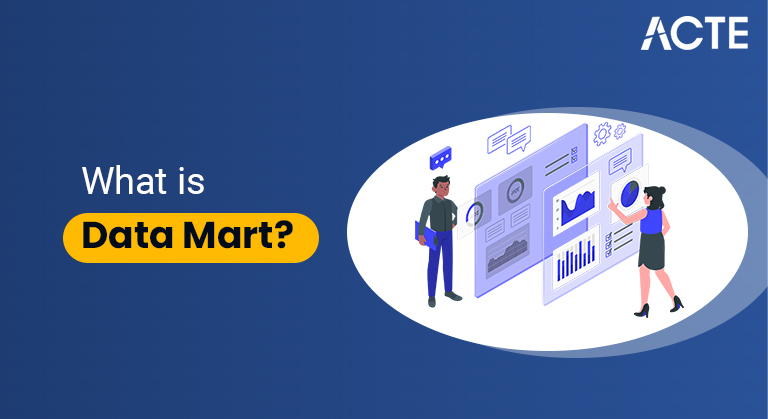
What is a Data Mart?
In a market overwhelmed by huge data and investigation, data stores are one key to productively changing data into experiences. data distribution centers ordinarily manage enormous dataal indexes, however data examination requires simple to-find and promptly accessible data. Should a finance manager need to perform complex questions just to get to the data they require for their reports? No—and that is the reason organizations savvy organizations use data stores.
An data store is a subject-situated data set that is regularly a divided portion of a venture data distribution center. The subset of data held in an data store regularly lines up with a specific specialty unit like deals, money, or promoting. data marts speed up business processes by permitting admittance to significant data in an data distribution center or functional data store in no time, rather than months or longer. Since an data store simply holds back the data relevant to a specific business region, it is a practical method for acquiring significant experiences rapidly.
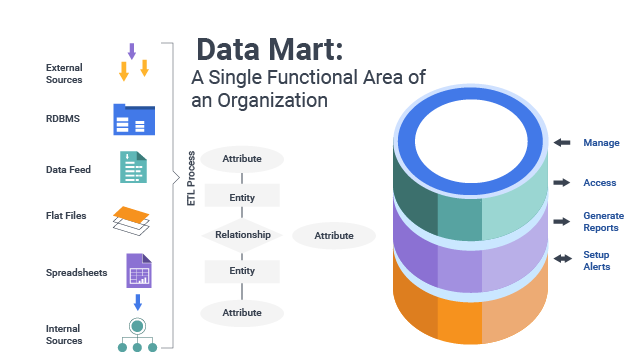
- Sensible view – A virtual table/view that is coherently—however not actually—isolated from the data stockroom
- Actual subset – Data remove that is a genuinely independent data base from the data stockroom
- Granular data—the most minimal degree of data in the objective set—in the data distribution center fills in as the single perspective for all reliant data stores that are made.
Types of Data Marts :-
There are three kinds of data stores: reliant, free, and half breed. They are arranged dependent on their connection to the data stockroom and the data sources that are utilized to make the framework.
1. Subordinate Data Marts :
A reliant data store is made from a current undertaking data stockroom. It is the hierarchical methodology that starts with putting away all business data in one focal area, then, at that point, removes an obviously characterized piece of the data when required for investigation.
To shape an data stockroom, a particular arrangement of data is collected (framed into a bunch) from the distribution center, rebuilt, then, at that point, stacked to the data mart where it very well may be questioned. It tends to be a sensible view or actual subset of the data distribution center:
2. Free Data Marts :
A free data mart is an independent framework—made without the utilization of an data stockroom—that spotlights on one branch of knowledge or business work. data is extricated from inside or outside data sources (or both), handled, then, at that point, stacked to the data mart vault where it is put away until required for business investigation.
Autonomous data marts are quite easy to plan and create. They are advantageous to accomplish momentary objectives yet may become lumbering to make due—each with its own ETL instrument and rationale—as business needs extend and turn out to be more intricate.
3. Half breed Data Marts :
A half breed data store consolidates data from a current data distribution center and other functional source frameworks. It joins the speed and end-client focal point of a hierarchical methodology with the advantages of the endeavor level incorporation of the base up strategy.
