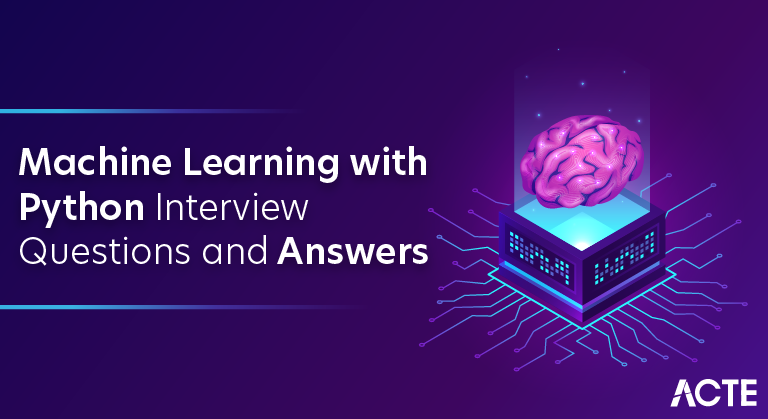
25+ BEST Machine Learning [ Python ] Questions | SURE SHOT
Last updated on 04th Jul 2020, Blog, Interview Questions
Machine learning is a field of artificial intelligence that focuses on developing algorithms and models capable of learning patterns from data to make predictions or decisions without explicit programming. In Python, machine learning is commonly implemented using libraries such as sci-kit-learn, TensorFlow, and PyTorch, offering a wide range of tools for tasks like classification, regression, clustering, and deep learning. Python’s simplicity and extensive libraries make it a popular choice for machine learning development.
1. What is machine learning, and how does it differ from traditional programming?
Ans:
Machine learning is a subfield of artificial intelligence (AI) that focuses on the development of algorithms and models that enable computers to learn from data and make predictions or decisions without being explicitly programmed. In other words, instead of relying on explicit programming, machine learning allows systems to learn and improve from experience. Python is a popular programming language for implementing machine learning algorithms due to its extensive libraries and frameworks, such as TensorFlow, PyTorch, and sci-kit-learn.
2. How does machine learning differ from traditional programming?
Ans:
In traditional programming, developers explicitly write code to instruct a computer on how to perform a specific task or solve a particular problem. The rules and logic governing the program are explicitly defined by the programmer based on their understanding of the problem domain. The program follows these instructions and produces the desired output.
While traditional programming relies on explicit instructions created by developers, machine learning leverages data-driven learning to enable systems to make decisions or predictions based on patterns and relationships found in the data.
3. Explain the difference between supervised and unsupervised learning.
Ans:
Supervised learning involves training a model on labeled data with known outputs, aiming to make predictions on new, unseen data. Unsupervised learning, on the other hand, deals with unlabeled data, focusing on uncovering patterns or structures within the data without explicit output guidance.
Supervised Learning:
- In supervised learning, the algorithm is trained on a labeled dataset, where each input data point is paired with its corresponding output or target.
Unsupervised Learning:
- In unsupervised learning, the algorithm is given unlabeled data and is tasked with finding patterns, relationships, or structures within the data without explicit guidance on the output.
4. What is the purpose of the train-test split in machine learning, and how do you implement it in Python?
Ans:
The purpose of the train-test split in machine learning is to assess how well a trained model generalizes to new, unseen data. The dataset is typically divided into two subsets: the training set, used to train the model, and the test set, used to evaluate its performance. This helps to estimate how the model will perform on data it has not seen during training, providing a more realistic evaluation of its effectiveness. In Python, you can implement the train-test split using libraries such as sci-kit-learn.
5. Describe the concept of overfitting in machine learning. How can it be prevented or mitigated?
Ans:
Overfitting occurs when a machine learning model learns the training data too well, including its noise and outliers, to the extent that it negatively impacts the model’s ability to generalize to new, unseen data. Essentially, the model becomes too complex, capturing the idiosyncrasies of the training data rather than learning the underlying patterns. As a result, an overfit model may perform well on the training data but poorly on new data. By employing a combination of these techniques, practitioners can effectively prevent or mitigate overfitting and build models that generalize well to new, unseen data.
6. What is the role of feature scaling in Python?
Ans:
Feature scaling is a preprocessing step in machine learning that standardizes or normalizes the range of independent variables or features of the dataset. The goal is to ensure that all features contribute equally to the model’s training process, preventing certain features from dominating due to their larger scale. Feature scaling is particularly crucial for algorithms that are sensitive to the scale of input features, such as gradient-based optimization algorithms in many machine-learning models.
Performing Feature Scaling in Python:
- After scaling the features, they can be used as input for training your machine-learning model. It’s important to note that the choice between Min-Max Scaling and Standardization depends on the requirements of the specific algorithm you are using.
7. Can you explain the difference between classification and regression algorithms in machine learning?
Ans:
Feature | Classification | Regression | |
Objective |
Predicts class/category |
Predicts continuous value/quantity | |
Output | Discrete labels | Continuous values | |
Applications | Spam detection, image recognition | House price prediction, stock forecasting | |
Evaluation | Accuracy, precision, recall | Configured in the web.xml file |
8. What is cross-validation, and why is it important in model evaluation?
Ans:
Cross-validation is a model evaluation technique used to assess the performance and generalization ability of a machine learning model. The primary purpose of cross-validation is to provide a more robust estimate of a model’s performance by using multiple splits of the dataset into training and testing subsets. Across-validation is a crucial technique in model evaluation as it provides a more reliable estimate of a model’s performance, helps detect issues like overfitting, and aids in optimizing hyperparameters for better generalization.
9. How does a decision tree work, and how can it be visualized in Python?
Ans:
A decision tree is a supervised machine-learning algorithm used for both classification and regression tasks. It works by recursively partitioning the data into subsets based on the most significant attribute at each node, forming a tree-like structure. The decision-making process involves traversing the tree from the root to a leaf node, where the final prediction is made.
Visualizing a Decision Tree in Python:
- To visualize a decision tree in Python, you can use the plot_tree function from the scikit-learn library.
10. Explain the terms “precision” and “recall” in the context of classification metrics.
Ans:
In classification, “precision” gauges the accuracy of positive predictions, while “recall” evaluates the model’s capability to detect all true positives. Precision is significant in scenarios where minimizing false positives is crucial, as seen in spam detection. Conversely, recall is essential when minimizing false negatives is a priority, particularly in medical diagnoses. The combined assessment of both metrics provides a thorough evaluation of the model’s performance.
