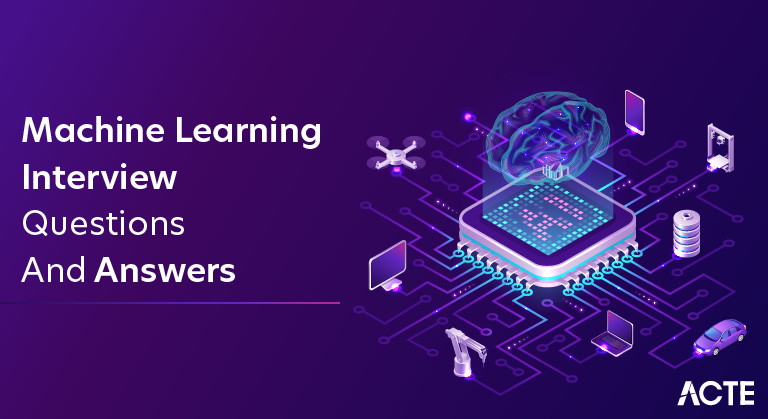
Machine learning is the study of computer algorithms that improve automatically through experience. It is seen as a subset of artificial intelligence.Machine learning focuses on the development of computer programs that can access data and use it learn for themselves
1. What is machine learning?
Ans:
Machine learning is the field of artificial intelligence where computers learn from a data and improve their performance over time without being explicitly programmed. In a traditional programming, rules and instructions are can explicitly defined by humans.
2. What are various types of machine learning algorithms?
Ans:
There are the three main types: supervised learning, unsupervised learning, and reinforcement learning. Supervised learning involves the labeled data for a training, unsupervised learning works with the unlabeled data, and reinforcement learning is based on the reward-based systems.
3. Explain overfitting in machine learning?
Ans:
Overfitting occurs when the model learns the training data too well, including the noise, and performs poorly on new, unseen data. To prevent it, techniques like a cross-validation, regularization, and using a more data can be employed.
4. What is bias-variance trade-off in machine learning?
Ans:
The bias-variance trade-off refers to balance between the model’s ability to fit training data well (low bias) and its ability to generalize to the new, unseen data (low variance). Finding a right balance is essential for a model performance.
5. What is cross-validation in machine learning?
Ans:
Cross-validation is used to assess the model’s performance and generalization ability. It involves the splitting the data into the multiple subsets, training on some, and testing on the others to evaluate how well model performs on unseen data.
