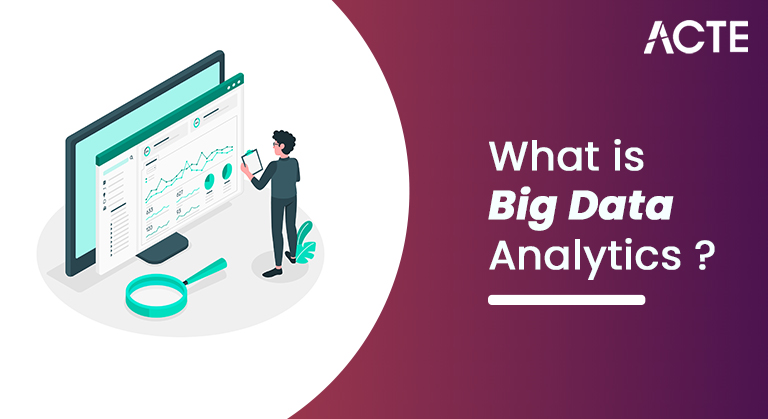
Big data analytics is the use of advanced analytic techniques against very large, diverse big data sets that include structured, semi-structured and unstructured data, from different sources, and in different sizes from terabytes to zettabytes.
- What is Big Data Analytics
- Big Data analytics equipment and era
- Characteristics Of Big Data Analytics
- Different Types of Big Data Analytics
- Example of Big Data Analytics:
- How Big data analytics works
- Why is Big data analytics vital
- Latest Trends in Big Data Analytics
- The massive advantages of Big data analytics
- Conclusion
What is Big Data Analytics
Big data analytics describes the manner of uncovering traits, styles, and correlations in big quantities of uncooked data to assist make statistics-knowledgeable selections. These strategies use acquainted statistical evaluation strategies—like clustering and regression—and observe them to greater vast datasets with the assist of more recent equipment. Big data has been a buzz phrase for the reason that early 2000s, whilst software program and hardware abilities made it viable for groups to address big quantities of unstructured statistics.
Since then, new technology—from Amazon to smartphones—have contributed even greater to the full-size quantities of datato be had to groups. With the explosion of statistics, early innovation tasks like Hadoop, Spark, and NoSQL databases have been created for the garage and processing of massive statistics. This area keeps to conform as dataengineers search for methods to combine the massive quantities of complicated records created with the aid of using sensors, networks, transactions, clever gadgets, net usage, and greater. Even now, massive dataanalytics techniques are getting used with rising technology, like gadget getting to know, to find out and scale greater complicated insights.
Big Data analytics equipment and era
Big data analytics can’t be narrowed right all the way down to a unmarried device or era. Instead, numerous sorts of equipment paintings collectively that will help you collect, manner, cleanse, and examine massive statistics. Some of the fundamental gamers in massive data ecosystems are indexed below.
Hadoop is an open-supply framework that effectively shops and strategies massive datasets on clusters of commodity hardware. This framework is unfastened and might manage big quantities of dependent and unstructured statistics, making it a precious mainstay for any massive data operation.
NoSQL databases are non-relational data control structures that don’t require a hard and fast scheme, making them a first-rate alternative for massive, uncooked, unstructured statistics. NoSQL stands for “now no longer most effective SQL,” and those databases can manage quite a few data models.
MapReduce is an important issue to the Hadoop framework serving functions. The first is mapping, which filters data to diverse nodes in the cluster. The 2d is lowering, which organizes and decreases the outcomes from every node to reply a query. YARN stands for “Yet Another Resource Negotiator.” It is every other issue of 2d-era Hadoop. The cluster control era facilitates with process scheduling and aid control in the cluster.
Spark is an open supply cluster computing framework that makes use of implicit data parallelism and fault tolerance to offer an interface for programming complete clusters. Spark can manage each batch and circulate processing for immediate computation.
Tableau is an cease-to-cease data analytics platform that permits you to prep, examine, collaborate, and proportion your massive data insights. Tableau excels in self-carrier visible evaluation, permitting humans to invite new questions of ruled massive data and without problems proportion the ones insights throughout the organization.