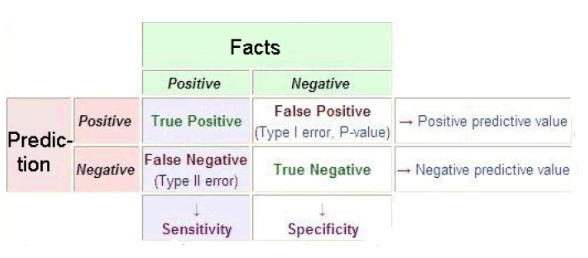
11. What is the purpose of regularization in machine learning, and how is it implemented in models like linear regression?
Ans:
Regularization is a technique used in machine learning to prevent overfitting and improve the generalization ability of a model. Overfitting occurs when a model learns the training data too well, capturing noise and outliers but fails to generalize to new, unseen data. Regularization introduces a penalty term to the model’s objective function, discouraging it from becoming too complex or over-reliant on certain featuresRegularization is a powerful tool to enhance the performance and robustness of machine learning models, particularly in scenarios with limited training data.
12. What is the curse of dimensionality, and how does it affect machine learning models?
Ans:
The curse of dimensionality refers to challenges that arise when dealing with high-dimensional data. As the number of features or dimensions increases, the amount of data needed to generalize accurately grows exponentially. This can lead to sparse data, increased computational complexity, and overfitting in machine learning models. To mitigate the curse of dimensionality, feature selection, dimensionality reduction techniques (e.g., PCA), and thoughtful preprocessing are often employed in machine learning workflows.
13. Explain the concept of overfitting and how it can be prevented.
Ans:
Overfitting occurs when a machine learning model learns the training data too well, capturing noise or random fluctuations in the data instead of the underlying patterns. As a result, the model performs well on the training set but fails to generalize to new, unseen data. Balancing model complexity, dataset size, and regularization helps prevent overfitting, ensuring that the model generalizes well to new, unseen data.
14. What is cross-validation, and why is it important in machine learning?
Ans:
Cross-validation is a statistical technique used in machine learning to assess the performance and generalizability of a model. It involves partitioning the dataset into multiple subsets, training the model on some of these subsets, and evaluating its performance on the remaining subsets. This process is repeated multiple times, and the average performance is used to estimate how the model is likely to perform on unseen data. Cross-validation is a crucial technique in machine learning for robustly evaluating and optimizing models, providing a more reliable indication of their generalization performance.
15. Compare and contrast classification and regression in the context of machine learning.
Ans:
Classification and regression differ in their objectives, output types, evaluation metrics, and the nature of the target variable. While classification deals with discrete classes, regression deals with continuous values, making them suitable for different types of machine-learning tasks.
16. What is the purpose of activation functions in neural networks?
Ans:
Activation functions in neural networks serve the crucial role of introducing non-linearity to the network. They allow neural networks to learn complex relationships and patterns in data by enabling the stacking of multiple layersActivation functions play a crucial role in shaping the behavior of neural networks, and the choice of activation function depends on the specific characteristics of the problem at hand.
17. Explain the bias-variance tradeoff in the context of machine learning models.
Ans:
The bias-variance tradeoff is a fundamental concept in machine learning that involves balancing two sources of error in a predictive model: bias and variance. Striking the right balance between bias and variance is essential for building models that generalize well to new, unseen data.
Implications:
- Underfitting (High Bias): The model needs to be more complex, capture underlying patterns, and perform better on both training and test data.
- Overfitting (High Variance): The model fits the training data too closely, capturing noise, and performs well on training data but poorly on new data.
18. What are hyperparameters, and how do they differ from parameters in machine learning models?
Ans:
In machine learning, both hyperparameters and parameters are essential components of a model, but they serve distinct roles. Optimizing hyperparameters is a crucial step in building effective machine-learning models, as the right configuration can significantly impact a model’s performance. Parameters, on the other hand, are learned from the data and adapted during the training process to capture patterns.
19. Explain Bagging and boosting in machine learning
Ans:
Bagging and boosting are ensemble learning techniques that aim to improve the performance of machine learning models. Bagging builds diverse models in parallel to reduce variance, while boosting builds models sequentially, focusing on instances that are challenging to classify to improve accuracy.
Example Algorithms:
- AdaBoost (Adaptive Boosting): Weak learners (e.g., decision trees) are trained sequentially, and each subsequent model corrects errors made by the previous ones.
- Gradient Boosting: Iteratively builds decision trees to correct errors of the preceding models. Examples include XGBoost, LightGBM, and CatBoost.
20. What is the purpose of regularization in machine learning, and how does it work?
Ans:
Regularization is a technique used to prevent overfitting in machine learning models. Overfitting occurs when a model learns the training data too well, capturing noise and specificities that don’t generalize well to new, unseen data. Regularization helps to create models that are more robust and perform better on new, unseen data. Regularization helps achieve a balance between fitting the training data well and avoiding unnecessary complexity, promoting models that generalize better to new, unseen data.
21. Explain the concept of feature engineering and why it is important in building machine learning models.
Ans:
Feature engineering is the process of selecting, transforming, or creating features (input variables) in a dataset to improve the performance of a machine learning model. It involves extracting relevant information, enhancing existing features, and creating new ones to provide a more informative representation of the data for the model. Feature engineering is a crucial aspect of building effective machine-learning models. It involves crafting input features to improve a model’s ability to learn and generalize patterns from data, ultimately leading to better predictive performance.
22. What is gradient descent, and how is it used in training machine learning models?
Ans:
Gradient descent is an iterative optimization algorithm used for finding the minimum of a function. In the context of machine learning, this function is the loss function or cost function, which measures the difference between the predicted values and the actual values. The goal of gradient descent is to adjust the model parameters (weights and biases) to minimize this loss function. Gradient descent is a fundamental optimization algorithm in machine learning, providing a systematic way to update model parameters and improve the model’s fit to the training data.
23. Discuss the differences between L1 and L2 regularization.
Ans:
L1 regularization introduces a penalty term based on the absolute values of the coefficients, promoting sparsity by encouraging some coefficients to become exactly zero. On the other hand, L2 regularization penalizes the squared values of the coefficients, preventing them from becoming too large and helping to avoid overfitting by keeping the model’s weights more balanced. Combining both L1 and L2 regularization is known as Elastic Net regularization, offering a balance between sparsity and coefficient size control.L1 regularization encourages sparsity and feature selection, while L2 regularization penalizes large coefficients to prevent overfitting and maintain a more balanced model.
