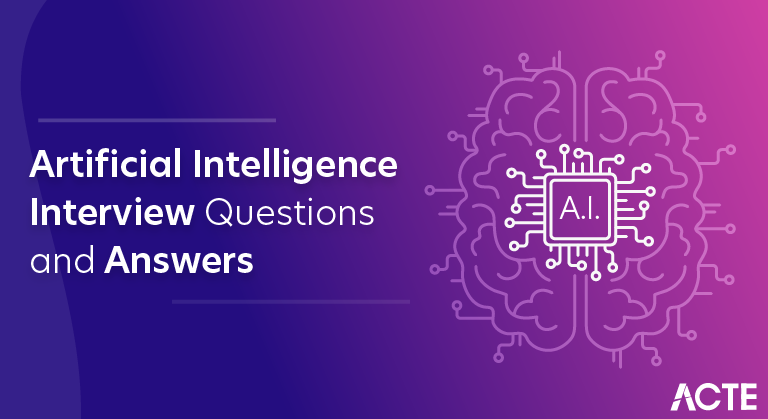
The future in the field of artificial intelligence is undoubtedly bright, regardless of whether you want to pursue a career in it or want to advance from where you now are. Alongside you, there are plenty other professionals who have realized there are prospects to advance in the industry. In light of the competitive nature of this field, present yourself as an exceptional job applicant who stands out from the masses. Therefore, it’s a good idea to not only obtain artificial intelligence certificates but also to get ready for important job interview questions using AI in advance. These frequently asked questions can help you become ready for the same.
1) Explain the concept of reinforcement learning in artificial intelligence.
Ans:
- Reinforcement learning is a branch of AI where an agent learns to make decisions by interacting with an environment.
- The agent receives feedback in the form of rewards or penalties, guiding it to discover optimal strategies.
- Through trial and error, the agent refines its actions to maximize cumulative rewards.
- This iterative process resembles how humans learn from experience, making reinforcement learning crucial for tasks like game playing and robotic control.
2) What distinguishes supervised learning from unsupervised learning?
Ans:
- Supervised learning involves training a model on labeled data, where input-output pairs guide the algorithm to make predictions.
- In contrast, unsupervised learning deals with unlabeled data, requiring the algorithm to uncover patterns or structures independently.
- While supervised learning is suitable for classification and regression, unsupervised learning finds applications in clustering and dimensionality reduction, offering insights into underlying data relationships.
3) Elaborate on the challenges associated with training deep neural networks.
Ans:
- Training deep neural networks poses challenges such as vanishing gradients and overfitting. Vanishing gradients hinder the update of early layers, impeding learning.
- Overfitting occurs when a model excessively fits the training data, compromising its ability to generalize.
- Addressing these challenges involves techniques like weight initialization, batch normalization, and dropout, which collectively enhance network stability and generalization performance.
- The intricate interplay of these factors underscores the complexity of optimizing deep neural networks for diverse tasks.
4) Define the term “bias” in the context of machine learning algorithms.
Ans:
- Bias in machine learning refers to the systematic error introduced when a model consistently makes predictions that deviate from the true values.
- It can result from the model’s oversimplified assumptions or inadequate representation of the underlying data.
- Managing bias is crucial for achieving accurate and fair predictions.
- Techniques like data augmentation, model complexity adjustment, and diverse dataset curation aim to mitigate bias, fostering more equitable and reliable machine learning outcomes.
5) How does transfer learning contribute to the efficiency of deep learning models?
Ans:
- Transfer learning enhances deep learning efficiency by leveraging knowledge acquired from one task to improve performance on a different but related task.
- Pre-trained models, having learned generic features from vast datasets, serve as starting points.
- Fine-tuning on task-specific data enables the model to adapt and specialize.
- This approach reduces the need for extensive labeled data and accelerates model convergence, making transfer learning a valuable strategy for optimizing the performance of deep learning models across various applications.
6) What role does the activation function play in a neural network?
Ans:
- The activation function in a neural network introduces non-linearity, enabling the model to learn complex patterns.
- Common activation functions like ReLU (Rectified Linear Unit) transform input signals, facilitating the network’s ability to approximate intricate relationships within data.
- The non-linear activation functions enable the network to capture and represent diverse features, fostering the depth and expressiveness necessary for effectively modeling real-world data in tasks such as image recognition and natural language processing.
7) Discuss the concept of explainable artificial intelligence (XAI) and its significance.
Ans:
- Explainable Artificial Intelligence (XAI) focuses on developing models that provide interpretable and understandable insights into their decision-making processes.
- This transparency is crucial, especially in critical domains like healthcare and finance.
- XAI methods, such as LIME (Local Interpretable Model-agnostic Explanations) and SHAP (SHapley Additive exPlanations), offer post-hoc explanations for complex models, enabling users to comprehend and trust AI decisions.
- Achieving explainability is pivotal for fostering user trust, regulatory compliance, and ensuring ethical deployment of AI systems.
8) How does the concept of attention mechanism improve the performance of neural networks?
Ans:
- This mechanism is particularly beneficial in tasks where specific parts of input sequences are more informative than others, such as machine translation and image captioning.
- Attention mechanisms enable the model to weigh contextual information dynamically, facilitating more accurate and context-aware predictions, thereby contributing to the improved overall performance of neural networks in various applications.
9) What is the role of recurrent neural networks (RNNs) in sequential data processing?
