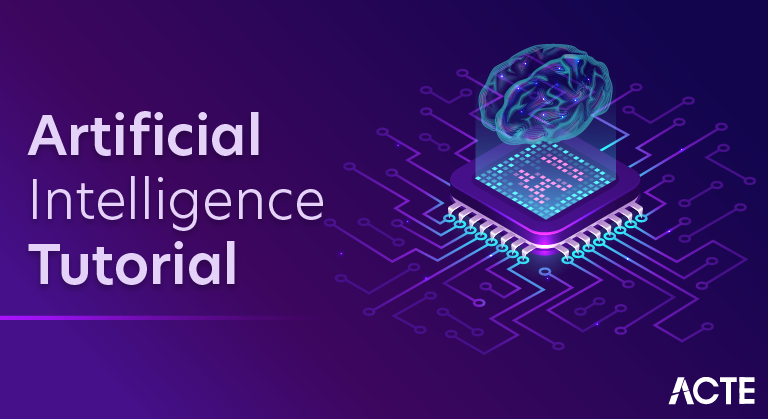
Artificial Intelligence Tutorial – Learn AI from Experts
Last updated on 04th May 2020, Blog, Tutorials
What is AI?
The simulation of human intelligence by computer programs is known as artificial intelligence (AI), which enables computers to reason, acquire knowledge, and carry out jobs that ordinarily demand human intelligence. Artificial intelligence (AI) seeks to develop systems that can imitate human cognitive abilities including problem-solving, reasoning, learning, perception, and language comprehension.
Levels of Artificial Intelligence: From Narrow to Superintelligence
- Narrow AI (Weak AI): Narrow AI, sometimes referred to as weak AI, describes AI systems that are created and instructed for a limited set of activities. These AI systems excel in performing predefined functions but lack the ability to generalize beyond their specific domain. Examples of narrow AI include virtual assistants like Siri and Alexa, image recognition systems, and language translation software.
- Artificial General Intelligence (AGI): AGI, or artificial general intelligence, is a type of artificial intelligence that approaches human general intelligence. AGI systems have the ability to understand, learn, and apply knowledge across diverse domains, similar to human cognition. Unlike narrow AI, AGI can perform any intellectual task that a human can. Achieving AGI is a significant challenge in AI research and remains a long-term goal.
- Artificial Superintelligence: Artificial Superintelligence is a theoretical concept that goes beyond human intelligence in all aspects. It refers to an AI system with capabilities far exceeding human cognitive abilities, including problem-solving, creativity, and social intelligence. Artificial Superintelligence, if realized, could potentially surpass human intelligence in almost every domain.
Large-scale data analysis, pattern recognition, and decision-making or prediction-making are all capabilities of artificial intelligence (AI) systems. Machine learning is a method used by these systems to learn from fresh data in order to adapt and enhance their performance over time. Building methods and models that allow computers to learn from experience without being explicitly programmed is the focus of the subfield of AI known as machine learning.
AI in Today’s Job Market: Navigating Opportunities and Challenges
Increasing Demand for AI Specialists:
As AI becomes more prevalent, there is a rising demand for AI specialists and professionals with expertise in machine learning, data science, natural language processing, and computer vision. Job seekers with advanced technical skills in AI and the ability to develop and deploy AI models are highly sought after by companies looking to use AI as a tool for innovation and business advancement.
AI-Driven Automation and Job Displacement:
The widespread adoption of AI-powered automation is streamlining processes and improving efficiency in various industries. While this automation brings benefits, it also raises concerns about job displacement. Artificial intelligence (AI) may replace or complement many traditional work functions, requiring reskilling and upskilling to keep up with the changing nature of the labor market.
Emerging AI-Related Job Roles:
AI’s impact is not limited to technical positions. The integration of AI in various industries has led to the emergence of new job roles such as AI ethics specialists, AI trainers, AI auditors, and AI project managers. These roles focus on ensuring responsible AI deployment, addressing bias, and overseeing AI projects’ ethical implications.
Cross-Disciplinary Skills in Demand:
The demand for AI expertise is not confined to the tech sector. AI is being utilized in marketing, finance, healthcare, education, and many other fields. As a result, professionals with cross-disciplinary skills, combining domain knowledge with AI proficiency, are becoming increasingly valuable in the job market.