24. Explain the ROC curve and its significance in binary classification.
Ans:
The Receiver Operating Characteristic (ROC) curve is a graphical representation of the trade-off between true positive rate (sensitivity) and false positive rate (1-specificity) across different threshold values in binary classificationThe ROC curve plots these rates at various threshold settings, helping to visualize the model’s performance across a range of classification scenarios. The area under the ROC curve (AUC-ROC) summarizes the overall performance of the classifier. A higher AUC-ROC indicates better discrimination between positive and negative classes.
25. What is transfer learning, and how can it be applied in machine learning?
Ans:
Transfer learning is a machine learning technique where a model developed for a particular task is reused as the starting point for a model on a second task. Instead of training a model from scratch, transfer learning leverages the knowledge gained from solving one problem and applies it to a different but related problem. Transfer learning is beneficial when you have a limited amount of data for your specific task, as it helps overcome data scarcity issues by leveraging knowledge from a larger dataset. It has been successfully applied in various domains, including computer vision, natural language processing, and speech recognition.
26. Explain that K-means clustering and hierarchical clustering are both techniques used in unsupervised machine learning.
Ans:
K-means clustering and hierarchical clustering are both techniques used in unsupervised machine learning for grouping similar data points, but they differ in several aspects. Choosing between K-means and hierarchical clustering depends on the nature of the data, the desired number of clusters, and the interpretability of the results. K-means is often preferred when a specific number of clusters is known, while hierarchical clustering is more exploratory and flexible in determining the cluster structure.
27. Explain the concept of ensemble learning. Provide examples of ensemble learning algorithms.
Ans:
Ensemble learning is a machine learning technique that combines the predictions of multiple models to produce a more robust and accurate prediction than any individual model. The idea is that by combining diverse models, each capturing different aspects of the data, the ensemble can perform better than its individual componentsEnsemble learning is widely used because it can enhance the overall performance and generalization of models, especially in situations where individual models may struggle. It is a powerful approach for improving model stability and predictive accuracy across various machine-learning tasks.
28. What is the purpose of a confusion matrix in the evaluation of classification models?
Ans:
A confusion matrix is a table that is used to evaluate the performance of a classification model. It provides a detailed breakdown of the model’s predictions compared to the actual classes in the dataset. The matrix is particularly useful when dealing with binary or multiclass classification problemsThe confusion matrix is valuable because it provides a more detailed understanding of how a classifier is performing, especially in terms of the types of errors it makes. It helps in assessing the trade-offs between different evaluation metrics and can guide model improvement strategies. For instance, a model with high accuracy might still perform poorly in terms of precision or recall, and the confusion matrix helps to identify such nuances in classification performance.
29. What is cross-validation, and why is it important in machine learning?
Ans:
Cross-validation is a technique used in machine learning to assess the performance and generalizability of a model. It involves splitting the dataset into multiple subsets, training the model on some of them, and then evaluating it on the remaining subsets. This helps estimate how well the model will perform on unseen data, reducing the risk of overfitting and providing a more reliable performance measure.
30. What is feature engineering, and why is it important in machine learning?
Ans:
Feature engineering involves creating new features or modifying existing ones to improve a machine learning model’s performance. It plays a crucial role in enhancing a model’s ability to capture patterns and relationships in the data. Effective feature engineering can lead to better predictive performance and more robust modelsEffective feature engineering depends on a good understanding of the domain and the data, and it can significantly impact the success of a machine learning model by providing it with the right input information.
31. Describe the bias-variance trade-off. How does it relate to overfitting and underfitting?
Ans:
The bias-variance trade-off is a key concept in machine learning that deals with finding the right balance between bias and variance when building a model. The trade-off occurs because decreasing bias often increases variance and vice versa. Achieving the right balance is crucial for building a model that generalizes well to new data. Overfitting tends to have low bias and high variance, while underfitting has high bias and low variance. Cross-validation is often used to find this balance and to identify whether a model is overfitting or underfitting by evaluating its performance on different subsets of the data.
32. Explain the concept of regularization. How does it help prevent overfitting in machine learning models?
Ans:
Regularization is a technique used in machine learning to prevent overfitting, which occurs when a model fits the training data too closely, capturing noise and fluctuations rather than the underlying patterns. The primary goal of regularization is to impose constraints on the model’s complexity, discouraging overly complex models that might perform well on the training data but fail to generalize to new, unseen data. By introducing regularization, the model is forced to find a balance between fitting the training data well and keeping the model’s parameters within reasonable bounds. This results in a more generalized model that is less likely to overfit and performs better on new, unseen data. The choice of the regularization strength is a crucial aspect, and it is often determined through techniques like cross-validation.
33. What are hyperparameters, and how are they different from parameters in machine learning models?
Ans:
In machine learning, both hyperparameters and parameters are essential components of a model, but they serve different roles. Parameters are internal variables that the model learns during training, while hyperparameters are external settings that guide the learning process but are not learned from the data. Tuning hyperparameters effectively is crucial for achieving the best performance from a machine learning model.
34. How does the k-nearest neighbors (KNN) algorithm work? What are its strengths and weaknesses?
Ans:
The k-Nearest Neighbors (KNN) algorithm is a simple and intuitive supervised machine learning algorithm used for both classification and regression tasks. It is a versatile algorithm suitable for various tasks, but its performance can be influenced by the choice of distance metric, the number of neighbors (k), and the characteristics of the dataset. It’s often used in scenarios where interpretability and simplicity are more important than computational efficiency.
35. Explain the term “one-hot encoding” and its significance in machine learning.
Ans:
One-hot encoding is a technique used to represent categorical variables with numerical values in machine learning. It is particularly useful when dealing with algorithms that require numerical input, as most machine learning models work with numerical dataWhile one-hot encoding is valuable, it can lead to a high-dimensional dataset, especially when dealing with categorical variables with many unique categories. This can impact computational efficiency and introduce the curse of dimensionality. In such cases, other encoding techniques like label encoding or embeddings might be considered.
36. What is dimensionality reduction, and why might it be useful in machine learning?
Ans:
Dimensionality reduction is the process of reducing the number of features (or dimensions) in a dataset while retaining as much relevant information as possible. In machine learning, high-dimensional datasets with a large number of features can pose challenges, including increased computational complexity, the risk of overfitting, and difficulty in visualizing the data. Dimensionality reduction aims to address these issues. Dimensionality reduction techniques should be chosen based on the specific characteristics of the data and the goals of the analysis or modeling task. Each technique comes with its assumptions and considerations, and the choice depends on the nature of the dataset and the underlying problem.