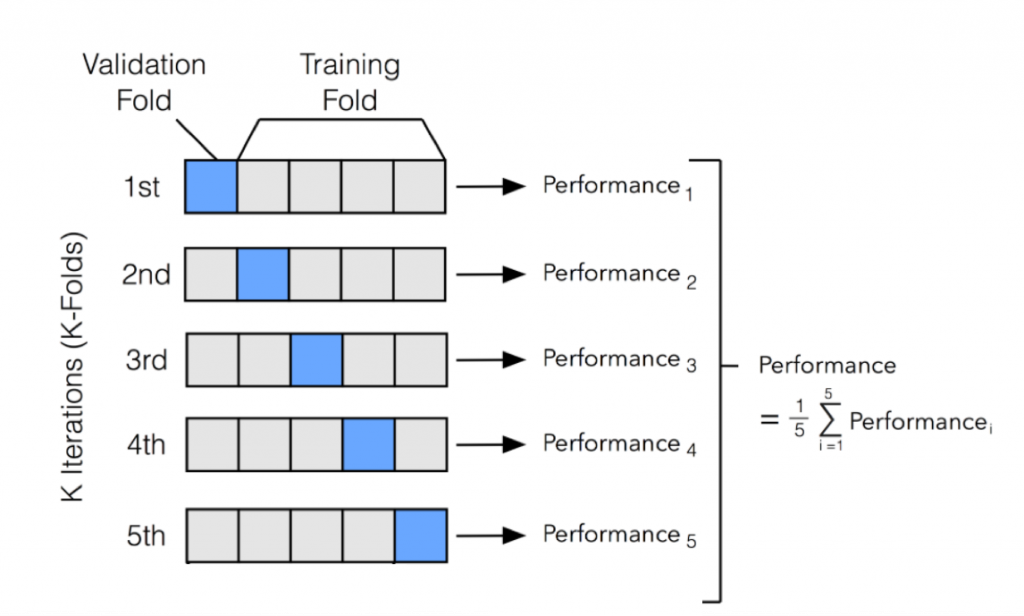
6. What is feature engineering?
Ans:
Feature engineering involves the selecting and transforming the right features (input variables) for the machine learning model. It’s important because well-engineered features can significantly impact the model’s performance.
7. What is confusion matrix in classification tasks?
Ans:
A confusion matrix is the table used to evaluate performance of a classification model. It shows a true positive, true negative, false positive, and false negative predictions, which are used to be alculate metrics like the accuracy, precision, recall, and F1-score.
8. What is gradient descent in context of training machine learning models?
Ans:
Gradient descent is the optimization algorithm used to minimize the error (loss) of machine learning model during training. It iteratively adjusts the model’s parameters in the direction of steepest descent of a loss function.
9. Explain ensemble learning?
Ans:
Ensemble learning combines predictions of the multiple machine learning models to improve the overall performance. Common techniques are include the bagging (e.g., Random Forest) and boosting (e.g., AdaBoost).
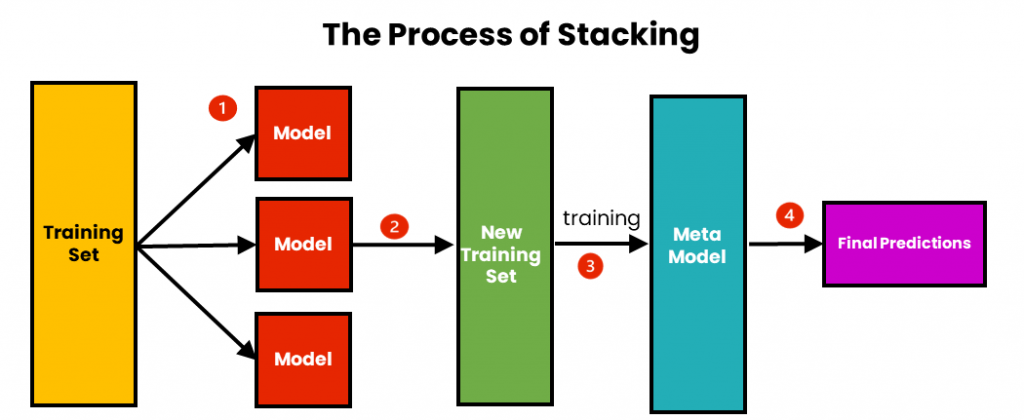
10. What is deep learning?
Ans:
Deep learning is the subset of machine learning that focuses on a neural networks with many layers (deep neural networks). It is particularly effective in the handling complex tasks like an image and speech recognition. Traditional machine learning often the involves simpler models with the fewer layers.
11. What is loss function in machine learning?
Ans:
A loss function quantifies how well the machine learning model is performing by a measuring the error between its predictions and the actual target values. The goal during training is to minimize loss function.
12. Explain regularization in machine learning.
Ans:
Regularization is the technique used to prevent overfitting in the machine learning models. It adds the penalty term to loss function, discouraging the model from a learning complex patterns that might fit training data noise.
13. What is curse of dimensionality, and how does it affect machine learning?
Ans:
The curse of the dimensionality refers to challenges that arise when dealing with the high-dimensional data. As the number of features (dimensions) increases, amount of data needed to make more reliable predictions also increases, making a some algorithms less effective.