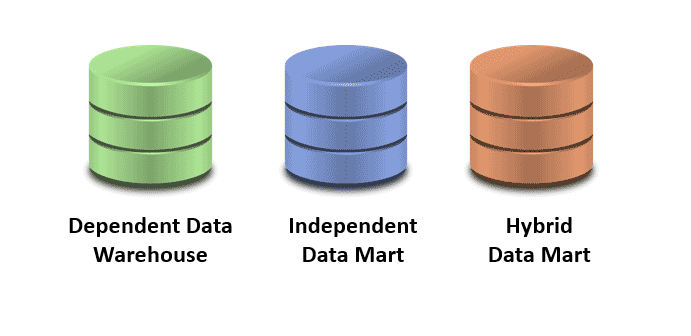
Structure of a Data Mart :-
Like an data stockroom, an data mart might be coordinated utilizing a star, snowflake, vault, or other pattern as a plan. IT groups commonly utilize a star pattern comprising of at least one reality tables (set of measurements connecting with a particular business interaction or occasion) referring to aspect tables (essential key joined to a reality table) in a social data base.
The advantage of a star composition is that less joins are required when composing questions, as there is no reliance between aspects. This works on the ETL demand process making it simpler for investigators to get to and explore.
In a snowflake mapping, aspects are not obviously characterized. They are standardized to assist with lessening data repetition and ensure data respectability. It takes less space to store aspect tables, yet it is a more convoluted construction (various tables to populate and synchronize) that can be hard to keep up with.
Benefits of a Data Mart :-
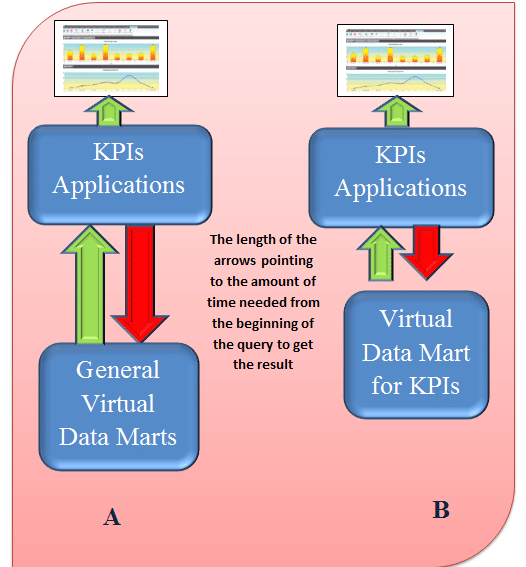
Overseeing huge data— and acquiring important business experiences—is a test all organizations face, and one that most are replying with vital data marts.
Effective access — An data mart is an efficient answer for getting to a particular arrangement of data for business knowledge.
Reasonable data stockroom elective — Data marts can be a modest option in contrast to fostering an endeavor data distribution center, where required dataal collections are more modest. A free data mart can be ready for action in a week or less.
Further develop data distribution center execution — Dependent and half breed data marts can work on the exhibition of an data stockroom by assuming the weight of handling, to address the issues of the examiner. At the point when subordinate data marts are set in a different handling office, they fundamentally lessen examination handling costs also.
Data Mart versus Data Warehouse :-
Data marts and data distribution centers are both profoundly organized storehouses where data is put away and overseen until it is required. In any case, they vary in the extent of data put away: data stockrooms are worked to fill in as the focal store of data for the whole business, while an data mart satisfies the solicitation of a particular division or business work. Since an data distribution center contains data for the whole organization, it is best practice to have stringently control who can get to it.
Furthermore, questioning the data you want in an data stockroom is a staggeringly troublesome assignment for the business. Consequently, the basic role of an data store is to segregate—or parcel—a more modest arrangement of data from an entire to give more straightforward data admittance to the end martpers.
An Data mart can be made from a current data stockroom—the hierarchical methodology—or from different sources, like inner functional frameworks or outer data. Like an data distribution center, it is a social data set that stores conditional data (time esteem, mathematical request, reference to at least one item) in sections and lines making it simple to sort out and get to.
Then again, separate specialty units might make their own data marts dependent on their own data prerequisites. On the off chance that business needs direct, numerous data marts can be consolidated to make a solitary, data stockroom. This is the base up improvement approach.
- The primary thing you do while executing an Data mart is settling on the extent of the undertaking and its plan. Since Data marts are subject-arranged data sets, this progression includes deciding a subject or a theme to which Data put away in a store will be connected.
- As well as gathering data about specialized particulars, you want to settle on business prerequisites during this stage as well. It is additionally important to recognize the Data sources connected with the subject and plan the legitimate and actual design of the Data store.
- When the extent of work is set up, here comes the second step that includes building the sensible and actual constructions of the Data store engineering planned during the main stage.