Ans:
- Recurrent Neural Networks (RNNs) are designed for sequential data processing, allowing them to maintain memory of past inputs.
- This enables RNNs to capture temporal dependencies and relationships in sequences, making them well-suited for tasks like natural language processing and time-series analysis.
- However, RNNs face challenges such as vanishing gradients, limiting their ability to capture long-range dependencies effectively.
- Advanced variants like Long Short-Term Memory (LSTM) networks and Gated Recurrent Units (GRUs) address these issues, enhancing the capability of RNNs in handling sequential information.
10) Discuss the trade-offs between model complexity and interpretability in machine learning.
Ans:
- The trade-off between model complexity and interpretability is a fundamental consideration in machine learning. Complex models, such as deep neural networks, often yield high accuracy but are challenging to interpret.
- Simpler models, like decision trees, are more interpretable but may sacrifice predictive performance.
- Striking the right balance is crucial, as excessively complex models may lead to overfitting and increased computational costs.
- Techniques like feature importance analysis and model-agnostic interpretability methods provide insights, enabling practitioners to navigate this trade-off based on the specific requirements of a given application.
11) Explain the concept of adversarial attacks on machine learning models.
Ans:
- Adversarial attacks involve manipulating input data to mislead machine learning models, causing them to make incorrect predictions.
- These attacks exploit model vulnerabilities, often imperceptible to humans, and can have serious consequences in safety-critical applications.
- Robustness against adversarial attacks is a significant challenge in AI. Techniques like adversarial training and input preprocessing aim to fortify models against such attacks.
- Achieving resilience is crucial, especially in applications like autonomous vehicles and cybersecurity, where adversarial attacks pose potential threats to the reliability and security of machine learning systems.
12) How do autoencoders contribute to unsupervised learning?
Ans:
- Autoencoders are unsupervised learning models designed for dimensionality reduction and feature learning.
- Consisting of an encoder and a decoder, autoencoders learn to encode input data into a compact representation and reconstruct the original data from this representation.
- By forcing the model to capture essential features during reconstruction, autoencoders uncover meaningful representations of input data.
- This unsupervised approach is valuable for tasks like anomaly detection and data denoising, where the model learns to encode salient information while disregarding noise, contributing to improved data representation and analysis.
13) What is overfitting in Machine Learning, and how can it be prevented?
Ans:
- Hyperparameter tuning is the process of systematically adjusting model hyperparameters to enhance performance.
- These parameters, not learned during training, influence model behavior and generalization.
- Techniques like grid search and random search explore hyperparameter combinations to identify optimal configurations.
- Tuning is critical, as the right set of hyperparameters can significantly improve a model’s accuracy and robustness.
- However, it requires careful consideration of computational resources and trade-offs, as exhaustive searches can be computationally expensive.
- Automated approaches, such as Bayesian optimization, streamline this process, efficiently navigating the vast hyperparameter space.
14) What challenges and solutions are associated with handling imbalanced datasets in machine learning?
Ans:
- Imbalanced datasets, where one class significantly outnumbers others, pose challenges in training models that may become biased towards the majority class. This imbalance affects predictive performance, especially for minority classes.
- Addressing this requires techniques like oversampling, undersampling, and the use of appropriate evaluation metrics such as precision-recall curves.
- Advanced approaches, including ensemble methods and synthetic data generation, contribute to mitigating imbalances.
- Striking a balance between class representation and computational efficiency is crucial for developing models that generalize well across diverse and imbalanced datasets.
15) Describe the difference between Classification and Regression.
Ans:
Aspect | Classification | Regression | |
Objective |
Assigns data points to predefined categories or classes. |
Predicts a continuous output or value. | |
Output Type | Discrete labels or categories. | Continuous numeric values. | |
Task Type | Classifying data into distinct groups. | Predicting a trend or quantity. | |
Example |
Predicting email as spam or not (binary classification). |
Predicting house prices or temperature (continuous). |
16) Discuss the importance of feature engineering in machine learning.
Ans:
- Feature engineering involves selecting, transforming, and creating relevant features to enhance a model’s performance.
- Despite advancements in deep learning, thoughtful feature engineering remains crucial, especially when dealing with small datasets or specific domains.
- Well-crafted features provide models with meaningful information, improving their ability to capture complex patterns.
- Techniques such as one-hot encoding, scaling, and polynomial features contribute to effective feature engineering.
- Striking the right balance between automated feature learning and domain expertise-driven feature engineering is pivotal for developing robust machine learning models with enhanced predictive capabilities.
17) What challenges are associated with deploying machine learning models in real-world scenarios?
Ans:
- Deploying machine learning models in real-world scenarios introduces challenges related to scalability, interpretability, and robustness.
- Scaling models to handle large volumes of data and ensuring low-latency responses are critical considerations.
- Interpretability is essential for user trust and regulatory compliance.
- Additionally, models must exhibit robustness against diverse inputs and changing environments. Continuous monitoring and updates are necessary to adapt to evolving data distributions and maintain model performance.
- Balancing these challenges requires a comprehensive approach, combining technical expertise, ethical considerations, and a thorough understanding of the specific application domain.
18) Discuss the concept of ensemble learning and its advantages.
Ans:
- Ensemble learning involves combining predictions from multiple models to improve overall performance.
- Methods like bagging and boosting create diverse models, reducing overfitting and enhancing generalization.