AI and Enhanced Customer Experience:
AI-powered chatbots, virtual assistants, and recommendation systems are transforming customer service and personalization. Job opportunities are expanding in customer experience management, where AI specialists work to implement intelligent systems that enhance customer interactions and improve overall satisfaction.
Artificial intelligence subtype
Artificial intelligence can be divided into three subfields:
- Artificial intelligence
- Machine learning
- Deep learning
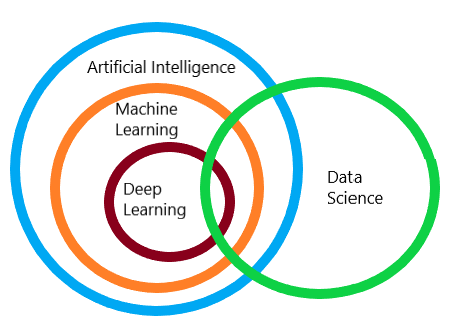
Machine Learning
The development of statistical models and techniques that enable computers to learn from data without being manually programmed is a component of the machine learning (ML) area of artificial intelligence (AI). Allowing computers to automatically learn and enhance their performance on a particular activity or problem based on experience with data is the main goal of machine learning.
Machine learning algorithms utilize data to generalize patterns, make predictions, or uncover hidden insights rather than being explicitly programmed with a set of rules or instructions. In order to provide more accurate and dependable results, the algorithm learns and modifies its internal parameters in response to the incoming data.
The following are some crucial elements of machine learning:
Data: To train machine learning models, you need data that is of high quality, relevance, and representativeness. The model learns patterns and generalises to new circumstances more effectively the more data it is exposed to.
Features: The specific traits or aspects of the data that the machine learning model utilises to generate judgements or predictions are referred to as features. The model’s performance is greatly influenced by the choice and engineering of pertinent features.
Model: The machine learning algorithm’s foundation is the model. It is a mathematical representation or function that connects predictions made based on input data (features).
Testing and Evaluation: After the model has been trained, it is put to the test on a different dataset, referred to as a test dataset, in order to gauge how well it performs and how well it generalizes. To determine the model’s efficacy, its accuracy, and other criteria are evaluated.
Prediction or Inference: Following effective training and assessment, the model may be used to generate predictions or inferences on brand-new, untainted data, offering answers to issues encountered in the actual world.
Deep learning
Deep learning is a branch of machine learning that focuses on modeling and interpreting complicated patterns and representations in data by using artificial neural networks with numerous layers (deep neural networks). The word “deep” refers to how many hidden layers there are between the input and output layers in a neural network. Each hidden layer processes the data and extracts higher-level features, allowing the network to learn hierarchical representations of the data.
Deep Learning’s crucial characteristics and elements include:
Neural Networks: Artificial neural networks used in deep learning are modeled after the structure and functioning of organic neurons in the human brain. These networks consist of interconnected nodes (neurons) organized into layers.
Representation Learning: Deep learning excels at automatically learning feature representations from raw data. Deep neural networks are able to learn valuable and discriminative features from data, reducing the need for human feature engineering and resulting in better performance across a range of applications.
Training with Large Datasets: Deep learning thrives when trained with large-scale datasets. The development of deep learning models has been greatly assisted by the accessibility of huge amounts of labeled data.
Backpropagation: Deep neural networks are trained using an optimization technique called backpropagation. During training, the network makes predictions, and the errors are propagated backward through the layers to update the model’s parameters, reducing the prediction errors iteratively.
Applications: Deep learning has achieved impressive results in various AI applications, including image recognition, natural language processing, language translation, recommendation systems, and game-playing.
AI VS ML
Although AI and machine learning are related concepts, they belong to different domains. The goal of AI is to build intelligent machines that can solve problems, make decisions, and comprehend natural language. Typically, these activities need human intellect. It uses a variety of methods, techniques, and algorithms to imitate the cognitive processes of humans. Machine learning, on the other hand, is a subfield of artificial intelligence that develops models and techniques that enable computers to learn from data and improve their performance on certain tasks without the need for explicit programming. ML approaches enable computers to recognise patterns, make predictions, and modify their behaviour depending on data inputs in tasks where rules-based programming may be expensive or difficult.