- Coherent design alludes to the situation where Data exists as virtual tables or perspectives isolated from the distribution center legitimately, not actually. Virtual Data marts might be a decent choice when assets are restricted.
- Actual construction alludes to the situation where an Data base is genuinely isolated from the stockroom. The data set might be cloud-put together or with respect to premises.
- Additionally, this progression requires the formation of the pattern objects (e.g., tables, lists) and setting up Data access structures.
- Extricating data from target Data sources, purifying and changing over Data into a fitting arrangement, and stacking Data into an Data mart.
- To play out the cycles of extraction, change, and stacking, ETL instruments are utilized.
- Setting up the middle (meta) layer for the front-end application (the layer changes over data set constructions into business terms so that end
- Customers can get to Data from Data stores without any problem);
- Setting up and overseeing data set designs like summed up tables; and
- Setting up APIs (application programming points of interaction) whenever required.
- Data marts can be gotten to by means of an order line or GUI (graphical UI), which is a more easy to use choice.
- Giving secure client admittance to Data;
- Improving and calibrating the framework for better execution;
- Adding and overseeing new Data; and
- Guaranteeing framework accessibility and arranging recuperation situations.
Data mart implementation steps :-
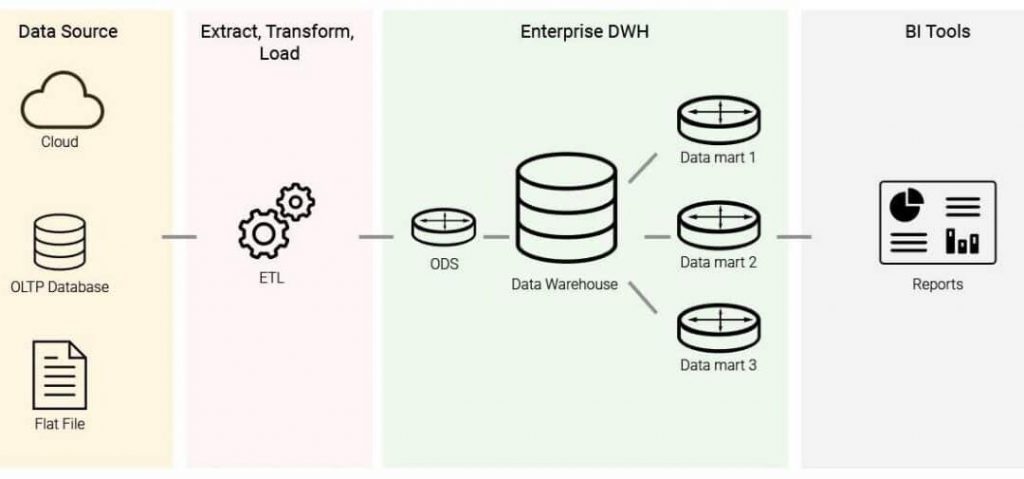
The most common way of making Data marts might be muddled and contrast contingent upon the requirements of a specific organization. As a rule, there are five center advances like planning an Data store, building it, moving Data, arranging admittance to an archive, lastly overseeing it. We’ll walk you through each progression in more detail.
Data store planning:
Data store developing:
Data moving:
The third step covers every one of the assignments connected with moving Data from sources to Data stores:
Data access designing:
Since Data is in Data marts, it’s an ideal opportunity to put it to utilize: making questions, examining Data, making reports, and so forth The getting to step includes the accompanying undertakings:
Managing:
The last advance of the Data mart execution process envelops distinctive administration errands like:
- Organizations face an unending development of data. Getting noteworthy, information driven bits of knowledge becomes hard for those actually utilizing on-premises arrangements. In the Big Data reality, information distribution centers are dynamically moving to the cloud — as are information stores. Cloud arrangements work with putting away and sharing monstrous arrangements of information opening the genuine force of compelling information examination.
- Cloud-based stages offer adaptable structures with isolated information stockpiling and process powers, bringing about better adaptability and quicker information questioning. With a solitary store containing all information marts in the cloud, organizations can bring down costs as well as furnish all divisions with unhindered admittance to information continuously.
- Moreover, cloud information stores can be an extraordinary apparatus for AI purposes. Information marts contain all the pertinent data associated with exchanges, items, or clients for a given timeframe. Since they’re dependable, they can be utilized to fabricate distinctive ML models, for example, affinity models anticipating client beat or those giving customized suggestions.
Conclusion :-