14. Differentiate between the classification and regression in machine learning?
Ans:
Aspect | Classification | Regression | |
Objective |
Assign data points to predefined classes. |
Predict a continuous numerical output. | |
Output Type | Categorical (class labels). | Continuous numerical values. | |
Example Algorithms | Logistic Regression, Decision Trees, SVM, | Linear Regression, Ridge Regression, Lasso |
15. What is bias in machine learning model. How address bias in AI systems?
Ans:
Bias in the machine learning model occurs when it consistently makes the predictions that are systematically different from a true values. To address bias, it’s crucial to ensure the diverse and representative training data and employ techniques like a re-sampling and re-weighting.
16.What are challenges in deploying machine learning models into production?
Ans:
Deploying the machine learning models into production involves the challenges such as managing model versions, scalability, monitoring for model drift, and ensuring a model fairness and security in the real-world applications.
17. What is kernel in context of support vector machines (SVMs)?
Ans:
A kernel in SVMs is the function that computes a dot product between the two data points in the higher-dimensional space. Kernels allow the SVMs to work effectively in the non-linearly separable data by transforming it into the higher-dimensional space.
18. Explain batch gradient descent in machine learning?
Ans:
Batch gradient descent is the optimization algorithm where a model’s parameters are updated using the gradient of loss function computed over the entire training dataset. It can be computationally expensive but usually converges to the more precise solution.
19. What is stochastic gradient descent (SGD), and why preferred over batch gradient descent?
Ans:
SGD is optimization algorithm that updatesa model’s parameters using a gradient of the loss function computed on single random training sample. It’s preferred over the batch gradient descent for its faster convergence, especially with the large datasets.
20. Explain bias-variance decomposition in context of expected prediction error?
Ans:
The expected prediction error can be decomposed into the three components: bias squared, variance, and irreducible error. Bias squared represents error due to model simplifications, variance represents error due to model complexity, and irreducible error is a noise inherent in the data.
21. Difference between generative and discriminative models in machine learning?
Ans:
Generative models model the joint probability distribution of the input features and target labels, while discriminative models model the conditional probability of target tags given as input features.
22. Explain cross-entropy in the context of logistic regression?
Ans:
Cross-entropy is the loss function used in the logistic regression to measure the dissimilarity between the predicted probabilities and actual class labels. It is particularly useful for binary classification problems.
23. What is activation functions in neural networks?
Ans:
Activation functions introduce a non-linearity into the neural networks, allowing them to learn a complex relationships in data. Common activation functions include the ReLU, sigmoid, and tanh.
24. What is vanishing gradient problem in deep learning, and how mitigated?
Ans:
The vanishing gradient problem occurs when a gradients during training become too small, hindering a learning process in deep neural networks. Techniques like using an appropriate activation functions and batch normalization can help to mitigate this issue.
25. Explain dropout in neural networks.?
Ans:
Dropout is the regularization technique where randomly selected neurons are dropped out (ignored) during training. It helps to prevent overfitting by promoting more robust feature learning.
26. What is K-nearest neighbors (K-NN) algorithm, and how does it work?
Ans:
K-NN is the simple machine-learning algorithm used for both the classification and regression tasks. It works by finding a K data points in the training set closest to the test point and making predictions based on labels (for classification) or values (for regression).
27. What are hyperparameters, and how different from model parameters?
Ans:
Hyperparameters are the settings or configurations of machine-learning model that are not learned from a data. Model parameters, on the other hand, are learned from data during training. Hyperparameters include the things like learning rate, batch size, and number of hidden layers.
28. Explain confusion matrix in context of binary classification?
Ans:
A confusion matrix is the table used to evaluate a performance of a binary classification model. It includes the four metrics: true positives, true negatives, false positives, and false negatives, which are used to calculate the e various evaluation metrics like the accuracy, precision, recall, and F1-score.
29. What is bagging, and how does it improve performance of machine learning models?
Ans:
Bagging is the ensemble learning technique that combines the multiple base models (usually decision trees) by training them on different subsets of the data and averaging predictions. It reduces the variance and improves the model stability.
