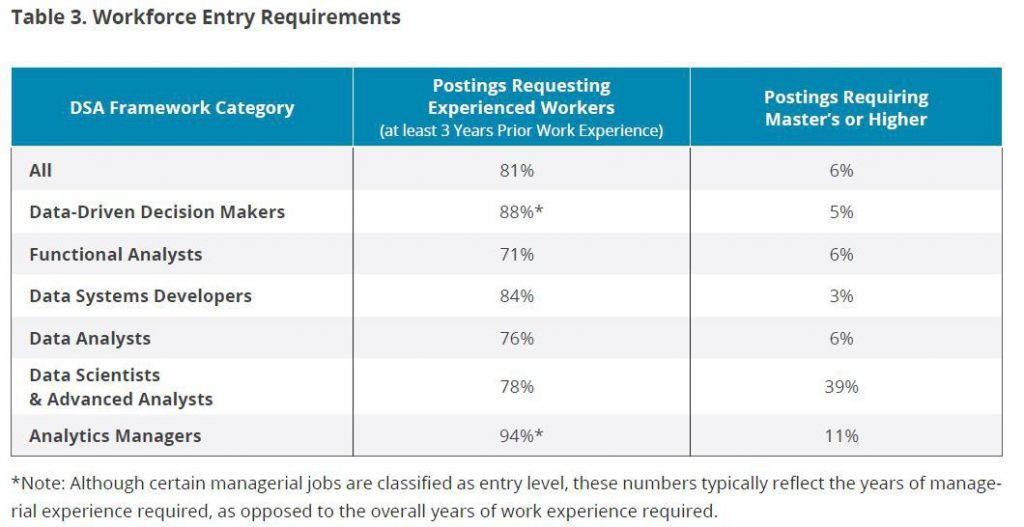
- A machine learning engineer must have a thorough understanding of multiple programming languages as well as AI programming. This role applies predictive models and makes efficient use of natural language processing to deal with large-scale datasets.
- Experience with agile development practises as well as software development IDE tools like IntelliJ and Eclipse and in-depth practical knowledge of programming languages like Scala, Python, Java is required. Analytical skills, experience in neural networks and deep learning, as well as cloud applications, are an added plus.
- A data scientist deals with extremely large and complex datasets using both machine learning as well as predictive analytics. The skill set for creating algorithms that enable the gathering as well as cleanup of such a large amount of data, making preparedness to analyse it is critical. Working knowledge of big data tools and platforms like MapReduce, Hive, Pig, Spark, Hadoop is essential. Experience in statistical computing, as well as programming languages such as Scala, Perl, SQL, Python, is also demanded.
- If you want to become an AI developer specifically in the data science field, you may need an advanced degree in computer science, otherwise, any higher degree will suffice, be it electrical engineering or mathematics.
- A business intelligence developer analyzes complex datasets to identify market trends along with the business to boost the organisation’s revenue. Using a cloud-based data platform, your task will be to design, model, and simultaneously maintain complex data.
- An ideal candidate should have experience in Data Mining, Data Warehouse Design, SQL Integration Services, SQL Queries, SQL Server Reporting Services as well as BI technologies.
- One of the most in-demand jobs in the field of artificial intelligence, a research scientist must have expertise in several disciplines of AI, including computational statistics, machine learning, deep learning as well as applied mathematics.
- Extensive experience in graphical models, natural language processing, and reinforcement learning and parallel computing, machine learning, distributed computing, artificial intelligence, and benchmarking is required.
- Big data engineers and architects develop and plan the entire big data environment on Spark and Hadoop systems. Experience in data mining, data migration, as well as data visualisation is essential, in addition to having demonstrable experience with Java, Python, C++ and Scala.
- Depending on the career you are looking for, whether AI or Data Science, it is important that you work backwards to acquire the necessary skills. There are many skills in common with some specialisations depending on your career choice. However, it is important to remember that this is an emerging space and the need for professionals skilled in the latest technologies is only gaining momentum.
How to choose the right career path:
How many times have you heard that data is the new oil and intelligent machines are the future? AI and Data Science are two skill-based career paths with a lot of crossroads. The building blocks of AI are mainly structured and unstructured data. Machine learning, deep learning, and other subsets of AI primarily use data science. Ideally, an AI professional should have a good understanding of data science, and a data science professional should be able to understand the basic nuances of AI. Data Science is an enabling skill set that is used by many digital technologies and applications. A grounding in data science will provide a foundation upon which many branches of digital careers can be built.
According to the recent Upgrade Report, the top five most in-demand digital roles are:
1. Machine Learning Engineer:
2. Data Scientist:
3. Business Intelligence Developer
4. Research Scientist
5. Big Data Engineer/Architect
- Knowledge of programming languages like Python, C++, Java
- Data Modelling and Evaluation
- Probability and statistics
- Distributed computing
- Machine learning algorithms
- As you can see, the skill sets requirements of both domains overlap. In most cases, courses in Data Science and AI-ML include a basic knowledge of both in addition to focusing on the respective specialisations.
- Even though the fields of Data Science vs Machine Learning vs Artificial Intelligence overlap, their specific functionalities differ and they have related areas of application. The data science market has opened up a number of services and product industries, creating opportunities for experts in this domain.
Technical Skills required for AI-ML Roles:
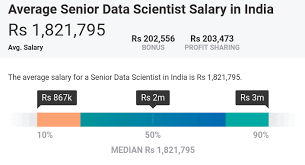
Conclusion:
First, one cannot compare two domains to decide which is better – precisely because they are two different branches of study. It is like comparing science and art. However, the apparent popularity of data science today cannot be denied. Almost all industries rely on data to make more robust business decisions. Data has become an integral part of businesses, whether for analysing performance or for device data-driven strategies or applications. Machine learning, on the other hand, is still an evolving branch that is yet to be adopted by some industries, which only says that ML technologies will have more demanding relevance in the near future. Hence, there will be equal demand for professionals in both these domains in future.
Since both machine learning and data science are closely related, a basic knowledge of each is essential to specialise in either of the domains. Having said that, getting started with machine learning requires knowledge of data analysis more than data science. Learning programming languages such as R, Python and Java requires understanding and cleaning the data to create ML algorithms. Most machine learning courses include tutorials on these programming languages and basic data analysis and data science concepts.
To put it a little differently, data science is the future. No business or industry for that matter would be able to run without data science. A large number of infections have already occurred around the world, with businesses demanding more data-driven decisions, with more to follow suit. Data science has rightly been termed as the oil of the 21st century, which can mean endless possibilities across industries. So, if you are willing to take this path, your efforts will be highly rewarded with not only a fulfilling career and *** paycheck but job security.