37. What is a decision tree, and how does it work in classification problems? How is a decision tree pruned?
Ans:
A decision tree is a supervised machine-learning algorithm used for both classification and regression tasks. It works by recursively partitioning the dataset into subsets based on the values of input features, ultimately assigning a label or predicting a continuous value for each observation. Pruning ensures that the decision tree generalizes well to new, unseen data by avoiding the memorization of noise in the training set. The goal is to strike a balance between simplicity and accuracy. Decision tree algorithms often provide hyperparameters to control pruning, such as maximum depth, minimum samples per leaf, or minimum samples for split.
38. What is the purpose of the ROC curve, and how does it relate to the area under the curve (AUC) in binary classification?
Ans:
The Receiver Operating Characteristic (ROC) curve is a graphical representation used to assess the performance of a binary classification model across different thresholds. It plots the true positive rate (sensitivity) against the false positive rate (1 – specificity) at various threshold settings. The ROC curve provides insights into how well a model can distinguish between the positive and negative classes. The ROC curve and AUC are valuable tools for assessing and comparing the performance of binary classification models. They provide a comprehensive view of a model’s ability to discriminate between classes across various threshold settings.
39. How does gradient descent work in the context of training machine learning models?
Ans
Gradient descent is an optimization algorithm used to minimize the cost or loss function during the training of machine learning models. Its primary goal is to adjust the model’s parameters iteratively in the direction that leads to the steepest decrease in the cost function. Gradient descent is a fundamental optimization technique used in various machine learning algorithms, particularly in training models with large amounts of data and parameters.
40. Explain the concept of a support vector machine (SVM) and its use in classification problems. What is the kernel trick?
Ans
A Support Vector Machine (SVM) is a supervised machine learning algorithm used for classification and regression tasks. Its primary objective in classification is to find the optimal hyperplane that separates data points of different classes while maximizing the margin between the classes.
Kernel Trick:- The kernel trick involves mapping the input features into a higher-dimensional space without explicitly computing the new feature representations. This is done by using a kernel function, which computes the dot product between the transformed feature vectors in the higher-dimensional space.
41. What is the difference between bagging and boosting in ensemble learning?
Ans
Provide examples of algorithms for each. Both bagging and boosting are ensemble techniques that combine multiple models to achieve better predictive performance. Bagging aims to reduce variance while boosting focuses on improving accuracy by correcting errors sequentially.
Differences:- Bagging: Parallel training of multiple instances, each independent of the others.
- Boosting: Sequential training, with each new model focused on correcting the errors of the ensemble so far.
- Bagging: Reduces variance and prevents overfitting.
- Boosting: Tends to improve accuracy and reduce bias.
42. How can you handle missing data in a dataset for machine learning?
Ans
The choice of the method depends on the nature of the data, the extent of missingness, and the requirements of the specific machine learning task.
It’s crucial to analyze the patterns of missing data to determine the most suitable approach.
Be cautious about introducing bias or distortions in the data when imputing missing values. Evaluate the impact on the model’s performance.
Remember that there is no one-size-fits-all solution, and the best approach depends on the characteristics of the dataset and the goals of the analysis or modeling task.
43. Explain the concept of a neural network. How does it work, and what are its components?
Ans
A neural network is a computational model inspired by the structure and functioning of the human brain. It is composed of interconnected nodes, often referred to as neurons or artificial neurons, organized into layers. Neural networks are a fundamental component of deep learning, a subset of machine learning. Neural networks are capable of learning complex patterns and representations from data, making them powerful tools in various machine-learning tasks such as image recognition, natural language processing, and regression analysis.
44. What is the role of activation functions in neural networks?
Ans
Activation functions play a crucial role in neural networks by introducing non-linearity into the model. The non-linearity allows neural networks to learn complex relationships and patterns in data, making them capable of approximating highly non-linear functions. These activation functions provide a range of choices, and the selection depends on the characteristics of the data and the specific requirements of the neural network architecture. Experimentation and tuning are often necessary to determine the most effective activation function for a given task.
45. How do you deal with imbalanced datasets in machine learning?
Ans
Handling imbalanced datasets is crucial in machine learning, especially when the distribution of classes is uneven. Imbalanced datasets can lead models to bias toward the majority class, resulting in poor predictions for minority classes. It’s important to note that the choice of strategy depends on the specific characteristics of the dataset and the goals of the machine learning task. Additionally, experimentation and thorough evaluation are crucial to understanding the impact of different strategies on model performance.
46. What is the purpose of the term “epochs” in training neural networks?
Ans
In training neural networks, an “epoch” refers to one complete pass through the entire training dataset during the training phase. During each epoch, the model is trained on all available training samples, and the optimization algorithm updates the model’s parameters (weights and biases) based on the calculated gradients and the chosen optimization strategy.
47. Explain the difference between batch gradient descent, stochastic gradient descent, and mini-batch gradient descent. When would you use each?
Ans
- Batch Gradient Descent: In batch gradient descent, the entire training dataset is used to compute the gradient of the cost function with respect to the model parameters in a single iteration.
- Stochastic Gradient Descent (SGD): In stochastic gradient descent, a single randomly selected data point is used to compute the gradient of the cost function in each iteration. This process is repeated for each data point.
- Mini-Batch Gradient Descent: Mini-batch gradient descent strikes a balance between batch and stochastic gradient descent by using a randomly selected subset (mini-batch) of the training dataset to compute the gradient in each iteration.
48. Discuss the concept of transfer learning in neural networks. How can pre-trained models be used to improve performance in specific tasks?
Ans
Transfer learning is a machine learning technique where a model trained on one task is adapted for a different but related task. In the context of neural networks, transfer learning involves using a pre-trained model as a starting point for a new task rather than training a model from scratch. Transfer learning is a powerful technique that has significantly contributed to the success of deep learning models, especially in scenarios where labeled data for the target task is limited.
49. What is the concept of grid search in hyperparameter tuning? How does it help in optimizing machine learning models?
Ans
Grid search is a hyperparameter tuning technique to find the optimal combination of hyperparameter values for a machine learning model. Hyperparameters are external configurations set before the training process begins, significantly impacting a model’s performance. Grid search systematically evaluates different hyperparameter combinations from a predefined set, helping to identify the combination that yields the best model performance. Grid search helps in efficiently navigating the hyperparameter space, enabling the selection of optimal configurations that lead to improved model performance.
50. Explain the use of the term “dropout” in neural network architectures. How does it prevent overfitting?
Ans
Dropout is a regularization technique used in neural network architectures to prevent overfitting. It involves randomly “dropping out” (i.e., setting to zero) a fraction of the neurons during each training iteration. This process helps to create a more robust and generalized neural network by reducing reliance on specific neurons.
Dropout is a powerful regularization technique that has proven effective in improving the generalization ability of neural networks, especially in scenarios with limited training data or complex model architectures.