30. What is boosting, and how does it differ from bagging?
Ans:
Boosting is another ensemble learning technique that combines multiple weak learners (e.g., shallow decision trees) sequentially, with each learner focusing on mistakes made by the previous ones. Boosting aims to reduce bias and variance, often leading to higher accuracy than bagging.
31. What is the ROC curve, and how is it used to evaluate classification models?
Ans:
The ROC (Receiver Operating Characteristic) curve is the graphical representation of the binary classification model’s performance across the different threshold values. It helps assess a trade-off between sensitivity (actual positive rate) and specificity (true negative momentum) and is used to choose the appropriate threshold.
32. What is AUC (Area Under Curve) in the context of the ROC curve?
Ans:
The AUC is the scalar value that represents the overall performance of a binary classification model. A higher AUC indicates better model discrimination, with the perfect model having an AUC of 1.
33. Explain the neural network’s architecture, including layers and nodes?
Ans:
A neural network’s architecture refers to the structural layout, which includes the input, hidden, and output layers. Nodes or neurons within the Ayers process and transmit information through the weighted connections.
34. What is feature scaling, and why is it essential in machine learning?
Ans:
Feature scaling standardises or normalises a range of independent variables or features in the data. It’s important because it ensures that features with different scales contribute equally to the model’s performance, preventing some features from dominating others.
35. What is one-hot encoding, and when used in machine learning?
Ans:
One-hot encoding is the technique used to convert categorical variables into the binary matrix format. Every category becomes a binary feature, crucial when dealing with categorical data in machine learning models.
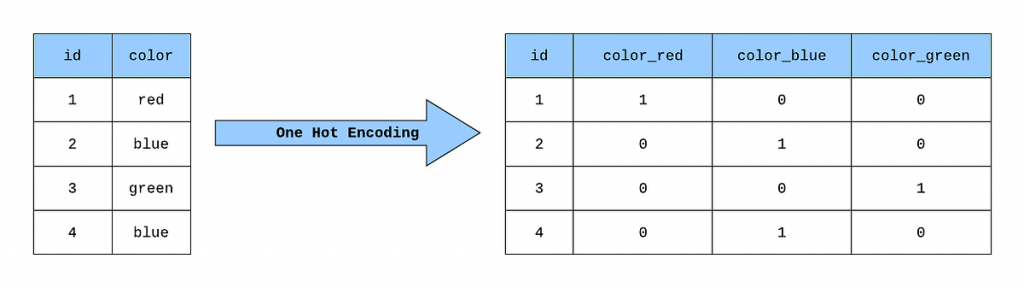
36. Explain bias in machine learning algorithms and how it can lead to unfairness.
Ans:
Bias in machine learning refers to the systematic errors or inaccuracies in the predictions that disproportionately favour or disfavour certain groups. It can lead to unfairness when models discriminate against protected attributes (e.g., gender, race) in forecasts.
37. What is the curse of dimensionality, and how can it affect machine learning algorithms?
Ans:
The curse of dimensionality refers to increased data sparsity and computational complexity as the dataset’s number of features (dimensions) grows. It can affect the performance of machine learning algorithms, making them less effective or requiring more data.
38. Explain transfer learning in deep learning and provide an example?
Ans:
Transfer learning involves using a pre-trained neural network on the related task as a starting point for a new job. For example, we can take a pre-trained image classification model and fine-tune it for the specific image recognition task, saving time and resources.
39. What is the L1 regularization term, and how does it differ from L2 regularization?
Ans:
L1 regularization adds the penalty term to the loss function based on absolute values of model weights. It tends to encourage sparse weight vectors. L2 regularization, on the other hand, adds a penalty based on squared values of weights and enables small but non-zero weights.
40. Explain data augmentation in deep learning?
Ans:
Data augmentation involves :
- Creating a new training example by applying the various transformations (e.g., Rotation.
- Flipping) to existing data.