- Ensemble learning is particularly effective when individual models have complementary strengths and weaknesses.
- Random Forests, a popular ensemble method, leverages decision trees to achieve robust predictions.
- The collaborative nature of ensemble learning not only enhances accuracy but also provides increased resilience against noisy data.
- Despite its advantages, ensemble learning requires careful consideration of computational resources and may face challenges in interpretability compared to single models.
19) Explain the role of natural language processing (NLP) in AI applications.
Ans:
- Natural Language Processing (NLP) is a subfield of AI that focuses on enabling machines to understand, interpret, and generate human language.
- NLP plays a crucial role in various applications, including sentiment analysis, machine translation, and chatbots.
- Techniques like tokenization, part-of-speech tagging, and named entity recognition contribute to processing and understanding textual data.
- The challenges in NLP include handling ambiguity and context, but recent advancements, such as transformer models, have significantly improved the performance of NLP systems, enabling them to achieve state-of-the-art results across a wide range of language-related tasks.
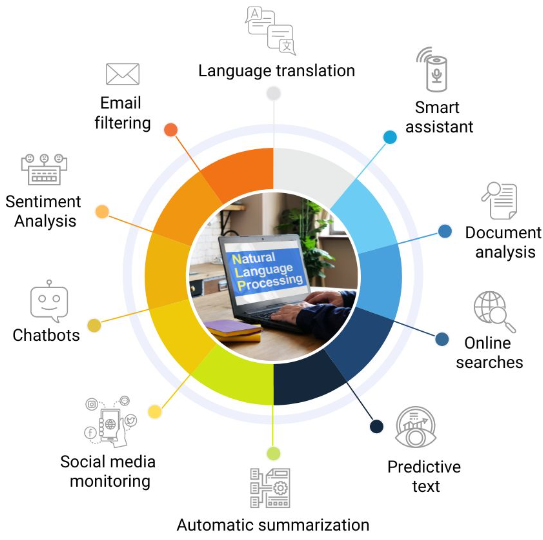
20) Discuss the limitations of current artificial intelligence systems.
Ans:
- Current AI systems face limitations related to interpretability, ethical concerns, and generalization.
- Deep learning models, while powerful, often lack interpretability, making it challenging to understand their decision-making processes.
- Ethical concerns arise from biased training data and potential misuse of AI technologies.
- Generalization, especially in dynamic environments, remains a challenge, as models may struggle to adapt to novel situations.
- Addressing these limitations requires interdisciplinary collaboration, incorporating ethical considerations into AI development, and advancing research in explainable AI and robust learning techniques.
21) What is the role of attention mechanisms in transformer models?
Ans:
- Attention mechanisms in transformer models enable the model to focus on specific parts of the input sequence when making predictions.
- This attention mechanism, introduced in the transformer architecture, allows the model to assign different weights to different positions in the input sequence dynamically.
- This mechanism is crucial for handling long-range dependencies in sequences and capturing contextual information effectively.
- Transformers, with their self-attention mechanism, have demonstrated exceptional performance in various natural language processing tasks, such as language translation and text summarization, showcasing the significance of attention mechanisms in modern AI architectures.
22) Discuss the challenges and advancements in AI for computer vision.
Ans:
- AI for computer vision faces challenges such as object recognition in complex scenes and handling variations in lighting and viewpoint.
- Advancements in convolutional neural networks (CNNs) have significantly improved object recognition, enabling models to learn hierarchical features. Transfer learning and pre-trained models further boost performance on specific computer vision tasks.
- Addressing challenges also involves innovations in semantic segmentation and instance segmentation, allowing models to understand not only objects but also their boundaries and relationships within an image.
- The continuous evolution of deep learning architectures contributes to the ongoing progress and applicability of AI in computer vision.
23) Explain the concept of federated learning and its applications.
Ans:
- Federated learning involves training a machine learning model across decentralized devices without exchanging raw data.
- Instead, models are trained locally on user devices, and only model updates are shared with a central server.
- This approach enhances privacy and reduces the need for data centralization. Federated learning finds applications in scenarios where data privacy is paramount, such as healthcare and edge computing.
- Challenges include communication efficiency and handling non-IID (non-identically distributed) data.
- Despite these challenges, federated learning offers a promising paradigm for collaborative model training while preserving user privacy.
24) How do Bayesian methods contribute to uncertainty quantification in machine learning?
Ans:
- Bayesian methods provide a probabilistic framework for modeling uncertainty in machine learning. Unlike traditional methods that output point estimates, Bayesian models yield probability distributions over parameters and predictions.
- This uncertainty quantification is valuable in decision-making processes, especially in critical domains like healthcare and finance. Bayesian methods, including Bayesian neural networks and Gaussian processes, enable practitioners to capture and propagate uncertainty, improving model robustness and reliability.
- Incorporating uncertainty quantification is essential for developing trustworthy machine learning models that account for variability and potential risks in real-world applications.
25) Discuss the impact of AI in healthcare and the challenges associated with its implementation.
Ans:
- AI in healthcare has transformative potential, aiding in medical diagnosis, drug discovery, and personalized treatment plans.
- Machine learning models analyze vast datasets, assisting clinicians in making informed decisions.
- Challenges include ensuring model interpretability, addressing biases in training data, and navigating regulatory compliance.
- Interdisciplinary collaboration between healthcare professionals and AI experts is crucial to overcome these challenges.
