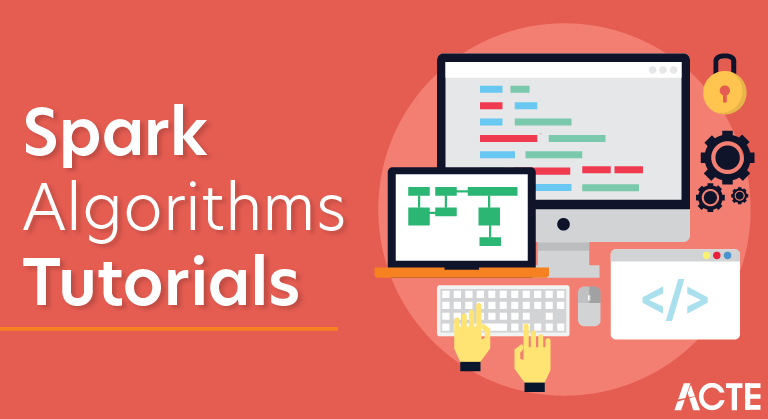
Spark Programming is nothing but a general-purpose & lightning fast cluster computing platform. In other words, it is an open source, wide range data processing engine. That reveals development API’s, which also qualifies data workers to accomplish streaming, machine learning or SQL workloads which demand repeated access to data sets. However, Spark can perform batch processing and stream processing. Batch processing refers, to the processing of the previously collected job in a single batch. Whereas stream processing means to deal with Spark streaming data.
Moreover, it is designed in such a way that it integrates with all the Big data tools. Like spark can access any Hadoop data source, also can run on Hadoop clusters. Furthermore, Apache Spark extends Hadoop MapReduce to the next level. That also includes iterative queries and stream processing.
- One more common belief about Spark is that it is an extension of Hadoop. Although that is not true. However, Spark is independent of Hadoop since it has its own cluster management system. Basically, it uses Hadoop for storage purpose only.
- Although, there is one spark’s key feature that it has in-memory cluster computation capability. Also increases the processing speed of an application.
- Basically, Apache Spark offers high-level APIs to users, such as Java, Scala, Python, and R. Although, Spark is written in Scala still offers rich APIs in Scala, Java, Python, as well as R. We can say, it is a tool for running spark applications.
Most importantly, by comparing Spark with Hadoop, it is 100 times faster than Hadoop In-Memory mode and 10 times faster than Hadoop On-Disk mode.
Why Spark?
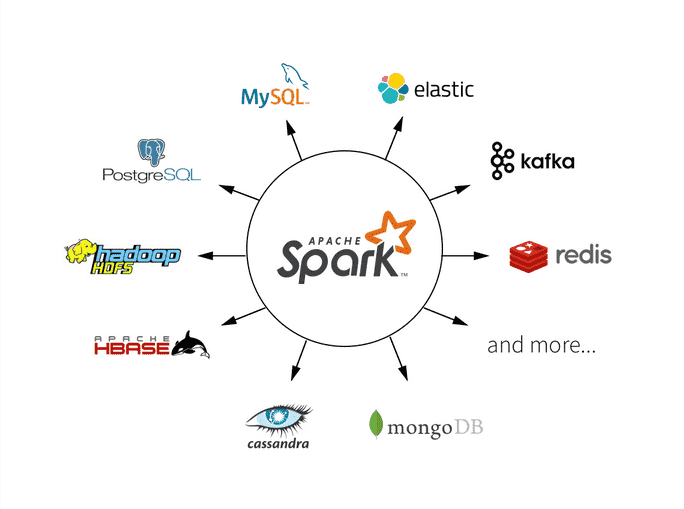
As we know, there was no general purpose computing engine in the industry, since
- To perform batch processing, we were using Hadoop MapReduce.
- Also, to perform stream processing, we were using Apache Storm / S4.
- Moreover, for interactive processing, we were using Apache Impala / Apache Tez.
- To perform graph processing, we were using Neo4j / Apache Giraph.