51. Describe the steps in building a machine learning pipeline using sci-kit-learn.
Ans
Building a machine learning pipeline using sci-kit-learn involves organizing and structuring the different stages of the machine learning process. A channel combines several data processing steps into a single object, making managing and reproducing the workflow easier. Here are the critical steps involved in building a machine learning pipeline using sci-kit-learn: you create a structured and reproducible machine learning pipeline using sci-kit-learn, which is essential for the efficient development and deployment of machine learning models.
52. What is the role of the confusion matrix in evaluating a classification model’s performance?
Ans
The confusion matrix is a tool used to assess the performance of a classification model by presenting a detailed breakdown of its predictions. It shows the number of true positives (correctly predicted positives), true negatives (correctly predicted negatives), false positives (actual negatives incorrectly predicted as positives), and false negatives (real positives incorrectly predicted as negatives). From these values, various metrics like accuracy, precision, recall, and F1 score can be derived, offering a comprehensive understanding of the model’s effectiveness in different aspects of classification.
53. Explain the concept of stratified sampling and its relevance in machine learning.
Ans
Stratified sampling is a technique where the population is divided into subgroups or strata based on specific characteristics, and samples are then randomly collected from each stratum. In machine learning, this technique ensures a representative distribution of classes or categories in both the training and testing datasets.
By maintaining the proportional representation of different classes within each stratum, stratified sampling helps prevent skewed datasets that might lead to biased model training. Applying stratified sampling in machine learning helps improve the model’s ability to generalize well across diverse classes and enhances its overall performance.
54. How can you assess the importance of features in a machine-learning model?
Ans
Another approach to assess the importance of features in a machine learning model is using SHAP (SHapley Additive explanations) values. SHAP values provide a way to allocate the contribution of each feature to the prediction across different instances. By analyzing these values, you can understand the impact of each element on individual predictions and overall model behavior. SHAP values offer a more nuanced and detailed perspective on feature importance, making them a valuable tool for model interpretation in various machine-learning scenarios.
55. What is the difference between L1 and L2 regularization in linear regression?
Ans
L1 and L2 regularization are techniques used in linear regression to prevent overfitting. Adding a penalty term to the regression equation regularization (Lasso) encourages sparsity, leading to feature selection by driving some coefficients to zero. L2 regularization (Ridge) penalizes large coefficients, promoting a more balanced distribution of feature impacts. The choice between L1 and L2 regularization depends on the data’s specific characteristics and the model’s desired properties.
56. Discuss the advantages and disadvantages of ensemble machine learning methods.
Ans
While ensemble methods offer significant advantages in terms of accuracy and robustness, they come with increased complexity and may require careful tuning. The choice to use ensemble methods should be based on the specific characteristics of the problem at hand and the trade-offs between accuracy, interpretability, and computational resources.
57. Explain the term “gradient boosting” and provide examples of popular gradient boosting algorithms.
Ans
Gradient Boosting is a machine learning technique that builds a robust predictive model by combining the predictions of multiple weak models, typically decision trees, sequentially. It works by fitting new models to the errors (residuals) of the existing ensemble, with each subsequent model focusing on areas where the choir performs poorly. The process continues iteratively, and the final prediction is the sum of the projections from all the individual models. Gradient boosting algorithms are robust and widely used in various machine learning applications due to their ability to create highly accurate predictive models. The choice of algorithm often depends on the specific characteristics of the dataset and the desired trade-offs in terms of speed, interpretability, and predictive performance.
58. Explain the concept of overfitting in machine learning. How can techniques like regularization help mitigate overfitting?
Ans
Overfitting occurs when a machine learning model learns the training data too well, capturing the underlying patterns and the noise or random fluctuations in the data. As a result, the model performs exceptionally well on the training set but fails to generalize to new, unseen data. Overfit models are highly complex and may capture idiosyncrasies specific to the training set, leading to poor performance on real-world data.
Regularization is a powerful tool to combat overfitting in machine learning by promoting simpler models and improving their generalization performance on unseen data. The choice between L1 and L2 regularization, as well as the appropriate regularization strength, depends on the data’s specific characteristics and the model’s desired properties.
59. What is the purpose of the ROC curve, and how is it used to evaluate the performance of a binary classification model?
Ans
The Receiver Operating Characteristic (ROC) curve is a graphical representation that illustrates the performance of a binary classification model at various classification thresholds. It plots the True Positive Rate (Sensitivity or Recall) against the False Positive Rate at different threshold settings, providing a comprehensive view of the trade-off between sensitivity and specificity. The ROC curve is a valuable tool for assessing the performance of binary classification models, especially when there is a need to understand the trade-offs between sensitivity and specificity at different classification thresholds.
60. Explain the concept of bagging. How does it work, and what is an example algorithm that uses bagging?
Ans
Bagging is an ensemble learning technique that aims to improve the stability and accuracy of machine learning models by combining multiple instances of the same model trained on different subsets of the training data. The idea is to introduce diversity among the models by teaching them on different subsets of the data, reducing the risk of overfitting and improving generalization. Bagging is a powerful technique for ensemble learning that builds diverse models using subsets of the data and combines their predictions to enhance overall performance and generality. Random Forest is a prominent example of a bagging algorithm, widely used for classification and regression tasks.
61. What is the purpose of the pickle module in Python, and how can it be used in the context of machine learning?
Ans
The pickle module in Python is used for serializing and deserializing Python objects. Serialization is the process of converting a Python object into a byte stream, and deserialization is the process of reconstructing the original object from a byte stream. This allows objects to be saved to a file, transferred over a network, or stored in a database.
It’s important to note that while pickle is convenient, it should be used with caution, especially when loading objects from untrusted sources, as it can execute arbitrary code during deserialization. For safer alternatives, consider using libraries like Joblib for model persistence in machine learning.
62. Explain the term “cross-entropy” and its significance in training neural networks.
Ans
Cross-entropy is a loss function commonly used in training neural networks, particularly in classification tasks. It quantifies the difference between the predicted probability distribution and the actual probability distribution of the classes in the training data. Cross-entropy is especially effective for models using a softmax activation function in the output layer, which is common in multi-class classification problems. Cross-entropy is a critical component in training neural networks, especially in classification tasks. Its mathematical properties and the way it penalizes incorrect predictions contribute to more effective learning, better calibration of predicted probabilities, and improved handling of imbalanced datasets.
63. What is the purpose of the train_test_split function in sci-kit-learn, and how is it used in the machine learning workflow?