26) Explain the concept of transfer learning in machine learning.
Ans:
- Utilizes knowledge gained from one task to improve performance on another.
- Involves pre-training models on large datasets before fine-tuning on specific tasks.
- Accelerates convergence and reduces the need for extensive task-specific data.
- Enhances model adaptability across diverse applications.
- Enables the leveraging of generic features learned from different but related tasks.
27) What is the significance of regularization techniques in machine learning, and how do they prevent overfitting?
Ans:
- Regularization techniques play a crucial role in preventing overfitting by imposing constraints on the model parameters during training.
- L1 and L2 regularization add penalty terms to the loss function based on the magnitudes of weights, discouraging overly complex models.
- Dropout, another regularization method, randomly deactivated neurons during training, introducing diversity and preventing over-reliance on specific features.
- These techniques promote generalization by discouraging the model from fitting noise in the training data, ultimately enhancing its ability to make accurate predictions on unseen data.
28) Discuss the challenges associated with training deep neural networks.
Ans:
- Training deep neural networks poses challenges such as vanishing gradients and overfitting.
- Vanishing gradients hinder the update of early layers, impeding learning. Overfitting occurs when a model excessively fits the training data, compromising its ability to generalize.
- Addressing these challenges involves techniques like weight initialization, batch normalization, and dropout, which collectively enhance network stability and generalization performance.
- The intricate interplay of these factors underscores the complexity of optimizing deep neural networks for diverse tasks.
29) Explain the role of attention mechanisms in natural language processing.
Ans:
- Attention mechanisms in natural language processing (NLP) allow models to selectively focus on different parts of input sequences when making predictions.
- This dynamic focus is particularly beneficial in tasks like machine translation, where certain words or phrases carry more significance than others.
- Attention mechanisms enhance the model’s ability to capture contextual information effectively, improving performance in understanding and generating human language.
- The introduction of attention mechanisms, especially in transformer-based models, has significantly advanced the state-of-the-art in various NLP applications, showcasing their pivotal role in language understanding.
30) What is the difference between bagging and boosting in ensemble learning?
Ans:
- Bagging (Bootstrap Aggregating) and boosting are ensemble learning techniques that aim to improve model performance by combining multiple base models.
- The key difference lies in their approach to constructing these models. Bagging involves training each base model independently on random subsets of the training data (with replacement) and aggregating their predictions.
- Random Forests, a popular bagging method, utilizes decision trees.
- Boosting, on the other hand, focuses on sequentially training models, with each subsequent model giving more weight to misclassified instances by the previous models.
- Gradient Boosting Machines (GBM) exemplify this boosting approach, demonstrating the nuanced distinctions between bagging and boosting in ensemble learning.
31) What is the purpose of dropout in neural networks?
Ans:
- Mitigates overfitting by randomly deactivating neurons during training.
- Promotes model generalization by preventing reliance on specific features.
- Introduces diversity in the learning process, improving robustness.
- Allows the model to learn more robust and generalized features.
- Enhances training efficiency and reduces the risk of model memorization.
32) What role does activation function play in a neural network?
Ans:
- Introduces non-linearity, enabling the network to learn complex patterns.
- Facilitates the network’s ability to approximate intricate relationships within data.
- Enables the model to capture and represent diverse features.
- Essential for fostering depth and expressiveness in neural network architectures.
- Common activation functions include ReLU, sigmoid, and tanh.
33) What is federated learning, and what are its advantages?
Ans:
- Trains models across decentralized devices without exchanging raw data.
- Enhances privacy by keeping data on local devices.
- Reduces the need for centralized data storage.
- Finds applications in privacy-sensitive domains like healthcare.
- Challenges include communication efficiency and non-IID data handling.
34) Explain the difference between shallow and deep learning.
Ans:
Traditional machine learning models with a modest number of layers (e.g., linear regression, decision trees) are commonly referred to as shallow learning.
Deep learning uses neural networks with several layers to learn sophisticated hierarchical data representations.
35) What distinguishes bagging from boosting in ensemble learning?
Ans:
- Bagging involves training models independently on random subsets of data.
- Reduces overfitting by aggregating predictions from diverse models.
- Popular example: Random Forests, using decision trees.
- Boosting focuses on sequential training models, giving more weight to misclassified instances.
- Gradient Boosting Machines (GBM) exemplify this approach.
36) What are some common applications of natural language processing (NLP)?
Ans:
- Sentiment Analysis
- Chatbots and Virtual Assistants
- Language Translation
- Named Entity Recognition (NER)
- Speech Recognition
- Text Summarization
- Question Answering Systems
- Information Retrieval
Natural Language Processing (NLP) has a wide range of applications across various domains. Here are some common applications:
37) Discuss the role of hyperparameter tuning in machine learning optimization.
Ans:
- Systematic adjustment of hyperparameters enhances model performance.
- Techniques include grid search and random search for optimal configurations.
- Crucial for improving model accuracy and robustness.
- Requires consideration of computational resources and trade-offs.
- Automated approaches like Bayesian optimization streamline the tuning process.
38) What is the significance of ensemble learning, and what are its advantages?
Ans:
- Ensemble learning combines predictions from multiple models.
- Reduces overfitting and enhances generalization performance.
- Effective when individual models have complementary strengths.
- Popular method: Random Forests, leveraging decision trees.