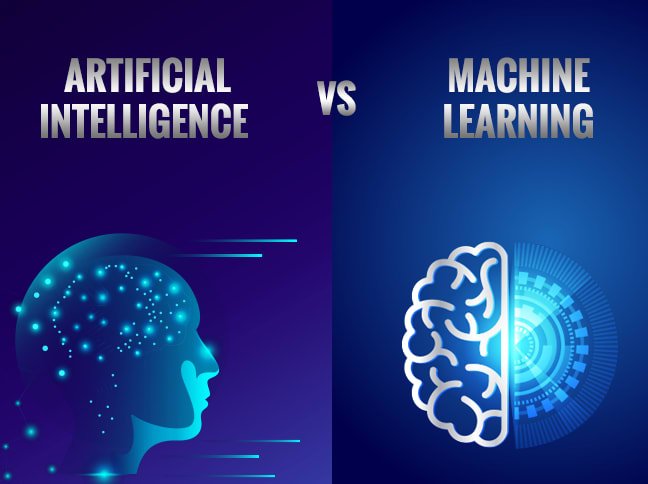
AI Applications: Exploring the Diverse Uses of Artificial Intelligence
AI, or Artificial Intelligence, has found its way into numerous aspects of our lives, revolutionizing how we interact with technology and shaping various industries. From the moment we use virtual assistants like Siri or Alexa to perform tasks or answer questions to the advanced algorithms powering personalized product recommendations when we shop online, AI plays an essential role.
In our daily conversations, AI-driven Natural Language Processing (NLP) enables virtual assistants to understand and respond to us, making interactions more seamless and intuitive. When we post pictures on social media, AI-driven image analysis helps us tag friends and identify objects or locations in the photos. Moreover, AI applications in healthcare have the potential to improve patient care, from medical imaging diagnostics to personalized treatment plans.
AI’s impact is evident in the financial sector, where AI-powered algorithms detect fraud and analyze financial data for smart investment decisions. In manufacturing, AI optimizes production processes and predicts equipment maintenance needs, enhancing efficiency and reducing downtime. Meanwhile, AI-driven customer service chatbots provide instant support, making interactions with companies more efficient and satisfying.
Beyond these uses, AI is advancing the field of autonomous vehicles, allowing these vehicles to negotiate traffic and make deft judgments. AI-driven adaptive learning systems in the classroom provide specialized coaching based on each student’s needs.
In agriculture, AI assists in monitoring soil health and optimizing crop yield, contributing to sustainable farming practices. Moreover, AI applications play a role in environmental monitoring, helping analyze satellite data for climate change research.
As AI continues to evolve and advance, its potential is virtually limitless. From education to healthcare, finance, manufacturing, and beyond, AI is transforming how we live and work. While there are still ethical considerations and challenges to address, the positive impact of AI on various industries is undeniable, and its widespread adoption is set to shape the future of technology and society.
Three Fundamental Components of Artificial Intelligence
Hardware: The term “hardware” describes the actual components and devices used to carry out computations and run AI algorithms. As AI and machine learning models often require immense computational power and parallel processing capabilities, specialized hardware architectures have been developed to optimize AI workloads. Hardware such as Tensor Processing Units (TPUs) and Graphics Processing Units (GPUs) is used to efficiently develop deep learning models and speed up AI computations. The fast expansion and widespread acceptance of AI applications have been greatly helped by improvements in hardware technology.