Ans
The train_test_split function in scikit-learn is a utility for splitting a dataset into two subsets: a training set and a testing set. This function is commonly used in the machine learning workflow for evaluating the performance of a model. The primary purpose of train_test_split is to assess how well the model generalizes to new, unseen data by training it on one subset and testing it on another.train_test_split is a fundamental tool in the machine learning workflow for partitioning data, enabling effective model training and evaluation. It plays a crucial role in ensuring that machine learning models generalize well to new, unseen instances.
64. Describe the steps in implementing a simple linear regression model in Python using scikit-learn.
Ans
Implementing a simple linear regression model in Python using sci-kit-learn involves several steps, from loading the data to evaluating the trained model. This simple linear regression example demonstrates the basic steps in implementing and assessing a linear regression model using sci-kit-learn. Adjustments can be made based on the specifics of your dataset and problem.
65. What is the term “epoch” ‘s purpose in training neural networks, and how does it relate to batch size?
Ans
In the context of neural network training, an “epoch” refers to one complete pass through the entire training dataset during the training phase. During each epoch, the model sees and processes every training example once. The number of periods is a hyperparameter that determines how often the learning algorithm works through the entire training dataset. an epoch in neural network training represents one complete pass through the training dataset. The batch size determines how many training examples are processed in each iteration. The relationship between epochs and batch size affects the learning dynamics, convergence speed, and computational efficiency of the training process. The appropriate choice depends on the characteristics of the dataset and the goals of the training process.
66. What is the purpose of the NumPy and Pandas libraries in the context of machine learning in Python?
Ans
NumPy (Numerical Python): It supports large, multi-dimensional arrays and matrices, along with mathematical functions to operate on these arrays. NumPy is the foundation for many other scientific computing libraries in Python. In machine learning, it’s used for efficient numerical operations, array manipulations, and handling mathematical computations involved in algorithms.
Pandas: It offers high-level data structures like DataFrame, which is particularly useful for data manipulation and analysis. Pandas simplifies tasks such as cleaning, transforming, and organizing data. In machine learning, Pandas are often used for data preprocessing, exploratory data analysis (EDA), and handling structured data before feeding it into machine learning models.
67. How does k-fold cross-validation work, and why is it essential in machine learning?
Ans
K-fold cross-validation involves dividing a dataset into k subsets, using k-1 subsets for training and the remaining one for validation. This process is repeated k times, with each subgroup serving as the validation data exactly once. The average performance across all iterations is then used as the final model evaluation.
It’s important in machine learning to assess a model’s performance robustly. K-fold cross-validation helps by providing a more reliable estimate of how well a model will generalize to new, unseen data. It helps detect issues like overfitting or underfitting and ensures that the model’s performance is consistent across different subsets of the data.
68. Explain the bias-variance tradeoff. How does it relate to model complexity?
Ans
The bias-variance tradeoff is a fundamental concept in machine learning that involves finding the right balance between two sources of error: bias and variance. In relation to model complexity, as models become more complex, they tend to have lower bias but higher variance.
Simpler models, on the other hand, have higher bias but lower variance. The challenge is choosing a model complexity that strikes the right balance, avoiding underfitting and overfitting. Cross-validation and regularization are commonly used to help navigate and manage the bias-variance tradeoff during model training.
69. What is the role of activation functions in neural networks, and can you name a few commonly used activation functions in Python?
Ans
Activation functions play a crucial role in neural networks by introducing non-linearities, enabling it to learn complex relationships in the data. Without activation functions, a neural network would essentially be a linear model incapable of approximating more intricate patterns. Activation functions introduce non-linearities that allow neural networks to model complex relationships in the data, enabling them to learn and adapt to various patterns during training. The choice of activation function can impact the model’s performance and training dynamics, and experimentation is often done to find the most suitable function for a specific task.
70. Explain the term “one-hot encoding” and why it is necessary when working with categorical data.
Ans
One-hot encoding is a technique used to represent categorical variables as binary vectors. In this encoding, each category is represented by a binary vector, where all elements are zero except for the one corresponding to the category’s index. This is why it’s called “one-hot” – only one element is “hot” or set to 1one-hot encoding is a crucial preprocessing step when dealing with categorical data in machine learning, as it enables effective representation and utilization of such data in various algorithms.
71. How does regularization help prevent overfitting in machine learning models?
Ans
Regularization is a technique used in machine learning to prevent overfitting, where a model performs well on the training data but fails to generalize to new, unseen data. Overfitting occurs when a model is too complex and captures noise or specific patterns in the training data that do not represent the underlying relationships in the overall dataset. Regularization is a powerful tool to prevent overfitting by promoting simpler models and improving their generalization to new, unseen data. The choice of regularization strength (λ) is essential and often determined through cross-validation techniques.
72. What is the purpose of a confusion matrix, and how is it used to evaluate the performance of a classification model?
Ans
A confusion matrix is a table that is used to evaluate the performance of a classification model on a set of labeled data. It provides a detailed breakdown of the model’s predictions, highlighting the instances of correct and incorrect classifications. The matrix is particularly useful when dealing with binary or multiclass classification problems. Confusion matrices are valuable because they provide a more detailed understanding of a model’s performance beyond a single accuracy score. They help identify specific errors the model makes, such as false positives or false negatives, and can guide adjustments to the model or its threshold for making predictions. This nuanced evaluation is critical when considering the consequences of different errors in a given application.
73. Explain the concept of feature scaling. Why is it essential, and can you name different methods for scaling features in Python?
Ans
Feature scaling is a preprocessing step in machine learning that involves transforming the features of a dataset to a standardized range. The goal is to ensure that all features contribute equally to the model’s learning process, preventing some features from dominating due to their larger magnitudes.
Choosing the appropriate scaling method depends on the distribution of your data and the requirements of the machine learning algorithm you are using. Experimentation and understanding the characteristics of your data are essential in making informed decisions about feature scaling.
nd dimensionality reduction is beneficial for analysis and modeling.74. How would you handle a class imbalance in a binary classification problem?
Ans
Handling class imbalance in a binary classification problem is crucial to ensure that the machine learning model doesn’t favor the majority class and provides accurate predictions for both types. The choice of strategy depends on the specific characteristics of your dataset and the problem at hand. It’s often beneficial to experiment with multiple approaches and evaluate their impact on model performance using appropriate metrics for imbalanced datasets.
75. What is the purpose of grid search in hyperparameter tuning, and how is it implemented in Python?
Ans
Grid search is a technique used in hyperparameter tuning to systematically search through a predefined set of hyperparameter combinations to find the variety that yields the best model performance. The purpose is to automate the process of tuning hyperparameters and find the optimal values, saving time and effort compared to manual tuning. In this example, param_grid defines the hyperparameter grid, and GridSearchCV performs the grid search. The best hyperparameters are obtained using best_params_, and the final model is trained with these hyperparameters. Adjust the hyperparameter grid and scoring metric based on your specific problem and model.