- Challenges include interpretability compared to single models.
39) Explain the impact of AI in healthcare and the challenges associated with its implementation.
Ans:
- AI aids in medical diagnosis, drug discovery, and personalized treatment.
- Challenges include ensuring model interpretability and addressing biases.
- Regulatory compliance and ethical considerations are crucial.
- Interdisciplinary collaboration between healthcare professionals and AI experts is vital.
- Positive impact evident in improved diagnostics, treatment planning, and patient outcomes.
40) How do you assess the performance of a machine learning model, and what evaluation metrics would you use for classification problems?
Ans:
Evaluation metrics are commonly used to evaluate the performance of models. The most used indicators for categorization issues are accuracy, precision, recall, F1 score, and area under the receiver operating characteristic (ROC) curve. The particular objectives of the model and the nature of the issues such as whether false positives or false negatives are more important to determine which metrics are used.
41) What role does natural language processing (NLP) play in AI applications?
Ans:
- NLP focuses on enabling machines to understand, interpret, and generate human language.
- Applications include sentiment analysis, machine translation, and chatbots.
- Techniques like tokenization and part-of-speech tagging contribute to processing textual data.
- Challenges include handling ambiguity and context.
- Transformer models, like BERT, have significantly improved NLP performance.
42) Discuss the impact of Bayesian methods on uncertainty quantification in machine learning.
Ans:
- Bayesian methods provide a probabilistic framework for modeling uncertainty.
- Contrast with traditional methods, Bayesian models yield probability distributions.
- Valuable for decision-making in critical domains like healthcare and finance.
- Bayesian neural networks and Gaussian processes contribute to uncertainty quantification.
- Essential for developing trustworthy machine learning models in real-world applications.
43) Explain the concept of autoencoders and their contribution to unsupervised learning.
Ans:
- Autoencoders are unsupervised learning models for dimensionality reduction and feature learning.
- Comprise an encoder and a decoder to learn compact data representations.
- Enforce the model to encode and reconstruct input data during training.
- Valuable for tasks like anomaly detection and data denoising.
- Unsupervised approach contributes to improved data representation and analysis.
44) Discuss the concept of deep learning interpretability. Why is it important, and how can it be achieved?
Ans:
Deep learning models, especially deep neural networks, are often considered “black boxes” due to their complexity. Achieving interpretability is crucial for understanding model decisions and building trust. Techniques include using interpretable model architectures, feature importance methods, and generating post-hoc explanations using tools like SHAP (SHapley Additive exPlanations).
45) Explain the concept of deep reinforcement learning. In what domains is it commonly applied?
Ans:
Combining deep learning methods with reinforcement learning is called deep reinforcement learning. Learning effective decision-making rules in complex and high-dimensional state spaces is accomplished through training deep neural networks. Autonomous vehicles, robotics, and gaming are among the fields where deep reinforcement learning finds use.
46) Can you explain the concept of bias in machine learning algorithms? How can it be addressed?
Ans:
- Bias in machine learning refers to the presence of systematic errors in the model’s predictions, often due to biased training data.
- This can result in disproportionate or unfair treatment outcomes.
- Addressing bias involves using diverse and representative datasets, employing fairness-aware algorithms, and continuously monitoring and mitigating biases in the model..
47) Mention some disadvantages of vanishing gradient.
Ans:
- Slow Convergence
- Difficulty in Capturing Long-Term Dependencies
- Impaired Weight Updates
- Difficulty in Learning Useful Representations
- Reduced Model Capacity
- Challenges in Training Deep Architectures
- Inefficient Use of Computational Resources
- Dependency on Initialization
- Increased Susceptibility to Local Minima
- Limitations in Representing Hierarchical Features
Some of the disadvantages includes:
48) What ethical considerations should be taken into account when developing AI systems?
Ans:
In the process of developing AI, ethical factors like responsibility, transparency, privacy concerns, bias and fairness, and the possible social effects of AI applications are all taken into account. Prioritizing ethical AI practices, taking into account how AI systems may affect society, and making a concerted effort to reduce prejudice and discrimination are all crucial.
49) What role does the kernel function play in Support Vector Machines (SVMs)?
Ans:
- Defines the similarity measure between data points in the input space.
- Maps input data into a higher-dimensional feature space.
- Enables SVMs to find complex decision boundaries.
- Common kernels include linear, polynomial, and radial basis function (RBF).
- The choice of kernel influences SVM performance on different types of data.
50) Discuss the challenges associated with training recurrent neural networks (RNNs).
Ans:
- Face difficulties in capturing long-range dependencies.
- Vulnerable to vanishing and exploding gradient problems.
- Limited by sequential processing, hindering parallelization.
- Advanced variants like LSTM and GRU address these challenges.
- Choosing suitable architectures and hyperparameters is crucial for RNN training.
51) What is the difference between AI and machine learning?
Ans:
Artificial intelligence (AI) is a broad term that refers to robots or systems that are capable of executing tasks that normally require human intelligence.
Machine Learning (ML) is a subfield of artificial intelligence (AI) that focuses on the development of algorithms that allow computers to learn from data and make predictions or judgments without explicit programming.
52) Explain the concept of Monte Carlo simulation in the context of reinforcement learning.
Ans:
- Utilizes random sampling to estimate numerical results.
- In reinforcement learning, it helps approximate expected rewards.
- Particularly useful in scenarios with complex state spaces.