Hence there was no powerful engine in the industry, that can process the data both in real-time and batch mode. Also, there was a requirement that one engine can respond in sub-second and perform in-memory processing.
Therefore, Apache Spark programming enters, it is a powerful open source engine. Since, it offers real-time stream processing, interactive processing, graph processing, in-memory processing as well as batch processing. Even with very fast speed, ease of use and standard interface. Basically, these features create the difference between Hadoop and Spark. Also makes a huge comparison between Spark vs Storm.
Features of Apache Spark
There are several sparkling Apache Spark features:
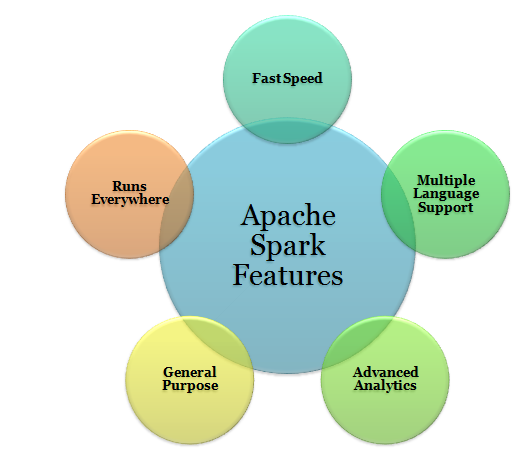
a. Swift Processing
Apache Spark offers high data processing speed. That is about 100x faster in memory and 10x faster on the disk. However, it is only possible by reducing the number of read-write to disk.
b. Dynamic in Nature
Basically, it is possible to develop a parallel application in Spark. Since there are 80 high-level operators available in Apache Spark.
c. In-Memory Computation in Spark
The increase in processing speed is possible due to in-memory processing. It enhances the processing speed.
d. Reusability
We can easily reuse spark code for batch-processing or join stream against historical data. Also to run ad-hoc queries on stream state.
e. Spark Fault Tolerance
Spark offers fault tolerance. It is possible through Spark’s core abstraction-RDD. Basically, to handle the failure of any worker node in the cluster, Spark RDDs are designed. Therefore, the loss of data is reduced to zero.
f. Real-Time Stream Processing
We can do real-time stream processing in Spark. Basically, Hadoop does not support real-time processing. It can only process data which is already present. Hence with Spark Streaming, we can solve this problem.
g. Lazy Evaluation in Spark
All the transformations we make in Spark RDD are Lazy in nature, that is it does not give the result right away rather a new RDD is formed from the existing one. Thus, this increases the efficiency of the system.
h. Support Multiple Languages
Spark supports multiple languages. Such as Java, R, Scala, Python. Hence, it shows dynamicity. Moreover, it also overcomes the limitations of Hadoop since it can only build applications in Java.
i. Support for Sophisticated Analysis
There are dedicated tools in Apache Spark. Such as for streaming data interactive/declarative queries, machine learning which add-on to map and reduce.
j. Integrated with Hadoop
As we know Spark is flexible. It can run independently and also on Hadoop YARN Cluster Manager. Even it can read existing Hadoop data.
k. Spark GraphX
In Spark, a component for graph and graph-parallel computation, we have GraphX. Basically, it simplifies the graph analytics tasks by the collection of graph algorithm and builders.
l. Cost Efficient
For Big data problem as in Hadoop, a large amount of storage and the large data center is required during replication. Hence, Spark programming turns out to be a cost-effective solution.
Features of Apache Spark
Let’s discuss sparkling features of Apache Spark:
a. Swift Processing
Using Apache Spark, we achieve a high data processing speed of about 100x faster in memory and 10x faster on the disk. This is made possible by reducing the number of read-write to disk.
b. Dynamic in Nature
We can easily develop a parallel application, as Spark provides 80 high-level operators.
c. In-Memory Computation in Spark
With in-memory processing, we can increase the processing speed. Here the data is being cached so we need not fetch data from the disk every time thus the time is saved. Spark has DAG execution engine which facilitates in-memory computation and acyclic data flow resulting in high speed.