76. What is the role of the pickle module in Python, and how can it be used in machine learning?
Ans
The pickle module in Python is used for serializing and deserializing Python objects. Serialization is the process of converting a Python object into a byte stream, and deserialization is the reverse process of reconstructing the original object from the byte stream. This module is particularly useful for saving and loading machine learning models, especially when you want to persistently store trained models or share them between different environments.
77. Describe the difference between batch gradient descent and stochastic gradient descent. When might you choose one over the other?
Ans
In practice, a compromise called Mini-Batch Gradient Descent is often used, where a small random subset of the data (a mini-batch) is used to compute the gradient at each iteration. Mini-batch SGD combines some advantages of both BGD and SGD, providing a good balance for many machine-learning scenarios.
Batch Gradient Descent (BGD):
- Update Rule: In BGD, the model parameters are updated based on the average gradient of the entire training dataset.
Stochastic Gradient Descent (SGD):
- Update Rule: In SGD, the model parameters are updated based on the gradient of a randomly chosen data point at each iteration.
78. How does Principal Component Analysis (PCA) work, and what is its significance in dimensionality reduction?
Ans
Principal Component Analysis (PCA) is a dimensionality reduction technique widely used in machine learning and data analysis. Its primary goal is to transform the original features of a dataset into a new set of uncorrelated variables called principal components, ordered by the amount of variance they explain. PCA is beneficial for reducing the dimensionality of a dataset while retaining as much information as possible.PCA is widely applied in various fields, such as image processing, pattern recognition, and bioinformatics, where high-dimensional data is common, and dimensionality reduction is beneficial for analysis and modeling.
79. Explain the concept of feature engineering. Can you provide examples of how feature engineering can improve model performance?
Ans
Feature engineering is creating new features or transforming existing ones in a dataset to improve a model’s performance. It involves selecting, combining, or transforming raw data to create informative and relevant features that enhance the model’s ability to learn patterns and make accurate predictions. Effective feature engineering requires a deep understanding of the data and the problem. It can significantly improve model performance by providing relevant information and facilitating the learning process for machine learning algorithms.
80. How do you address multicollinearity in a multiple linear regression model?
Ans
Multicollinearity occurs when two or more independent variables in a multiple linear regression model are highly correlated, making it challenging to isolate the individual effect of each variable on the dependent variable. This can lead to unstable coefficient estimates and increased standard errors. Addressing multicollinearity is crucial for obtaining reliable and interpretable results from the regression model. It’s important to note that the choice of strategy depends on the specific characteristics of the dataset and the goals of the analysis. Combining multiple approaches and iteratively refining the model can help effectively address multicollinearity.
81. What is the purpose of the matplotlib library in Python, and how can it be used to visualize data in machine learning?
Ans
Matplotlib is a widely used data visualization library in Python that provides a versatile set of tools for creating static, animated, and interactive visualizations in various formats. Its purpose is to enable the creation of high-quality charts, plots, and figures to visually explore and communicate patterns, trends, and relationships within data. In machine learning, Matplotlib is valuable for understanding data distributions, model performance, and other aspects of the analysis. Matplotlib is used to create a confusion matrix plot and display a classification report for a machine learning model. Visualizing such metrics is crucial for understanding the model’s performance, identifying improvement areas, and communicating results effectively. Matplotlib’s versatility makes it a powerful tool for creating a wide range of visualizations in machine-learning workflows
82. What is the difference between precision and recall?
Ans
Precision and recall are two metrics commonly used to evaluate the performance of a classification model, especially in the context of binary classification problems. precision and recall offer complementary perspectives on a classifier’s performance, and the choice between them depends on the specific goals and priorities of the application.
83. How do these metrics contribute to evaluating the performance of a classification model?
Ans
Precision, recall, and other related metrics comprehensively evaluate a classification model’s performance by providing insights into different aspects of its behavior. Evaluating a classification model involves considering multiple metrics to comprehensively understand its strengths and weaknesses. The choice of metrics depends on the nature of the problem, the importance of different types of errors, and the application’s goals.
84. How does early stopping work in training neural networks, and why might it be useful?
Ans
Early stopping is a regularization technique used in training neural networks to prevent overfitting and improve generalization. The basic idea is to monitor the model’s performance on a validation dataset during exercise and stop the training process when the performance starts to degrade, i.e., when the validation error stops decreasing or even increases.
In this example, training will stop if the validation loss does not improve for five consecutive epochs (patience=5). The restore_best_weights=True argument ensures that the model weights are restored to the best-performing consequences when training is stopped.
85. Describe the purpose of the pip tool in Python. How can it be used to manage dependencies in a machine-learning project?
Ans
The pip tool in Python is a package installer that simplifies installing, upgrading, and managing Python packages or libraries. It is a part of the Python Package Index (PyPI) ecosystem, a repository of Python packages and their metadata. The primary purpose of pip is to make it easy for developers to install and distribute Python packages. By leveraging pip and related practices, machine learning projects can maintain a well-defined and reproducible environment, making collaborating, sharing code, and deploying models across different systems easier.
86. What is the concept of data leakage in machine learning, and how can it be prevented?
Ans
Data leakage in machine learning refers to the situation where information from the training dataset is inadvertently used during the model training process in a way that would not be available when the model is applied to new, unseen data. This can lead to overly optimistic performance estimates during training but poor generalization when the model is deployed. By following these preventive measures, machine learning practitioners can minimize the risk of data leakage and build models that generalize well to new, unseen data. It’s essential to be vigilant and thoroughly understand the characteristics of the data to avoid unintentional mistakes that could compromise the integrity of the machine learning model.
87. What is the purpose of the term “feature scaling” in machine learning, and what methods can be used for feature scaling?
Ans
The purpose of feature scaling in machine learning is to standardize or normalize the range of independent variables or features of the dataset. This ensures that no component dominates the others, leading to a more balanced and effective learning process. Feature scaling is crucial for algorithms sensitive to the scale of input features, such as gradient-based methods. Choosing the appropriate scaling method depends on the characteristics of your data and the requirements of the machine learning algorithm you are using.
88. Explain the concept of ensemble learning. What are the advantages of ensemble methods, and provide examples of popular ensemble algorithms?
Ans
Ensemble learning is a machine learning paradigm that combines multiple models’ predictions to improve overall performance and generalization.