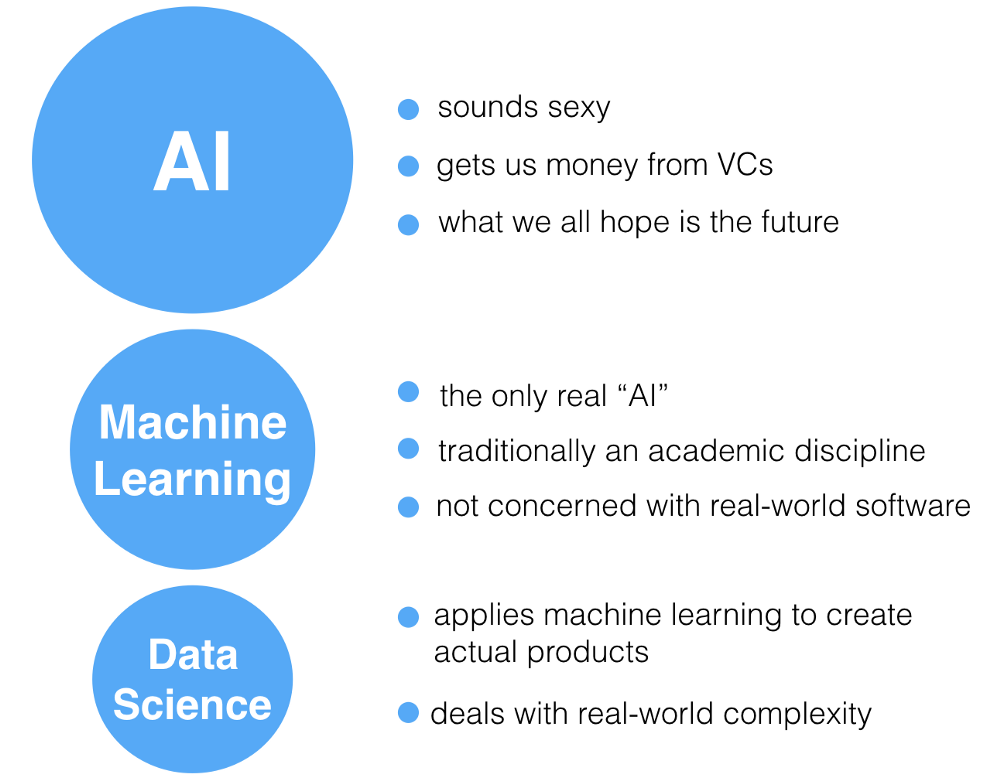
Data: Data is the fuel that drives AI systems. High-quality, diverse, and relevant data is essential for training AI models and ensuring their accuracy and effectiveness. Training an AI algorithm involves feeding it with large amounts of labeled data, allowing the algorithm to learn patterns and make predictions. The more data an AI system can learn from, the better its performance is likely to be. AI development and application have been made easier by the collection, storage, and analysis of enormous amounts of data made possible by big data technology and storage solutions. Data privacy, security, and bias are important considerations in AI, as the quality and integrity of the data directly impact the AI system’s performance and ethical implications.
Algorithms: Algorithms are the mathematical procedures and instructions that AI systems use to analyze data, learn patterns, and make predictions or decisions. Machine learning algorithms, including supervised learning, unsupervised learning, and reinforcement learning, are the core of AI models. Convolutional neural networks (CNNs) and recurrent neural networks (RNNs), two examples of deep learning algorithms, have shown exceptional performance in various tasks like image recognition and natural language processing. Innovations and research in AI algorithms continue to drive the advancement of AI capabilities and expand its potential applications.
What is Intelligence Composed of?
Intelligence is intangible. It is composed of:
- Reasoning
- Learning
- Problem Solving
- Perception
- Linguistic Intelligence
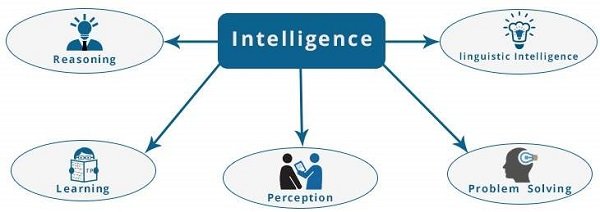
Let us go through all the components briefly
- Reasoning − It is the set of processes that enables us to provide the basis for judgment, making decisions, and prediction.
- Learning − It is the activity of gaining knowledge or skill by studying, practicing, being taught, or experiencing something. Learning enhances the awareness of the subjects of the study. The ability of learning is possessed by humans, some animals, and AI-enabled systems. Learning is categorized as
- Auditory Learning − It is learning by listening and hearing. For example, students listening to recorded audio lectures
- Episodic Learning − To learn by remembering sequences of events that one has witnessed or experienced. This is linear and orderly.