41. What is the Difference between underfitting and overfitting in machine learning?
Ans:
Underfitting occurs when the model is too simple to capture underlying patterns in data, resulting in poor performance on both the training and test datasets. Conversely, overfitting occurs when the model is too complex and fits the training data too closely, resulting in poor performance on test data.
42. What is a neural network activation function, and why is it necessary?
Ans:
An activation function introduces a non-linearity into the neural network, allowing it to learn complex relationships in data. It transforms the weighted sum of input values into an output value for each neuron.
43. Explain bias in a neural network?
Ans:
Bias in the neural network is an additional learnable parameter that allows the model to make predictions even when all the input features are zero. It helps to model capture patterns not solely determined by input features.
44. What is the purpose of learning rate in gradient descent optimization?
Ans:
The learning rate is the hyperparameter that controls a step size of parameter updates during the gradient descent. It affects the speed and convergence of the optimization process, and choosing the appropriate learning rate is crucial for training neural networks effectively.
45. What is CNN?
Ans:
A Convolutional Neural Network is a deep neural network designed for processing and analyzing visual data, like images and videos. They are commonly used in computer vision tasks like image classification, object detection, and image segmentation.
46. Explain RNN and its application in sequential data analysis?
Ans:
An RNN is a type of neural network well-suited for sequential data, where the order of the data points matters. RNNs have connections that loop back on themselves, allowing them to maintain a hidden state that captures information from previous time steps. They are used in natural language processing, speech recognition, and time series forecasting.
47. What are word embeddings in natural language processing?
Ans:
A word embedding is the dense vector representation of words in the natural language. It captures semantic relationships between terms and allows algorithms to understand words’ meaning in textual data better, improving the performance of tasks like text classification and sentiment analysis.
48. Explain decision boundary in machine learning?
Ans:
A decision boundary is a hypersurface that separates different classes or groups in the classification problem. It is determined by the machine learning model and is used to predict which type the new data point belongs to.
49. What is the difference between parametric and non-parametric machine learning algorithms?
Ans:
Parametric algorithms make assumptions about the model’s functional form (e.g., linear regression). In contrast, non-parametric algorithms do not make solid assumptions and can adapt to complex data patterns (e.g., k-nearest neighbours).
50. What is reinforcement learning, and how does it differ from supervised learning?
Ans:
Reinforcement learning is the type of machine learning where an agent learns to make decisions by interacting with the environment and receiving feedback in the form of rewards. It differs from supervised learning in that it does not require the labelled data but instead learns through trial and error.
51. Explain Q-learning in reinforcement learning?
Ans:
Q-learning is the model-free reinforcement learning algorithm used to find optimal action-selection policy for the given finite Markov decision process. It learns the Q-value for each state-action pair and uses these values to make decisions that maximize an expected rewards.
52. What is trade-off between exploration and exploitation in reinforcement learning?
Ans:
The exploration-exploitation trade-off involves the balancing the agent’s desire to try a new actions (exploration) with its desire to choose the actions that are known to yield to high rewards (exploitation). Striking a right balance is crucial for an effective learning in reinforcement learning tasks.
53. Explain Markov Decision Process (MDP) in reinforcement learning?
Ans:
An MDP is the mathematical framework used to model the decision-making in situations where outcomes are partly random and partly under the control of the decision maker. It consists of the states, actions, transition probabilities, rewards, and a policy.
54. What are recurrent neural networks (RNNs), and how do handle sequential data?
Ans:
Recurrent Neural Networks (RNNs) are the type of neural network designed to work with the sequences of data. They use a recurrent connections to maintain the hidden state that captures information from a previous time steps, allowing them to model sequential dependencies in a data.
55. What is gradient clipping?
Ans:
Gradient clipping is the technique used to prevent an exploding gradients during the training of deep neural networks. It involves the capping the gradient values during the backpropagation to a predefined threshold, ensuring a stable and more reliable training.