Advantages of Ensemble Methods:- Improved Accuracy: Ensemble methods can enhance predictive performance by reducing overfitting and capturing patterns that individual models might miss.
- Increased Robustness: Ensembles are less sensitive to noise and outliers since they consider multiple models, which can smooth out errors from individual models.
89. Describe the steps in preprocessing textual data for natural language processing (NLP) tasks.
Ans
Preprocessing textual data is a crucial step in natural language processing (NLP) tasks to ensure that the data is in a suitable format for analysis. The specific steps may vary based on the nature of the text data and the requirements of the NLP task at hand. Experimentation and understanding the characteristics of the data are essential to effective preprocessing.
90. What is the significance of the term “one-hot encoding” in handling categorical data?
Ans
One-hot encoding is a method used to represent categorical variables with a binary matrix, where a unique column represents each category, and each observation is marked with a 1 in the column corresponding to its category. This encoding is crucial when working with machine learning algorithms that require numerical input, as it transforms categorical data into a format that can be easily processed by these algorithms. one-hot encoding is a vital technique in handling categorical data, ensuring that the information is represented in a way that is both meaningful to the model and compatible with various machine learning algorithms.
91. Explain the concept of kernel functions in support vector machines (SVMs). How do different kernel functions impact the model’s performance?
Ans
Kernel functions in support vector machines (SVMs) are crucial in transforming input data into a higher-dimensional space, where the data becomes more separable. SVMs use these kernel functions to efficiently compute the dot product of data points in this higher-dimensional space without explicitly calculating the transformation. This allows SVMs to find complex decision boundaries in the original feature space.
Kernel functions in SVMs enable the modeling of non-linear relationships by implicitly mapping data into higher-dimensional spaces. The selection of an appropriate kernel is essential for achieving good performance, and it depends on the nature of the data and the problem being solved. Proper hyperparameter tuning is also critical for optimizing the SVM’s performance with different kernel functions.
92. What is dimensionality reduction, and why is it important in machine learning?
Ans
Dimensionality reduction is the process of reducing the number of features or variables in a dataset while retaining its essential information. The primary goal is to simplify the dataset’s representation, making it more manageable, interpretable, and computationally efficient without losing significant information. This is particularly important in machine learning for several reasons: Choosing the appropriate dimensionality reduction technique depends on factors such as the nature of the data, the desired interpretability, and the specific goals of the machine learning task.
93. Explain the concept of stratified sampling and its importance in creating representative training and testing datasets.
Ans
Stratified sampling is a sampling technique used in statistics and machine learning to ensure that each subgroup or stratum within a population is adequately represented in the sample. In the context of creating training and testing datasets, stratified sampling is particularly important to maintain the distribution of classes or categories, ensuring that the sample is representative of the overall population.stratified sampling is a valuable technique in machine learning to create representative training and testing datasets, especially when dealing with imbalanced class distributions. It helps mitigate biases, ensures statistical significance, and contributes to the overall robustness and fairness of machine learning models.
94. How does the area under the precision-recall curve (AUC-PR) differ from the AUC-ROC, and in what scenarios would you prefer one over the other?
Ans
The area under the precision-recall curve (AUC-PR) measures the area under the curve formed by plotting precision against recall, while the AUC-ROC (Receiver Operating Characteristic) measures the area under the curve formed by plotting true positive rate (sensitivity) against false positive rate.
AUC-PR is often preferred in imbalanced datasets where one class significantly outnumbers the other, as it focuses on the performance of a classifier in correctly identifying the positive class. It’s particularly relevant in scenarios where false positives are more critical than false negatives, such as in medical diagnoses.
95. Describe the concept of transfer learning in the context of deep learning. How can pre-trained models be beneficial in building new models for specific tasks?
Ans
Transfer learning in deep learning involves leveraging knowledge gained from solving one problem and applying it to a different but related problem. Pre-trained models are neural networks that have been trained on large datasets for a specific task, often a more general one like image recognition or language understanding. For example, a pre-trained convolutional neural network (CNN) for image classification can be fine-tuned for a specific task like recognizing different dog breeds. The lower layers of the network, which capture generic features like edges and textures, can be kept fixed, while the upper layers are adapted to the new task.
In summary, transfer learning allows the reuse of knowledge from pre-trained models, providing a head start for new tasks and addressing challenges such as limited data and computational resources.
96. What is the purpose of grid search in hyperparameter tuning, and how is it implemented in Python?
Ans
Grid search is a hyperparameter tuning technique used to systematically search through a predefined set of hyperparameter values to find the combination that yields the best model performance. The purpose is to identify the optimal hyperparameters for a machine learning algorithm, improving its predictive accuracy.
This example uses a RandomForestClassifier, but you can replace it with the model of your choice. The param_grid dictionary defines the hyperparameters and their possible values. The GridSearchCV object then performs cross-validated grid search to find the best combination of hyperparameters. Finally, you can access the best parameters and the best model for further evaluation.
97. Explain the concept of early stopping in neural network training. Why is it used, and how does it help prevent overfitting?
Ans
Early stopping is a regularization technique used during the training of neural networks to prevent overfitting. The idea is to monitor the model’s performance on a validation dataset during training and stop the training process once the performance stops improving or starts degrading.To implement early stopping in neural networks, you can use callbacks in deep learning frameworks like TensorFlow or PyTorch. These callbacks allow you to monitor the validation performance and stop training when necessary.
98. What is the curse of dimensionality in machine learning? How does it affect model performance?
Ans
The curse of dimensionality refers to the challenges and issues that arise when dealing with high-dimensional data in machine learning. As the number of features or dimensions in a dataset increases, the volume of the feature space grows exponentially. This phenomenon has several implications that can impact model performance.
To address the curse of dimensionality, techniques such as feature selection, dimensionality reduction (e.g., PCA), and careful consideration of model complexity are commonly employed. These methods aim to retain essential information while reducing the negative impact of high dimensionality on model performance.
99. Explain the concept of transfer learning in the context of deep neural networks. How can pre-trained models be utilized?
Ans
Transfer learning in the context of deep neural networks involves leveraging knowledge gained from solving one problem and applying it to a different but related problem. This is particularly useful when working with deep learning models that have millions of parameters and require substantial amounts of labeled data for training.Pre-trained models, often available in deep learning libraries like TensorFlow or PyTorch, can be utilized by loading the pre-trained weights into the model architecture. These pre-trained models are typically trained on large datasets for tasks like image classification (e.g., ImageNet) or natural language processing (e.g., Word2Vec, BERT). Fine-tuning involves adjusting the model’s parameters on the specific target task, ensuring that the model becomes well-suited to the nuances of the new problem.