- Enables agents to make informed decisions based on sampled outcomes.
- Balances exploration and exploitation in the learning process.
53) Discuss the trade-offs between model accuracy and interpretability in machine learning.
Ans:
- Complex models may achieve high accuracy but lack interpretability.
- Simpler models, like decision trees, offer interpretability but may sacrifice accuracy.
- Striking a balance depends on the application requirements.
- Model-agnostic interpretability methods provide insights into complex models.
- Trade-offs involve considering the specific needs of the problem at hand.
54) What are the challenges associated with scaling machine learning models, and how can they be addressed?
Ans:
Issues with distributed computing, computational resources, and model performance maintenance arise when machine learning models are scaled. Using distributed training frameworks, enhancing algorithms for parallel processing, and utilizing cloud computing platforms are some solutions. Scalable architectures must be created for the training and inference stages.
55) Discuss the impact of imbalanced datasets on machine learning models.
Ans:
- Imbalanced datasets have unequal distribution of classes.
- Can lead to biased models favoring the majority class.
- Challenges model evaluation, as accuracy may not reflect true performance.
- Techniques like oversampling and undersampling address imbalance.
- Addressing class imbalance is crucial for fair and accurate model training.
56) What is the role of the learning rate in training machine learning models?
Ans:
- Determines the size of steps taken during optimization.
- Influences the convergence speed of the training process.
- Too high a learning rate may lead to overshooting the optimal solution.
- Too low a learning rate results in slow convergence.
- Adjusting the learning rate is crucial for effective model training.
57) Explain the concept of natural language processing (NLP) and its applications.
Ans:
- NLP focuses on enabling machines to understand, interpret, and generate human language.
- Applications include sentiment analysis, machine translation, and chatbots.
- Tokenization and part-of-speech tagging are fundamental NLP techniques.
- Challenges involve handling ambiguity and context in language.
- Transformer models have significantly advanced the performance of NLP systems.
58) What distinguishes reinforcement learning from supervised learning?
Ans:
- Reinforcement learning involves learning from interactions with an environment.
- Agents take actions to maximize cumulative rewards over time.
- Feedback is in the form of rewards or penalties based on actions.
- Exploration-exploitation trade-off is a fundamental challenge in reinforcement learning.
- Well-suited for dynamic environments where optimal strategies evolve over time.
59) Discuss the challenges associated with deploying machine learning models in production.
Ans:
- Ensuring scalability for handling large volumes of real-time data.
- Model interpretability is crucial for user trust and regulatory compliance.
- Addressing issues related to model drift and changing data distributions.
- Continuous monitoring and updates for adapting to evolving environments.
- Collaborative efforts involving data scientists, engineers, and domain experts are essential.
60) Explain the concept of hierarchical clustering in unsupervised learning.
Ans:
- Groups similar data points into hierarchical clusters.
- Builds a tree-like structure (dendrogram) to represent the clustering process.
- Common linkage methods include single, complete, and average linkage.
- Enables exploration of cluster relationships at different levels.
- Useful in scenarios where hierarchical relationships exist in the data.
61) Discuss the challenges associated with machine learning model interpretability.
Ans:
- Complex models like deep neural networks often lack interpretability.
- Interpretable models like decision trees may sacrifice predictive accuracy.
- Balancing trade-offs between accuracy and interpretability is challenging.
- Model-agnostic interpretability methods provide post-hoc explanations.
- Addressing interpretability is crucial for user trust and regulatory compliance.
62) How do you handle missing data in a dataset when building a machine learning model?
Ans:
Handling missing data is crucial for building robust machine learning models. Strategies include removing instances with missing values, imputing missing values using statistical measures (mean, median, or mode), or using advanced techniques like data imputation algorithms. The choice depends on the nature of the data and the specific task.
63) Explain the concept of Bayesian methods in machine learning.
Ans:
- Bayesian methods provide a probabilistic framework for modeling uncertainty.
- Contrast with traditional methods, Bayesian models yield probability distributions.
- Valuable for decision-making in critical domains like healthcare and finance.
- Bayesian neural networks and Gaussian processes contribute to uncertainty quantification.
- Essential for developing trustworthy machine learning models in real-world applications.
64) What distinguishes ensemble learning from individual models in machine learning?
Ans:
- Ensemble learning combines predictions from multiple models.
- Reduces overfitting and enhances generalization performance.
- Effective when individual models have complementary strengths.
- Popular method: Random Forests, leveraging decision trees.
- Challenges include interpretability compared to single models.
65) What distinguishes deep learning from traditional machine learning approaches?
Ans:
- Deep learning differs from traditional machine learning approaches primarily in its use of deep neural networks, characterized by multiple layers of interconnected nodes.
- While traditional machine learning models may involve handcrafted feature engineering, deep learning models learn hierarchical features directly from data.
- Deep learning excels in tasks requiring complex pattern recognition, such as image and speech recognition.
- However, it often demands substantial computational resources and large datasets.
- The depth and representational capacity of deep neural networks contribute to their success in capturing intricate relationships within data, setting them apart from shallower machine learning models.
66) What is the role of reinforcement learning in training autonomous agents, such as self-driving cars or robotic systems?
Ans:
Learning optimal decision-making rules with interacting with the environment is an essential aspect of reinforcement learning in the training of autonomous agents. Reinforcement learning is used to help agents in robotics and self-driving automobiles navigate complex surroundings, make decisions, and adjust to changing circumstances.