- Volume
- Variety
- Velocity
- Variability
Characteristics Of Big Data Analytics
Big data may be defined with the aid of using the subsequent characteristics:
(i) Volume – The call Big Data itself is associated with a length that is enormous. Size of data performs a totally essential function in figuring out fee out of statistics. Also, whether or not a specific data can without a doubt be taken into consideration as a Big Data or now no longer, depends upon the extent of statistics. Hence, ‘Volume’ is one feature which wishes to be taken into consideration even as coping with Big Data answers.
(ii) Variety – The subsequent component of Big Data is its variety. Variety refers to heterogeneous reassets and the character of statistics, each dependent and unstructured. During in advance days, spreadsheets and databases have been the most effective reassets of datataken into consideration with the aid of using maximum of the packages. Nowadays, data withinside the shape of emails, photos, videos, tracking gadgets, PDFs, audio, etc. also are being taken into consideration withinside the evaluation packages. This sort of unstructured data poses positive problems for garage, mining and studying statistics.
(iii) Velocity – The term ‘velocity’ refers to the velocity of era of statistics. How rapid the data is generated and processed to fulfill the demands, determines actual capacity withinside the statistics.
Big Data Velocity offers with the velocity at which dataflows in from reassets like commercial enterprise strategies, software logs, networks, and social media sites, sensors, Mobile gadgets, etc. The go with the drift of data is big and continuous.
(iv) Variability – This refers back to the inconsistency which may be proven with the aid of using the data at times, for that reason hampering the manner of being capable of manage and manipulate the data effectively.
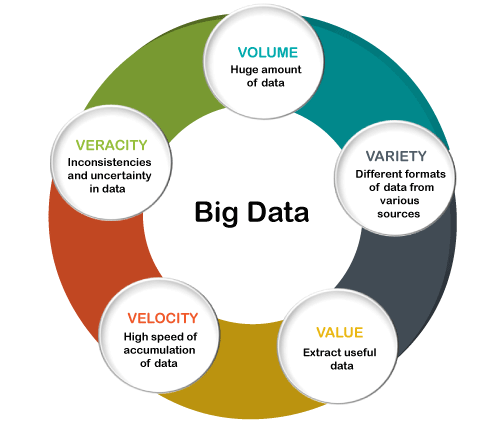
Different Types of Big Data Analytics
Here are the 4 sorts of Big Data analytics:
1. Descriptive Analytics
This summarizes beyond data right into a shape that humans can without problems read. This facilitates in growing reports, like a employer’s revenue, profit, sales, and so on. Also, it facilitates withinside the tabulation of social media metrics.
2. Diagnostic Analytics
This is performed to apprehend what induced a hassle withinside the first place. Techniques like drill-down, datamining, and data healing are all examples. Organizations use diagnostic analytics due to the fact they offer an in-intensity perception into a specific hassle.
3. Predictive Analytics
This sort of analytics seems into the ancient and gift data to make predictions of destiny. Predictive analytics makes use of datamining, AI, and gadget getting to know to research modern data and make predictions approximately destiny. It works on predicting consumer traits, marketplace traits, and so on.
4. Prescriptive Analytics
This sort of analytics prescribes the answer to a specific hassle. Perspective analytics works with each descriptive and predictive analytics. Most of the time, it is predicated on AI and gadgets getting to know.
Example of Big Data Analytics:
Following are a number of the Big Data examples-
The New York Stock Exchange is an instance of Big Data that generates approximately one terabyte of recent exchange data consistent with day.
Social Media: The statistic indicates that 500+terabytes of recent data get ingested into the databases of social media web website online Facebook, each day. This data is specially generated in phrases of picture graph and video uploads, message exchanges, setting remarks etc.
An unmarried Jet engine can generate 10+terabytes of data in half-hour of flight time. With many thousand flights consistent with day, the era of data reaches as much as many Petabytes.
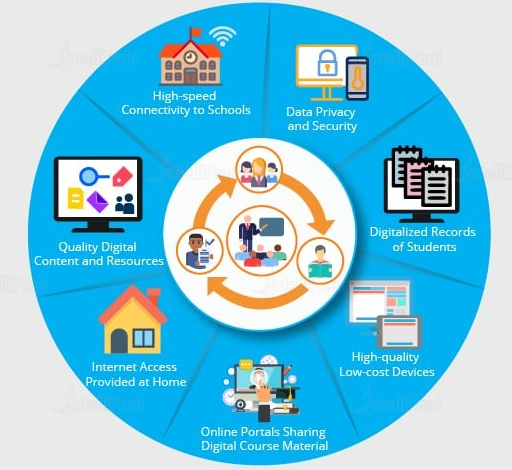
- Data mining types via big datasets to become aware of styles and relationships with the aid of using figuring out anomalies and growing data clusters.