56. What is batch normalization?
Ans:
Batch normalization is the technique used to normalize the input of each layer in the neural network within a mini-batch. It helps to stabilize and speed up training by reducing the internal covariate shift and making an optimization more predictable.
57. What are autoencoders in deep learning?
Ans:
Autoencoders are the neural network architectures used for the unsupervised learning and dimensionality reduction. They are often used for a tasks like image denoising, anomaly detection, and feature learning.
58. What is F1 score?
Ans:
The F1 score is the metric that combines both the precision and recall to provide a single value that balances trade-off between false positives and false negatives in the classification model’s predictions. It is particularly useful when dealing with the imbalanced datasets.
59. Difference between bagging and boosting in ensemble learning?
Ans:
Bagging (Bootstrap Aggregating) involves the training multiple base models independently on a different subsets of the data and combining their predictions, typically by averaging (e.g., Random Forest). Boosting, on other hand, combines the multiple weak learners sequentially, with each learner focusing on a mistakes made by previous ones (e.g., AdaBoost, Gradient Boosting).
60. What are some real-life applications of clustering algorithms?
Ans:
The clustering technique can be used in the multiple domains of data science like the image classification, customer segmentation, and recommendation engine. One of the most common use is in the market research and customer segmentation which is utilized to target a particular market group to expand businesses and profitable outcomes.
61. How to choose optimal number of clusters?
Ans:
By using Elbow method decide an optimal number of clusters that clustering algorithm must try to form. The main principle behind this method is that if will increase the number of clusters error value will decrease. But after optimal number of features, the decrease in error value is insignificant so, at point after which this starts to be happen, choose that point as optimal number of clusters that algorithm will try to form.
62. What is Hypothesis in Machine Learning?
Ans:
A hypothesis is the term that is generally used in a Supervised machine learning domain. As have independent features and target variables and try to find an approximate function mapping from feature space to a target variable that approximation of mapping is known as the hypothesis.
63. How do measure effectiveness of clusters?
Ans:
- Data collected by the interested/self is primary data. This data is collected afresh and first time.
- Someone else has collected the data and being used by you is secondary data.
64. Why do take smaller values of learning rate?
Ans:
Smaller values of the learning rate help the training process to converge the more slowly and gradually toward global optimum instead of fluctuating around it. This is because smaller learning rate results in the smaller updates to model weights at each iteration, which can help to ensure that updates are the more precise and stable.
65. Why do perform normalization?
Ans:
To achieve stable and fast training of a model use normalization techniques to bring all features to a certain scale or range of values. If do not perform normalization then there are chances that gradient will not converge to a global or local minima and end up oscillating back and forth..
66. Difference between precision and recall?
Ans:
Precision is the simply the ratio between true positives(TP) and all the positive examples (TP+FP) predicted by a model. In other words, precision measures how many of predicted positive examples are actually true positives. It is the measure of the model’s ability to the avoid false positives and make accurate positive predictions. But in case of a recall, calculate the ratio of true positives (TP) and total number of examples (TP+FN) that actually fall in a positive class. recall measures how many of actual positive examples are the correctly identified by model. It is the measure of model’s ability to avoid false negatives and identify all the positive examples correctly.
67. Difference between upsampling and downsampling?
Ans:
In upsampling method, increase the number of samples in minority class by randomly selecting some points from a minority class and adding them to the dataset repeat this process till dataset gets balanced for each class. But here is disadvantage the training accuracy becomes high as in every epoch model trained more than once in each epoch but a same high accuracy is not observed in validation accuracy. In case of downsampling, decrease the number of samples in majority class by selecting a some random number of points that are equal to number of data points in minority class so that distribution becomes balanced. In this case, have to suffer from a data loss which may lead to a loss of some critical information as well.
68. What is data leakage and how can identify it?
Ans:
If there is high correlation between a target variable and input features then this situation is referred to as a data leakage. This is because when train the model with that highly correlated feature then model gets most of a target variable’s information in training process only and it has to do very little to achieve high accuracy. In this situation, model gives pretty decent performance both on training as well as validation data but as use that model to make the actual predictions then model’s performance is not up to a mark. This is how can identify data leakage.