- Motor Learning − It is learning by precise movement of muscles. For example, picking objects, Writing, etc.
- Observational Learning − To learn by watching and imitating others. For example, child tries to learn by mimicking her parent.
- Perceptual Learning − It is learning to recognize stimuli that one has seen before. For example, identifying and classifying objects and situations.
- Relational Learning − It involves learning to differentiate among various stimuli on the basis of relational properties, rather than absolute properties. For Example, Adding ‘little less’ salt at the time of cooking potatoes that came up salty last time, when cooked with adding say a tablespoon of salt.
- Spatial Learning − It is learning through visual stimuli such as images, colors, maps, etc. For Example, A person can create a roadmap in mind before actually following the road.
- Stimulus-Response Learning − It is learning to perform a particular behavior when a certain stimulus is present. For example, a dog raises its ear on the hearing doorbell.
- Problem Solving − It is the process in which one perceives and tries to arrive at a desired solution from a present situation by taking some path, which is blocked by known or unknown hurdles.
Problem solving also includes decision making, which is the process of selecting the best suitable alternative out of multiple alternatives to reach the desired goal. - Perception − It is the process of acquiring, interpreting, selecting, and organizing sensory information.
Perception presumes sensing. In humans, perception is aided by sensory organs. In the domain of AI, perception mechanism puts the data acquired by the sensors together in a meaningful manner. - Linguistic Intelligence − It is one’s ability to use, comprehend, speak, and write the verbal and written language. It is important in interpersonal communication.
Artificial Intelligence – Research Areas
The domain of artificial intelligence is huge in breadth and width. While proceeding, we consider the broadly common and prospering research areas in the domain of AI
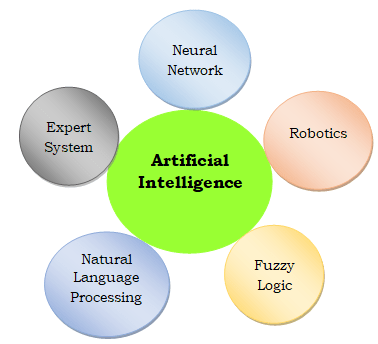
Speech and Voice Recognition
These both terms are common in robotics, expert systems and natural language processing. Though these terms are used interchangeably, their objectives are different.
Speech Recognition | Voice Recognition |
---|---|
The speech recognition aims at understanding and comprehending WHAT was spoken. | The objective of voice recognition is to recognize WHO is speaking. |
It is used in hand-free computing, map, or menu navigation. | It is used to identify a person by analysing its tone, voice pitch, and accent, etc. |
Machine does not need training for Speech Recognition as it is not speaker dependent. | This recognition system needs training as it is person oriented. |
Speaker independent Speech Recognition systems are difficult to develop. | Speaker dependent Speech Recognition systems are comparatively easy to develop. |
Working of Speech and Voice Recognition Systems
The user input spoken at a microphone goes to the sound card of the system. The converter turns the analog signal into an equivalent digital signal for the speech processing. The database is used to compare the sound patterns to recognize the words. Finally, a reverse feedback is given to the database.
This source-language text becomes input to the Translation Engine, which converts it to the target language text. They are supported with interactive GUI, large database of vocabulary, etc.
Real Life Applications of Research Areas
There is a large array of applications where AI is serving common people in their day-to-day lives
The domain of AI is classified into Formal tasks, Mundane tasks, and Expert tasks.
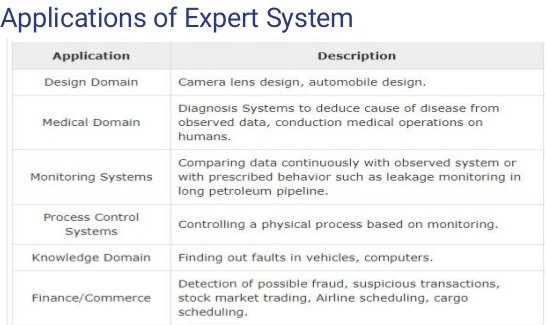
- Expert Systems:Examples − Flight-tracking systems, Clinical systems.