- Predictive analytics makes use of an organization’s ancient data to make predictions approximately the destiny, figuring out upcoming dangers and possibilities.
- Deep getting to know imitates human getting to know styles with the aid of using the use of synthetic intelligence and gadget getting to know to layer algorithms and locate styles withinside the maximum complicated and summary statistics.
How massive data analytics works
Big data analytics refers to accumulating, processing, cleaning, and studying big datasets to assist groups operationalize their massive statistics.
1. Collect Data
Data series seems distinct for each organization. With today’s era, groups can collect each dependent and unstructured data from quite a few reassets — from cloud garage to cell packages to in-shop IoT sensors and beyond. Some data can be saved in data warehouses in which commercial enterprise intelligence equipment and answers can get entry to it without problems. Raw or unstructured data this is too numerous or complicated for a warehouse can be assigned metadata and saved in a data lake.
2. Process Data
Once data is gathered and saved, it have to be prepared nicely to get correct outcomes on analytical queries, specifically whilst it’s big and unstructured. Available data is developing exponentially, making data processing a assignment for groups. One processing alternative is batch processing, which seems at big data blocks over time. Batch processing is beneficial whilst there may be an extended turnaround time among accumulating and studying statistics. Stream processing seems at small batches of data right now, shortening the postpone time among series and evaluation for faster choice-making. Stream processing is greater complicated and frequently greater expensive.
3. Clean Data
Data massive or small calls for scrubbing to enhance data exceptional and get more potent outcomes; all data have to be formatted correctly, and any duplicative or inappropriate data have to be removed or accounted for. Dirty data can difficult to understand and mislead, growing incorrect insights.
4. Analyze Data
Getting massive data right into a usable nation takes time. Once it’s ready, superior analytics strategies can flip massive data into massive insights. Some of those massive data evaluation techniques include:
Why is massive data analytics vital
Big data analytics facilitates groups harness their data and use it to become aware of new possibilities. That, in flip, results in smarter commercial enterprise moves, greater green operations, better earnings and happier clients. In his file Big Data in Big Companies, IIA Director of Research Tom Davenport interviewed greater than 50 agencies to apprehend how they used massive statistics. He discovered they were given fee withinside the following methods:
Cost reduction Big data technology which include Hadoop and cloud-primarily based totally analytics deliver tremendous fee blessings in terms of storing big quantities of data– plus they could become aware of greater green methods of doing commercial enterprise.
New merchandise and services With the capacity to gauge consumer wishes and pride via analytics comes the strength to offer clients what they need. Davenport factors out that with massive data analytics, greater corporations are growing new merchandise to fulfill clients’ wishes.
Latest Trends in Big Data Analytics
You can be amazed with the aid of using the reality that every day we’re generating greater data in 2 days than a long time of history. Yes, that’s true, and maximum people do now no longer even realize this component that we produce a lot data simply with the aid of using surfing at the Internet. If you don’t need the destiny technology to seize you off guard, take note of those modern traits in massive data analytics and succeed!
1. Data as carrierTraditionally the Data is saved in data shops, evolved to attain with the aid of using unique packages. When the SaaS (software program as a carrier) turned into popular, Daas turned into only a beginning. As with Software-as-a-Service packages, Data as a carrier makes use of cloud era to offer customers and packages with on-call for get entry to to records with out relying on in which the customers or packages can be.
Data as a Service is one of the modern traits in massive data analytics and could supply it less complicated for analysts to attain data for commercial enterprise overview responsibilities and less difficult for regions for the duration of a commercial enterprise or enterprise to proportion statistics.
2. Responsible and Smarter Artificial Intelligence
Responsible and Scalable AI will allow higher getting to know algorithms with shorter time to marketplace. Businesses will reap loads greater from AI structures like formulating strategies that could characteristic effectively. Businesses will discover a manner to take AI to scale, which has been a first-rate assignment until now.
3. Predictive Analytics
Big data analytics has constantly been a essential method for corporations to turn out to be a competing part and achieve their aims. They observe primary analytics equipment to put together massive data and find out the reasons of why unique problems arise. Predictive techniques are applied to study contemporary-day data and ancient activities to understand clients and understand viable risks and activities for a corporation.