d. Reusability
we can reuse the Spark code for batch-processing, join stream against historical data or run ad-hoc queries on stream state.
e. Fault Tolerance in Spark
Apache Spark provides fault tolerance through Spark abstraction-RDD. Spark RDDs are designed to handle the failure of any worker node in the cluster. Thus, it ensures that the loss of data reduces to zero. Learn different ways to create RDD in Apache Spark.
f. Real-Time Stream Processing
Spark has a provision for real-time stream processing. Earlier the problem with Hadoop MapReduce was that it can handle and process data which is already present, but not the real-time data. but with Spark Streaming we can solve this problem.
g. Lazy Evaluation in Apache Spark
All the transformations we make in Spark RDD are Lazy in nature, that is it does not give the result right away rather a new RDD is formed from the existing one. Thus, this increases the efficiency of the system. Follow this guide to learn more about Spark Lazy Evaluation in great detail.
h. Support Multiple Languages
In Spark, there is Support for multiple languages like Java, R, Scala, Python. Thus, it provides dynamicity and overcomes the limitation of Hadoop that it can build applications only in Java.
Get the best Scala Books To become an expert in Scala programming language.
i. Active, Progressive and Expanding Spark Community
Developers from over 50 companies were involved in making of Apache Spark. This project was initiated in the year 2009 and is still expanding and now there are about 250 developers who contributed to its expansion. It is the most important project of Apache Community.
j. Support for Sophisticated Analysis
Spark comes with dedicated tools for streaming data, interactive/declarative queries, machine learning which add-on to map and reduce.
k. Integrated with Hadoop
Spark can run independently and also on Hadoop YARN Cluster Manager and thus it can read existing Hadoop data. Thus, Spark is flexible.
l. Spark GraphX
Spark has GraphX, which is a component for graph and graph-parallel computation. It simplifies the graph analytics tasks by the collection of graph algorithm and builders.
m. Cost Efficient
Apache Spark is cost effective solution for Big data problem as in Hadoop large amount of storage and the large data center is required during replication.
Spark Tutorial – Spark Streaming
While data is arriving continuously in an unbounded sequence is what we call a data stream. Basically, for further processing, Streaming divides continuous flowing input data into discrete units. Moreover, we can say it is a low latency processing and analyzing of streaming data.
In addition, an extension of the core Spark API Streaming was added to Apache Spark in 2013. That offers scalable, fault-tolerant and high-throughput processing of live data streams. Although, here we can do data ingestion from many sources. Such as Kafka, Apache Flume, Amazon Kinesis or TCP sockets. However, we do processing here by using complex algorithms which are expressed with high-level functions such as map, reduce, join and window.
a. Internal working of Spark Streaming
Let’s understand its internal working. While live input data streams are received. It further divided into batches by Spark streaming, Afterwards, these batches are processed by the Spark engine to generate the final stream of results in batches.
b. Discretized Stream (DStream)
Apache Spark Discretized Stream is the key abstraction of Spark Streaming. That is what we call Spark DStream. Basically, it represents a stream of data divided into small batches. Moreover, DStreams are built on Spark RDDs, Spark’s core data abstraction. It also allows Streaming to seamlessly integrate with any other Apache Spark components. Such as Spark MLlib and Spark SQL.
Benefits of Apache Spark:
- Speed
- Ease of Use
- Advanced Analytics
- Dynamic in Nature
- Multilingual
- Apache Spark is powerful
- Increased access to Big data
- Demand for Spark Developers
- Open-source community
1. Speed:
When comes to Big Data, processing speed always matters. Apache Spark is wildly popular with data scientists because of its speed. Spark is 100x faster than Hadoop for large scale data processing. Apache Spark uses in-memory(RAM) computing system whereas Hadoop uses local memory space to store data. Spark can handle multiple petabytes of clustered data of more than 8000 nodes at a time.
2. Ease of Use:
Apache Spark carries easy-to-use APIs for operating on large datasets. It offers over 80 high-level operators that make it easy to build parallel apps.
