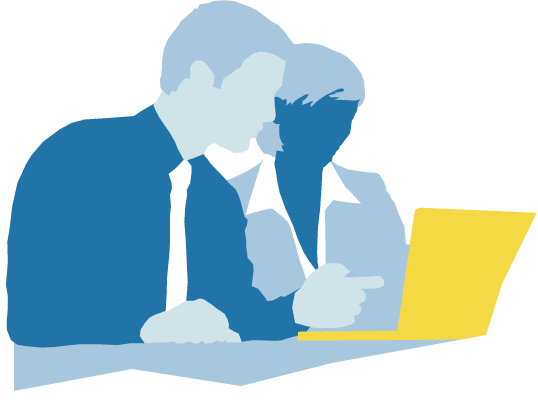
Live Instructor LED Online Training
Learn from Certified Experts
- Beginner & Advanced level Classes.
- Hands-On Learning in R Programming.
- Best Practice for interview Preparation Techniques in R Programming.
- Lifetime Access for Student’s Portal, Study Materials, Videos & Top MNC Interview Question.
- Affordable Fees with Best curriculum Designed by Industrial R Programming Expert.
- Delivered by 9+ years of R Programming Certified Expert | 12402+ Students Trained & 350+ Recruiting Clients.
- Next R Programming Batch to Begin this week – Enroll Your Name Now!