- Natural Language Processing : Examples: Google Now feature, speech recognition, Automatic voice output.
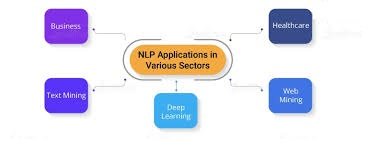
3.Neural Networks :Examples − Pattern recognition systems such as face recognition, character recognition, handwriting recognition.
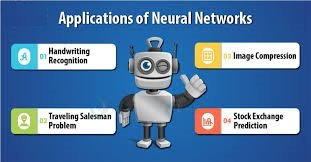
4.Robotics: Examples − Industrial robots for moving, spraying, painting, precision checking, drilling, cleaning, coating, carving, etc.
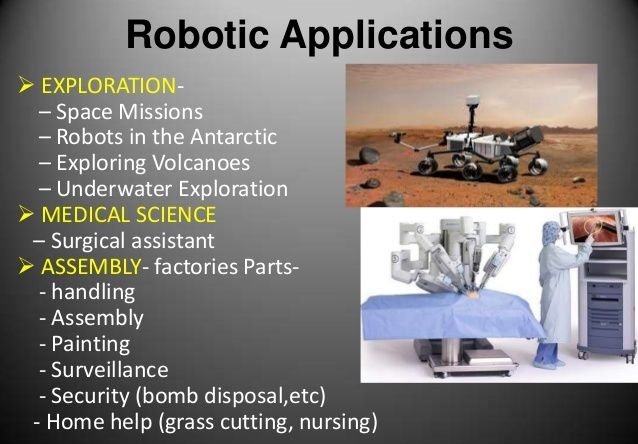
Task Classification of AI
The domain of AI is classified into Formal tasks, Mundane tasks, and Expert tasks.
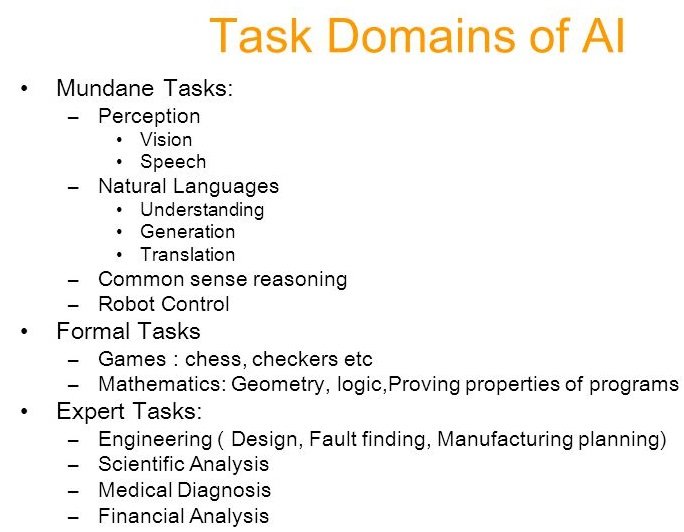
Mundane(Ordinary)Tasks | Formal Tasks | Expert Tasks |
---|---|---|
Perception,Computer Vision,Speech,Voice | Mathematics,Geometry,Logic,Integration and Differentiation | Engineering Fault Finding,Manufacturing,Monitoring |
Natural Language Processing,Understanding Language Generation,Language Translation | Games,Go,Chess(Deep Blue),Checkers | Scientific Analysis |
Common Sense | Verification | Financial Analysis |
Reasoning | Theorem Proving | Medical Diagnosis |
Planning | — | Creativity |
Humans have learned mundane (ordinary) tasks since their birth. They learn by perception, speaking, using language, and locomotives. They learn Formal Tasks and Expert Tasks later, in that order.For humans, the mundane tasks are easiest to learn. The same was considered true before trying to implement mundane tasks in machines.
Earlier, all work of AI was concentrated in the mundane task domain.Later, it turned out that the machine requires more knowledge, complex knowledge representation, and complicated algorithms for handling mundane tasks. This is the reason why AI work is more prospering in the Expert Tasks domain now, as the expert task domain needs expert knowledge without common sense, which can be easier to represent and handle.