3. Advanced Analytics:
Spark not only supports ‘MAP’ and ‘reduce’. It also supports Machine learning (ML), Graph algorithms, Streaming data, SQL queries, etc.
4. Dynamic in Nature:
With Apache Spark, you can easily develop parallel applications. Spark offers you over 80 high-level operators.
5. Multilingual:
Apache Spark supports many languages for code writing such as Python, Java, Scala, etc.
6. Apache Spark is powerful:
Apache Spark can handle many analytics challenges because of its low-latency in-memory data processing capability. It has well-built libraries for graph analytics algorithms and machine learning.
7. Increased access to Big data:
Apache Spark is opening up various opportunities for big data and making As per the recent survey conducted by IBM’s announced that it will educate more than 1 million data engineers and data scientists on Apache Spark.
8. Demand for Spark Developers:
Apache Spark not only benefits your organization but you as well. Spark developers are so in-demand that companies offering attractive benefits and providing flexible work timings just to hire experts skilled in Apache Spark. As per PayScale the average salary for Data Engineer with Apache Spark skills is $100,362. For people who want to make a career in the big data, technology can learn Apache Spark. You will find various ways to bridge the skills gap for getting data-related jobs, but the best way is to take formal training which will provide you hands-on work experience and also learn through hands-on projects.
9. Open-source community:
The best thing about Apache Spark is, it has a massive Open-source community behind it.
Limitations of Apache Spark
As we know Apache Spark is the next Gen Big data tool that is being widely used by industries but there are certain limitations of Apache Spark due to which industries have started shifting to Apache Flink– 4G of Big Data. Before we learn what are the disadvantages of Apache Spark, let us learn the advantages of Apache Spark.
a. No Support for Real-time Processing
In Spark Streaming, the arriving live stream of data is divided into batches of the pre-defined interval, and each batch of data is treated like Spark Resilient Distributed Database (RDDs). Then these RDDs are processed using the operations like map, reduce, join etc. The result of these operations is returned in batches. Thus, it is not real time processing but Spark is near real-time processing of live data. Micro-batch processing takes place in Spark Streaming.
b. Problem with Small File
If we use Spark with Hadoop, we come across a problem of a small file. HDFS provides a limited number of large files rather than a large number of small files. Another place where Spark legs behind is we store the data gzipped in S3. This pattern is very nice except when there are lots of small gzipped files. Now the work of the Spark is to keep those files on network and uncompress them. The gzipped files can be uncompressed only if the entire file is on one core. So a large span of time will be spent in burning their core unzipping files in sequence.
In the resulting RDD, each file will become a partition; hence there will be a large amount of tiny partition within an RDD. Now if we want efficiency in our processing, the RDDs should be repartitioned into some manageable format. This requires extensive shuffling over the network.
c. No File Management System
Apache Spark does not have its own file management system, thus it relies on some other platform like Hadoop or another cloud-based platform which is one of the Spark known issues.
d. Expensive
In-memory capability can become a bottleneck when we want cost-efficient processing of big data as keeping data in memory is quite expensive, the memory consumption is very high, and it is not handled in a user-friendly manner. Apache Spark requires lots of RAM to run in-memory, thus the cost of Spark is quite high.
e. Less number of Algorithms
Spark MLlib lags behind in terms of a number of available algorithms like Tanimoto distance.
f. Manual Optimization
The Spark job requires to be manually optimized and is adequate to specific datasets. If we want to partition and cache in Spark to be correct, it should be controlled manually.
g. Iterative Processing
In Spark, the data iterates in batches and each iteration is scheduled and executed separately.
h. Latency
Apache Spark has higher latency as compared to Apache Flink.
i. Window Criteria
Spark does not support record based window criteria. It only has time-based window criteria.
j. Back Pressure Handling
Back pressure is build up of data at an input-output when the buffer is full and not able to receive the additional incoming data. No data is transferred until the buffer is empty. Apache Spark is not capable of handling pressure implicitly rather it is done manually.
These are some of the major pros and cons of Apache Spark. We can overcome these limitations of Spark by using Apache Flink – 4G of Big Data.