69. Difference between covariance and correlation?
Ans:
As name suggests, Covariance provides us with the measure of the extent to which two variables differ from the each other. But on other hand, correlation gives us a measure of the extent to which two variables are related to the each other. Covariance can take on the any value while correlation is always between -1 and 1. These measures are used during exploratory data analysis to gain the insights from a data.
70. What is use of violin plot?
Ans:
The name violin plot has been derived from a shape of the graph which matches violin. This graph is the extension of Kernel Density Plot along with the properties of a boxplot. All statistical measures shown by the boxplot are also shown by a violin plot but along with this, The width of violin represents the density of the variable in different regions of values. This visualization tool is generally used in a exploratory data analysis step to check distribution of continuous data variables.
71. Difference between stochastic gradient descent (SGD) and gradient descent (GD)?
Ans:
In gradient descent algorithm train the model on the whole dataset at once. But in the Stochastic Gradient Descent, model is trained by using a mini-batch of training data at once. If using SGD then one cannot expect training error to go down smoothly. The training error oscillates but after some training steps, can say that training error has gone down. Also, minima achieved by using the GD may vary from that achieved using SGD. It is observed that minima achieved by using SGD are close to the GD but not a same.
72. What is Central Limit theorem?
Ans:
This theorem is related to the sampling statistics and its distribution. As per this theorem sampling distribution of sample means tends to towards a normal distribution as a sample size increases. No matter how population distribution is shaped. i.e if take some sample points from a distribution and calculate its mean then distribution of those mean points will follow the normal/gaussian distribution no matter from which distribution have taken the sample points. There is one condition that size of the sample must be greater than or equal to 30 for CLT to hold. and the mean of sample means approaches the population mean.
73. Explain SMOTE method used to handle data imbalance?
Ans:
The synthetic Minority Oversampling Technique is one of the methods which is used to handle a data imbalance problem in a dataset. In this method, synthesized a new data points using existing ones from the minority classes by using the linear interpolation. The advantage of using this method is that model does not get trained on same data
74. What is KNN Imputer?
Ans:
Generally impute the null values by a descriptive statistical measures of a data like mean, mode, or median but KNN Imputer is the more sophisticated method to fill the null values. A distance parameter is also used in this method which is also known as a k parameter. The work is somehow similar to clustering algorithm. The missing value is imputed in the reference to a neighborhood points of missing values.
75. Explain working procedure of the XGB model?
Ans:
XGB model is the example of ensemble technique of machine learning in this method weights are optimized in the sequential manner by passing them to decision trees. After each pass, weights become better and better as each tree tries to optimize weights, and finally, obtain the best weights for a problem at hand. Techniques like a regularized gradient and mini-batch gradient descent have been used to implement this algorithm so, that it works in the very fast and optimized manner.
76. What is splitting given dataset into training and validation data?
Ans:
The main purpose is to keep a some data left over on which model has not been trained so, that can evaluate a performance of machine learning model after training. Also, sometimes use the validation dataset to choose among multiple state-of-the-art machine learning models. Like first train some models let’s say LogisticRegression, XGBoost, or any other than test performance using the validation data and choose model which has less difference between validation and the training accuracy.
77. Explain some methods to handle missing values in data?
Ans:
- Removing a rows with the null values may lead to loss of some important information.
- Removing a column having null values if it has very less valuable information. it may lead to loss of some important information.
- Imputing null values with the descriptive statistical measures like a mean, mode, and median.
- Using methods like the KNN Imputer to impute null values in the more sophisticated way.
78. Difference between k-means and KNN algorithm?
Ans:
k-means algorithm is one of popular unsupervised machine learning algorithms which is used for a clustering purposes. But KNN is the model which is generally used for classification task and is the supervised machine learning algorithm. The k-means algorithm helps us to label a data by forming clusters within dataset.