Predictive evaluation in massive data can are expecting what can also additionally arise withinside the destiny. This method is extraordinarily green in correcting analyzed assembled data to are expecting consumer response. This permits groups to outline the stairs they should exercise with the aid of using figuring out a consumer’s subsequent flow earlier than they even do it.
4. Quantum Computing
Using modern time era can take a whole lot of time to manner a massive quantity of statistics. Whereas, Quantum computer systems, calculate the possibility of an object’s nation or an occasion earlier than it’s miles measured, which suggests that it is able to manner greater data than classical computer systems.
If most effective we compress billions of data right now in just a few minutes, we are able to lessen processing length immensely, presenting groups the opportunity to benefit well timed selections to achieve greater aspired outcomes. This manner may be viable the use of Quantum computing. The test of quantum computer systems to accurate purposeful and analytical studies over numerous corporations could make the enterprise greater precise.
5. Edge Computing
Running strategies and transferring the ones strategies to a neighborhood machine which include any user’s machine or IoT tool or a server defines Edge Processing. Edge computing brings computation to a network’s part and decreases the quantity of long-distance connection that has to show up among a consumer and a server, that is making it the modern traits in massive data analytics. It offers a lift to Data Streaming, which include actual-time data Streaming and processing with out containing latency. It permits the gadgets to reply immediately. Edge computing is an effective manner to manner big datawith the aid of using ingesting much less bandwidth usage.
6. Natural Language Processing
Natural Language Processing( NLP) lies internal synthetic intelligence and works to broaden conversation among computer systems and humans. The goal of NLP is to read, decode the which means of the human language. Natural language processing is ordinarily primarily based totally on gadget getting to know, and it’s miles used to broaden phrase processor packages or translating software program. Natural Language Processing Techniques want algorithms to understand and attain the desired data from every sentence with the aid of using making use of grammar rules.
Mostly syntactic evaluation and semantic evaluation are the strategies which are utilized in herbal language processing. Syntactic evaluation is the only that handles sentences and grammatical problems, while semantic evaluation handles the which means of the statistics/text.
7. Hybrid Clouds
A cloud computing machine makes use of an on-premises personal cloud and a 3rd birthday birthday celebration public cloud with orchestration among interfaces. Hybrid cloud offers tremendous flexibility and greater data deployment alternatives with the aid of using transferring the strategies among personal and public clouds. An organization have to have a personal cloud to benefit adaptability with the aspired public cloud.
For that, it has to broaden a datacenter, which include servers, garage, LAN, and cargo balancer. The organization has to set a virtualization layer/hypervisor to help the VMs and containers. And, deployation a personal cloud software program layer. The implementation of software program permits times to switch data among the personal and public clouds.
8. Data Fabric
Data cloth is an structure and series of data networks. That offers steady capability throughout quite a few endpoints, each on-premises and cloud environments. To force virtual transformation, Data Fabric simplifies and contains data garage throughout cloud and on-premises environments. It permits get entry to and sharing of data in a allotted data environment. Additionally offers steady data control framework throughout un-siloed garage.
9. XOps
The purpose of XOps (statistics, ML, model, platform) is to reap efficiencies and economies of scale. XOps is completed with the aid of using enforcing DevOps first-class practices. Thus, making sure performance, reusability, and repeatability even as lowering era, manner replication and permitting automation. These improvements could allow prototypes to be scaled, with bendy layout and agile orchestration of ruled structures.The massive advantages of massive data analytics
The capacity to research greater data at a quicker charge can offer massive advantages to an organization, permitting it to greater effectively use data to reply vital questions. Big data analytics is vital as it we could groups use enormous quantities of data in more than one codecs from more than one reassets to become aware of possibilities and dangers, assisting groups flow fast and enhance their backside lines. Some advantages of massive data analytics include:
Cost financial savings. Helping groups become aware of methods to do commercial enterprise greater effectively
Product development. Providing a higher knowledge of consumer wishes Market insights. Tracking buy conduct and marketplace traits.
Conclusion
Big Data is a game-changer. Many groups are the use of greater analytics to force strategic movements and provide a higher consumer experience. A mild trade in the performance or smallest financial savings can result in a massive profit, that is why maximum groups are transferring toward massive statistics.