67) What is the role of the activation function in a neural network?
Ans:
- The activation function in a neural network introduces non-linearity, enabling the model to learn complex patterns.
- Common activation functions like ReLU (Rectified Linear Unit) transform input signals, facilitating the network’s ability to approximate intricate relationships within data.
- The non-linear activation functions enable the network to capture and represent diverse features, fostering the depth and expressiveness necessary for effectively modeling real-world data in tasks such as image recognition and natural language processing.
68) Discuss the challenges associated with deploying machine learning models in real-world scenarios.
Ans:
- Deploying machine learning models in real-world scenarios introduces challenges related to scalability, interpretability, and robustness.
- Scaling models to handle large volumes of data and ensuring low-latency responses are critical considerations.
- Interpretability is essential for user trust and regulatory compliance.
- Additionally, models must exhibit robustness against diverse inputs and changing environments.
- Continuous monitoring and updates are necessary to adapt to evolving data distributions and maintain model performance.
- Balancing these challenges requires a comprehensive approach, combining technical expertise, ethical considerations, and a thorough understanding of the specific application domain.
69) How does reinforcement learning work?
Ans:
- Reinforcement learning (RL) involves an agent interacting with an environment and making decisions (actions) based on its current state.
- The agent receives feedback in the form of rewards or penalties, aiming to learn a strategy (policy) that maximizes cumulative rewards over time. Key points include the state representing the environment, actions chosen by the agent, immediate rewards, and the exploration-exploitation trade-off.
- RL algorithms, such as Q-learning, use value functions to estimate rewards and techniques like temporal difference learning for iterative updates.
- RL finds applications in game playing, robotics, finance, and other domains requiring adaptive decision-making in dynamic environments.
70) What is deep learning, and how does it differ from traditional machine learning?
Ans:
- Feature Extraction: Deep learning automates feature extraction, eliminating manual engineering compared to traditional machine learning.
- Representation Hierarchy: Deep learning creates hierarchies of features through multiple layers, capturing complex patterns, while traditional machine learning may struggle with intricacies.
- Scale and Data: Deep learning efficiently handles large datasets for generalization, contrasting with traditional machine learning’s scalability challenges.
- Computation Power: Deep learning demands significant computational power due to complex architectures during training, while traditional machine learning models may be less computationally intensive.
- Interpretability: Deep learning models are often considered “black boxes,” lacking interpretability, while traditional machine learning models offer more transparency.
Deep learning, a subset of machine learning, uses neural networks with multiple layers for automated feature extraction, excelling in tasks like image and speech recognition.
Key Differences:In summary, deep learning automates feature extraction, excelling in complex, unstructured data tasks, but may lack interpretability. The choice depends on the task, available data, and computational resources.
71) Explain the concept of overfitting.
Ans:
Overfitting occurs when a machine learning model learns the training data too well, capturing noise and irrelevant details. It results in poor generalization performance on fresh, previously unknown data. Common signs include excessively high accuracy on the training set but low accuracy on validation or test sets. To mitigate overfitting, techniques like regularization and cross-validation are employed.
72) What are some common algorithms used in machine learning?
Ans:
- Linear Regression
- Decision Trees
- Random Forest
- Support Vector Machines (SVM)
- Neural Networks
- Gradient Boosting
- Principal Component Analysis (PCA)
- XGBoost
In machine learning, several common algorithms cater to different tasks. Some of them are as follows:
73) What is natural language processing (NLP)?
Ans:
- In the fascinating realm of Natural Language Processing (NLP), researchers delve into the intricate dance between computers and the richness of human language.
- This captivating field revolves around crafting algorithms and models that empower machines to grasp, interpret, and even emulate human-like language.
- NLP’s real-world magic unfolds in applications like speech recognition, language translation, sentiment analysis, and chatbots, serving as a cornerstone technology for elevating the dialogue between humans and computers.
74) Explain the term “feature engineering”.
Ans:
In the intricate world of machine learning, feature engineering is like crafting a bespoke language for models. It’s the art of carefully choosing, transforming, or inventing key features within a dataset, unlocking the potential for models to better understand and predict patterns. Mastering this process is akin to providing a tailored toolkit, significantly influencing the success of the entire learning journey.
75) What is a Convolutional Neural Network (CNN), and when is it used?
Ans:
- A Convolutional Neural Network (CNN) stands out as a tailored neural architecture crafted for deciphering grid-like information found in images and videos.
- By leveraging convolutional layers, it autonomously absorbs intricate hierarchical representations from input data.
- This specialized design proves highly effective in domains like image recognition, object detection, and computer vision, showcasing superior performance due to its knack for capturing spatial hierarchies and local patterns—a distinguishing feature compared to conventional neural networks.
76) Explain the concept of generative adversarial networks (GANs) and their applications.
Ans:
- Generative Adversarial Networks (GANs) consist of a generator and a discriminator engaged in a competitive learning process.
- The generator aims to create realistic data, while the discriminator differentiates between real and generated samples.
- This adversarial training leads to the generation of high-quality synthetic data, applicable in diverse fields like image synthesis, style transfer, and data augmentation.
- GANs have transformative potential in generating realistic content, though ethical considerations, such as deepfake creation, highlight the need for responsible development and deployment of these powerful generative models.