79. How can visualize high-dimensional data in 2-d?
Ans:
One of the most common and effective methods is by using a t-SNE algorithm which is the short form for t-Distributed Stochastic Neighbor Embedding. This algorithm uses the some non-linear complex methods to reduce dimensionality of a given data. Can also use PCA or LDA to convert n-dimensional data to 2 – dimensional so, that can plot it to get the visuals for a better analysis. But difference between the PCA and t-SNE is that former tries to preserve the variance of the dataset but t-SNE tries to preserve local similarities in a dataset.
80. Why is “Naive” Bayes naive?
Ans:
Despite its practical applications, especially in the text mining, Naive Bayes is considered “Naive” because it makes the assumption that is virtually impossible to see in a real-life data: conditional probability is calculated as pure product of individual probabilities of components. This implies absolute independence of features — a condition probably never met in a real life.
81. What’s Fourier transform?
Ans:
A Fourier transform is the generic method to decompose a generic functions into the superposition of symmetric functions. Or as this more intuitive tutorial puts it, given smoothie, it’s how find the recipe. The Fourier transform finds a set of cycle speeds, amplitudes, and phases to match the any time signal. A Fourier transform converts the signal from time to frequency domain—it’s common way to extract features from the audio signals or the other time series such as sensor data.
82. How is decision tree pruned?
Ans:
Pruning is what happens in decision trees when branches that have a weak predictive power are removed in order to reduce complexity of a model and increase predictive accuracy of the decision tree model. Pruning can happen bottom-up and top-down, with the approaches such as reduced error pruning and cost complexity pruning.
83. How would handle an imbalanced dataset?
Ans:
An imbalanced dataset is when have, for example, a classification test and 90% of the data is in a one class. That leads to problems: an accuracy of 90% can be skewed if have no predictive power on other category of data.
84. When should use classification over regression?
Ans:
Classification produces a discrete values and dataset to the strict categories, while regression gives continuous results that allow to better distinguish differences between the individual points. would use the classification over regression if wanted results to reflect the belongingness of data points in the dataset to certain explicit categories.
85. What’s “kernel trick” and how is it useful?
Ans:
The Kernel trick involves the kernel functions that can enable in the higher-dimension spaces without explicitly calculating a coordinates of points within that dimension: instead, kernel functions compute inner products between images of all the pairs of data in the feature space. This allows them very useful attribute of calculating a coordinates of higher dimensions while being computationally cheaper than explicit calculation of said coordinates. More algorithms can be expressed in terms of the inner products. Using kernel trick enables us effectively run the algorithms in high-dimensional space with the lower-dimensional data.
86. Describe hash table?
Ans:
A hash table is the data structure that produces the associative array. A key is mapped to a certain values through use of a hash function. They are often used for a tasks such as database indexing.
87. How are primary and foreign keys related in SQL?
Ans:
Most machine learning engineers are going to have to be a conversant with the lot of different data formats. SQL is still one of key ones used. Ability to understand how to manipulate a SQL databases will be something will most likely need to demonstrate. In this example, can talk about how foreign keys allow to match up and join tables together on a primary key of corresponding table—but just as useful is to talk through how would think about setting up SQL tables and querying them.
88. How would build a data pipeline?
Ans:
Data pipelines are bread and butter of machine learning engineers, who take a data science models and find ways to automate and scale them. Make sure familiar with the tools to build a data pipelines (such as Apache Airflow) and platforms where can host models and pipelines (such as Google Cloud or AWS or Azure).
89. What are three stages of model building in machine learning?
Ans:
Model Building : In this stage,will choose the ideal algorithm for a model, and will train it based on the requirements.
Model Testing : In this stage, will check model’s accuracy by using a test data. Applying Model: After testing, have to make changes, and then can use model for a real-time projects.
90. What are applications of supervised machine learning?
Ans:
- Fraud Identification
- Healthcare
- Email spam identification
- Sentiment Analysis