77) What is the Turing test, and does it determine true artificial intelligence?
Ans:
The Turing test, proposed by Alan Turing, assesses a machine’s ability to engage in human-like conversations. While passing the test signifies conversational competence, it doesn’t determine true artificial general intelligence (AGI), which encompasses broader cognitive functions like reasoning and problem-solving across diverse domains. The Turing test focuses on language skills rather than providing a comprehensive evaluation of AGI.
78) Explain the concept of a Support Vector Machine (SVM) and its applications.
Ans:
- A Support Vector Machine (SVM) is a supervised learning algorithm used for classification and regression tasks.
- It works by finding the hyperplane that best separates data points into different classes.
- SVMs are commonly used in image classification, text classification, and bioinformatics due to their effectiveness in handling high-dimensional data.
79) Explain the concept of a Markov Decision Process (MDP) in reinforcement learning.
Ans:
A Markov Decision Process (MDP) in reinforcement learning is a framework modeling decision-making in sequential and uncertain environments. It involves states, actions, transition probabilities, and rewards, aiming to find an optimal policy for an agent to maximize cumulative rewards over time. MDPs provide a structured approach to tackle problems where decisions impact future outcomes.
80) What is the vanishing gradient problem, and how can it be addressed?
Ans:
The vanishing gradient problem occurs in deep neural networks when gradients become extremely small during backpropagation, hindering weight updates. Techniques like using activation functions with non-vanishing gradients (e.g., ReLU) and employing batch normalization can address this issue.
81) How do you stay updated with the latest developments and trends in artificial intelligence?
Ans:
Staying updated in AI involves regularly reading research papers, following conferences, participating in online communities, and engaging with relevant blogs and forums. Continuous learning through online courses and hands-on projects also helps in staying abreast of the latest advancements in AI.
82) How does a decision tree work?
Ans:
- Think of a decision tree as a decision-making guide shaped like a tree. At every junction (internal node), a decision is made based on a specific feature, leading to different outcomes along branches.
- Ultimately, at the end of each branch, we reach a leaf node, which holds a class label or a value.
- It’s akin to navigating through a series of questions, guiding us to the best conclusion based on the features and their associated outcomes.
83) Can you explain the concept of fine-tuning in transfer learning, and when is it appropriate to use it?
Ans:
- In transfer learning, fine-tuning is taking a pre-trained model and modifying it for a new, particular purpose.
- To do this, the model is further trained using a smaller dataset that is associated with the intended job.
- When the pre-trained model has acquired general characteristics that are helpful for the new task, fine-tuning is acceptable and saves time and resources in comparison to starting from scratch during training.
84) What is the role of data preprocessing in machine learning, and can you provide examples of common preprocessing techniques?
Ans:
A critical phase in machine learning is data preprocessing, which converts unprocessed data into a format that can be used to train models. Typical methods encompass managing absent values, adjusting features, encoding category variables, and standardizing or normalizing numerical attributes. Effective learning of patterns from the data by models is ensured by proper preprocessing of the data.
85) Discuss the concept of model ensembles and how they can improve predictive performance.
Ans:
- In order to improve overall performance, model ensembles combine predictions from various models.
- By using a variety of models, ensembles decrease overfitting and improve generalization.
- Boosting (AdaBoost, Gradient Boosting) and bagging (Random Forests, for example) are two methods.
- The prediction robustness and accuracy can be increased with the use of ensembles.
86) How can you address the curse of dimensionality in machine learning, especially when dealing with high-dimensional data?
Ans:
The difficulties associated with handling high-dimensional data, such as sparsity and higher processing complexity, are referred to as the “curse of dimensionality.” This problem can be solved by employing feature selection, dimensionality reduction techniques (like PCA), and high-dimensional data-specific algorithms like LASSO regression.
87) Explain the concept of cross-validation. Why is it important, and how does it help in model evaluation?
Ans:
- Using a method called cross-validation, several subsets of the dataset are utilized to evaluate a model’s performance.
- Remaining data is used for testing after the model has been trained on a subset of it.
- Averaging performance measures is done after this process is conducted several times.
- A resilient model’s performance that is insensitive to the particular way the data is partitioned is ensured through the use of cross-validation.
88) What are some potential ethical concerns associated with the use of AI, and how would you address them in the development of AI systems?
Ans:
Biases in training data, a lack of transparency, job displacement, and the misuse of AI technology are some ethical concerns with AI. Implementing impartial and equitable algorithms, encouraging openness in model decision-making, and actively participating in moral debates during the development phase are all necessary to allay these worries.
89) Explain the concept of semi-supervised learning and provide examples of scenarios where it is applicable.
Ans:
Training models using both labeled and unlabeled data is known as semi-supervised learning. When getting tagged data is costly or time-consuming, this method can be used. Examples include training a model with a big amount of unlabeled data and a limited amount of labeled data, with the intention of maximizing the performance of the model by utilizing the unlabeled data.
90) Mention some of the advantages and disadvantages of a decision tree in machine learning.
Ans:
- Interpretability
- No Assumptions
- Handles Non-Linearity
- Automatic Feature Selection
- Robust to Outliers
- Minimal Data Preprocessing
- Overfitting
- Instability
- Limited Expressiveness
- Biased to Dominant Classes
- Global Optimization
- Sensitive to Small Variations
Advantages of Decision Trees:
Disadvantages of Decision Trees: