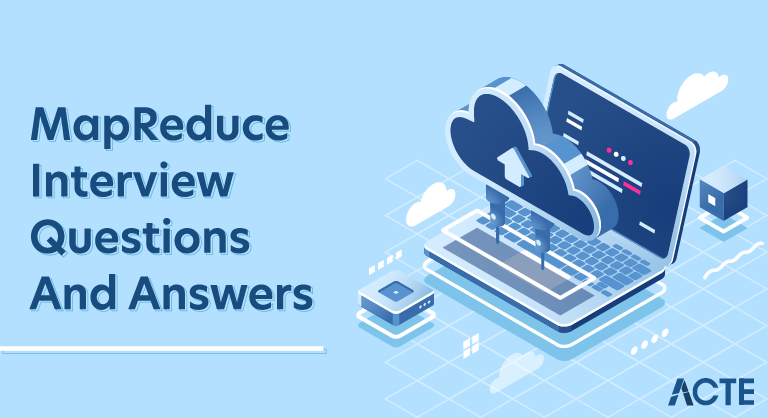
45+Top MapReduce Interview Questions & Answers
Last updated on 03rd Jul 2020, Blog, Interview Questions
MapReduce is a programming model and processing technique designed for distributed computing and handling large data sets. It operates by dividing the data processing into two primary phases: the “Map” phase, which involves breaking down a complex task into smaller sub-tasks and processing them in parallel, and the “Reduce” phase, which aggregates and summarizes the results from the Map phase to produce a single output or a reduced set of data.
1. What is MapReduce?
Ans:
MapReduce is a powerful programming model and processing technique for distributed computing. Google developed it to efficiently process large data sets across a distributed group of computers. MapReduce consists of two primary functions: Map (which processes and filters data) and Reduce (which aggregates and summarizes the results).
2. Explain the difference between the map and reduce functions.
Ans:
Feature | map | reduce |
---|---|---|
Purpose | Applies a function to each element in a list and returns a new list of results | Applies a function cumulatively to the items in a list to reduce it to a single value |
Output | List with the same number of elements as the input list | Single cumulative value |
Function Signature | map(function, iterable) | reduce(function, iterable[, initializer]) |
Common Use Cases | Transforming or processing elements individually | Aggregating data, such as summing a list or finding the product of elements |
3. Describe the data flow in a MapReduce job.
Ans:
The data flow in a MapReduce job follows these sequential steps:
- Input Splitting: The input data is split into smaller, manageable chunks for parallel processing.
- Mapping: Each split is processed by a Map task, which generates intermediate key-value pairs from the input data.
- Shuffling and Sorting: The intermediate key-value pairs are shuffled (grouped by key) and sorted for efficient processing.
4. What are key-value pairs in MapReduce?
Ans:
Key-value pairs are the fundamental data structures used in the MapReduce programming model. Each piece of data processed by MapReduce is represented as a key-value pair, in which the data linked to the key is the value, and the key is a unique identifier. These pairs are used both as input and output in the Map and Reduce functions, facilitating the processing, sorting, and aggregation of large data sets across a distributed computing environment.
5. What is the role of the Mapper?
Ans:
The Mapper’s role is to process input data and generate intermediate key-value pairs from the input data set. It performs filtering, transformation, and preparation of data for further processing by the Reducer. Each Mapper works on a portion of the data split independently, ensuring parallel processing and efficient utilization of computational resources. The Mapper helps in breaking down the input data into smaller, manageable pieces for the Reduce phase.
6. What is the role of the Reducer?
Ans:
The Reducer processes the intermediate key-value pairs generated by the Mapper. It aggregates, summarizes, and transforms these pairs to produce the final output. The Reducer processes grouped data by key and performs operations such as summation, averaging, or concatenation to generate meaningful results.
7. What is a Combiner in MapReduce?
Ans:
- A Combiner is an optional component in MapReduce that performs local aggregation of intermediate key-value pairs before they are sent to the Reducer.
- By combining intermediate results on the Mapper’s side, the Combiner reduces the amount of data transferred across the network, thereby improving efficiency and performance in the overall MapReduce job.
8. What is a Partitioner in MapReduce?
Ans:
A Partitioner determines how intermediate key-value pairs are divided among the Reducers. It ensures that the same Reducer receives all key-value pairs with the same key for consistent processing. The default partitioning strategy is based on hashing the key, but custom partitioners can be implemented for specific requirements.
9. Explain the shuffle and sort phase.
Ans:
The shuffle and sort phase occurs between the Map and Reduce phases in a MapReduce job. It involves the following steps:
- Shuffle: Intermediate key-value pairs generated by Mappers are transferred to the appropriate Reducers based on their keys.
- Sort: The key-value pairs are sorted by key within each Reducer to ensure that the Reducer processes all values for a given key together. The shuffle and sort phase is crucial for organizing and preparing the data for efficient processing by the Reducers.
10. What is the data locality in Hadoop MapReduce?
Ans:
Data locality refers to the principle of moving computation close to the data rather than moving data to the calculation. In Hadoop MapReduce, tasks are scheduled on nodes where the data is already present, reducing network congestion and improving overall performance. This is achieved by placing the computation on the same node or a nearby node where the data is stored for optimal processing efficiency.
11. Explain the OutputFormat class.
Ans:
The OutputFormat class in MapReduce is responsible for defining how the output data is written to the storage system. It specifies the output files’ format and structure, how records are written, and how output paths are handled. Typical implementations include TextOutputFormat for plain text files and SequenceFileOutputFormat for sequence files. The OutputFormat ensures that the final data output is formatted correctly and stored in a consistent and accessible manner.
12. What is the InputFormat class?
Ans:
The InputFormat class defines how input data is split and read by the Mapper in a MapReduce job. It is responsible for creating InputSplits and RecordReaders. The InputSplit defines a chunk of the input data that a single Mapper will process, while the RecordReader converts the data within the split into key-value pairs for the Mapper. Typical implementations include TextInputFormat for plain text files and KeyValueTextInputFormat for key-value pair text files.
13. Define RecordReader in MapReduce.
Ans:
- RecordReader is a component in MapReduce that reads input data from an InputSplit and converts it into key-value pairs that the Mapper processes.
- It handles the parsing of raw input data and presents it in a structured format suitable for mapping.
- The RecordReader ensures that data is accurately and efficiently read and transformed into intermediate key-value pairs for the mapping phase.
14. Describe the JobTracker.
Ans:
The JobTracker is a master daemon in the Hadoop MapReduce framework responsible for managing and coordinating the execution of MapReduce jobs. It handles job scheduling, monitoring, and resource allocation across the cluster. The JobTracker keeps track of all the tasks running on various TaskTrackers, reassigns failed tasks, and ensures overall job progress and completion.
15. What is the TaskTracker?
Ans:
The TaskTracker is a slave daemon in the Hadoop MapReduce framework that runs on each node in the cluster. It is responsible for executing the tasks assigned by the JobTracker, including Map and Reduce tasks. The TaskTracker regularly sends heartbeat messages to the JobTracker to report its status and task progress. It also handles task retries in case of failures.
16. How do MapReduce jobs handle failures?
Ans:
MapReduce jobs handle failures through task re-execution and fault tolerance mechanisms. If a Mapper or Reducer task fails, the JobTracker reassigns the task to another TaskTracker. Data is replicated across the cluster, ensuring that input data is available even if a node fails. Heartbeat messages from TaskTrackers help detect failures early, allowing the JobTracker to take corrective actions promptly.
17. What are counters in MapReduce?
Ans:
- Counters in MapReduce are a mechanism for tracking and reporting statistics or metrics during job execution.
- They count occurrences of specific events, such as the number of processed records, errors, or specific conditions met during task execution.
- Counters provide valuable insights for debugging, monitoring, and optimizing MapReduce jobs.
- Both user-defined and built-in counters are available.
18. What is speculative execution?
Ans:
Speculative execution in MapReduce is an optimization technique that mitigates the impact of slow-running tasks. When the JobTracker detects that a task is significantly slower than others, it may launch a duplicate (speculative) task on another node. The task that is completed first is accepted, and the other is terminated. This approach helps improve job completion times by addressing straggler tasks.
19. How can you optimize a MapReduce job?
Ans:
Optimizing a MapReduce job involves several strategies:
- Combiner: Use a Combiner to reduce the volume of intermediate data.
- Data Locality: Ensure data locality to minimize data transfer.
- Compression: Enable intermediate data compression to save bandwidth and storage.
- Partitioner: Implement custom partitioners for balanced load distribution.
- Memory Tuning: Adjust JVM settings and memory allocation.
- Efficient Algorithms: Design efficient maps and reduce algorithms.
20. Explain the concept of distributed cache in MapReduce.
Ans:
The distributed cache in MapReduce is a mechanism that allows files to be cached and made available to all nodes in the cluster. It is used to distribute read-only data files, such as lookup tables, configuration files, or libraries, that are needed by the tasks during execution. Files added to the distributed cache are automatically copied to the local file system of each node, ensuring efficient access and reducing the need for repeated data transfer from the central storage. This improves performance and ensures consistency across the cluster.
21. What are the differences between Hadoop 1 and Hadoop 2 regarding MapReduce?
Ans:
Resource Management: Hadoop 1 uses a single JobTracker for resource management and job scheduling, which can become a bottleneck. Hadoop 2 introduces YARN (Yet Another Resource Negotiator), which separates resource management from job scheduling, enhancing scalability and performance. Cluster Utilization: Hadoop 1’s cluster utilization is limited due to the single JobTracker. Hadoop 2’s YARN allows for better cluster resource utilization by running multiple types of distributed applications, not just MapReduce.
22. How does YARN enhance MapReduce functionality?
Ans:
YARN enhances MapReduce functionality by:
Decoupling Resource Management and Job Scheduling: YARN separates these functions into the ResourceManager and ApplicationMaster, allowing for more flexible and efficient resource allocation. Improving Scalability: YARN can handle a larger number of concurrent applications and nodes, overcoming the scalability limitations of Hadoop 1.
23. What are custom data types in MapReduce?
Ans:
Custom data types in MapReduce are user-defined classes that implement the Writable and WritableComparable interfaces. These custom types can be used as keys or values in MapReduce programs. They allow for more complex data structures beyond the default primitive types. Implementing custom data types involves defining the serialization and deserialization logic and the comparison methods for sorting keys.
24. Explain the purpose of the DistributedCache.
Ans:
The DistributedCache in MapReduce distributes read-only files needed by the Map and Reduce tasks across the cluster. It caches files locally on each node, ensuring that the data is available without repeatedly fetching it from a central location. This improves performance by reducing network overhead and ensuring consistency. Common use cases include configuration files, lookup tables, and libraries.
25. How can you implement a custom Partitioner?
Ans:
Implementing a custom Partitioner in MapReduce involves:
- Creating a Class: Implement the Partitioner interface.
- Override getPartition Method: Define logic to determine the partition based on the key.
- Configure Job: Set the custom partitioner class in the job configuration using `job.setPartitionerClass(MyCustomPartitioner.class)`.
- The custom partitioner helps ensure an even distribution of data across reducers based on specific requirements.
26. Describe everyday use cases of MapReduce.
Ans:
Everyday use cases of MapReduce include:
- Log Analysis: Processing and analyzing large volumes of server logs.
- Data Warehousing: ETL processes for loading and transforming large datasets.
- Web Indexing: Crawling and indexing web pages.
- Recommendation Systems: Building collaborative filtering models.
- Text Processing: Analyzing and processing giant text corpora, such as word count.
- Fraud Detection: Analyzing transaction data to detect fraudulent activities.
27. How do you handle large files in MapReduce?
Ans:
To handle large files in MapReduce:
- Split Files: Hadoop automatically splits large files into manageable chunks (input splits).
- Distributed Storage: Store files in HDFS, which supports large files and fault tolerance through replication.
- Parallel Processing: Leverage the distributed nature of MapReduce to process chunks in parallel.
- Compression: Use compression to reduce data size and improve I/O performance.
- Tuning Parameters: Optimize job parameters, such as the number of mappers and reducers, to handle large files efficiently.
28. Explain the chaining of MapReduce jobs.
Ans:
Chaining MapReduce jobs involves running multiple jobs sequentially, where the output of one job serves as the input for the next. This is useful for complex workflows requiring various stages of processing. Job chaining can be implemented using:
- Driver Code: Writing driver code to configure and submit each job in sequence.
- JobControl Class: Using Hadoop’s JobControl class to manage dependencies and job execution.
- Oozie: Utilizing Apache Oozie to define and manage complex workflows of MapReduce jobs.
29. How can you perform a join operation using MapReduce?
Ans:
Performing a join operation in MapReduce can be achieved through:
- Map-Side Join: Pre-sorting data and joining it during the map phase.
- Reduce-Side Join: Emitting keys from both datasets to the same Reducer, where the join is performed.
- Replicated Join: Using the DistributedCache to replicate a smaller dataset to all mappers and joining it with a larger dataset during the map phase.
- These methods facilitate joining large datasets distributed across a cluster.
30. Describe common performance bottlenecks.
Ans:
Common performance bottlenecks in MapReduce include:
Data Skew: Uneven distribution of data, causing some reducers to take longer.
Network Congestion: Excessive data transfer between nodes, especially during the shuffle phase.
Disk I/O: Slow read/write operations due to inefficient data access patterns.
Insufficient Resources: Limited CPU, memory, or disk space leading to resource contention.
31. How does the combine phase differ from the reduce phase?
Ans:
Combine Phase: The combined phase acts as a mini-reducer that runs on the Mapper nodes. Its primary purpose is to reduce the amount of data transferred between the Map and Reduce phases by performing local aggregation of intermediate key-value pairs before they are sent to the Reducers. Reduce Phase: The reduce phase is the final stage where the actual aggregation and processing of the intermediate data occur. Reducers receive all key-value pairs for a particular key and perform the final computation to produce the output.
32. What are map-side joins?
Ans:
Map-side joins are a join operation in MapReduce where the join logic is implemented in the map phase. This approach is efficient when one of the datasets is small enough to fit in memory and can be distributed to each Mapper. The small dataset is loaded into memory, and the larger dataset is streamed and joined during the map phase, reducing the need for data shuffling and improving performance.
33. What are reduce-side joins?
Ans:
Reduce-side joins are a join operation in MapReduce where the join logic is implemented in the reduce phase. Both datasets are mapped to emit join keys, and the corresponding values are shuffled to the same Reducer. The Reducer then performs the join operation by combining values with the same key.
34. How can you debug a MapReduce job?
Ans:
Debugging a MapReduce job can be done through several methods:
- Logs: Analyzing the logs generated by the JobTracker, TaskTracker, and individual tasks.
- Counters: Using built-in and custom counters to track job progress and identify issues.
- Local Mode: Running the job in local mode for easier debugging.
- Web UI: Monitoring the job execution and task details through the Hadoop Web UI.
- Debugging Scripts: Using Hadoop’s built-in scripts and tools to gather debugging information.
35. What is the role of the JobConf object?
Ans:
The JobConf object is used to configure a MapReduce job. It holds configuration parameters such as input and output paths, Mapper and Reducer classes, job-specific settings, and resource parameters. JobConf defines the job’s environment and controls various aspects of job execution.
36. How can you monitor and manage MapReduce jobs?
Ans:
MapReduce jobs can be monitored and managed through the following:
- Web UI: The Hadoop Web UI provides a dashboard to monitor job progress, task status, and resource utilization.
- Job History Server: After job completion, the Job History Server allows viewing past job details.
- Command Line Tools: Tools like `Hadoop job -status,` `Hadoop job -list,` and `Hadoop job -kill` can be used to manage jobs from the command line.
- Logs: Checking logs on HDFS and local file systems for detailed task information.
37. What are the limitations of MapReduce?
Ans:
Limitations of MapReduce include:
- Latency: High latency due to batch processing, making it unsuitable for real-time data processing.
- Complexity: Complex programming model requiring developers to write custom Map and Reduce functions.
- Data Flow: Limited data flow patterns, primarily supporting linear data flows.
- Iterative Processing: Inefficient for iterative algorithms due to repeated reading and writing of data.
- Resource Utilization: Poor resource utilization for small jobs, leading to inefficient cluster usage.
38. Describe the role of the Resource Manager in YARN.
Ans:
The Resource Manager in YARN is the master daemon responsible for managing cluster resources and scheduling applications. It tracks available resources on each node and allocates them to running applications based on scheduling policies. The ResourceManager ensures efficient resource utilization, handles resource requests from ApplicationMasters, and manages cluster-wide resource allocation.
39. What is the NodeManager in YARN?
Ans:
The NodeManager is a daemon that runs on each node in a YARN cluster. It is responsible for managing the execution of individual containers on its node, monitoring their resource usage (CPU, memory, disk), and reporting the node’s health and resource availability to the ResourceManager. The NodeManager ensures that tasks run efficiently and resources are utilized optimally.
40. How does the ApplicationMaster work in YARN?
Ans:
The ApplicationMaster is a per-application entity in YARN responsible for managing an application’s lifecycle. It negotiates resources with the Resource Manager, monitors task execution, handles task failures, and performs application-specific logic. The ApplicationMaster launches containers on NodeManagers, keeps track of their progress, and reports back to the ResourceManager. Each application has its own ApplicationMaster, providing isolation and better resource management.
41. What is the secondary sort in MapReduce?
Ans:
- Secondary sort in MapReduce refers to the capability to control the order of values within each key group during the shuffle and sort phase before they are passed to the Reducer.
- By default, MapReduce sorts values by keys. However, in cases where you need values within a critical group to be sorted in a specific order (secondary sort), you can implement techniques such as composite keys or custom partitioners to achieve this.
42. Explain the role of the InputSplit.
Ans:
InputSplit represents a chunk of data from an input file in Hadoop MapReduce. It is responsible for:
- Defining a logical division of the input data.
- Determining how much data each Mapper will process.
- Ensuring that each Mapper gets a portion of the input data to work on independently.
- InputSplits are created by the InputFormat and are passed to Mappers for processing.
43. How can you implement a custom RecordReader?
Ans:
Implementing a custom RecordReader involves:
- Creating a class that implements the RecordReader interface.
- Override methods to define how to read data from InputSplits and convert it into key-value pairs.
- Implement logic for parsing input data and handling record boundaries.
- Custom RecordReaders are used to handle different data formats or to perform specialized parsing and processing of input data in MapReduce jobs.
44. What are the benefits of using Hadoop MapReduce over traditional databases?
Ans:
- Scalability: Hadoop MapReduce can scale horizontally across commodity hardware, handling massive datasets that traditional databases may struggle with.
- Cost: Hadoop MapReduce leverages cost-effective storage solutions like HDFS, reducing storage costs compared to traditional databases.
- Flexibility: MapReduce can process diverse data types and formats, while traditional databases may be limited to structured data.
- Fault Tolerance: Hadoop’s built-in fault tolerance ensures job completion even if nodes fail, which can be challenging with traditional databases.
- Parallel Processing: MapReduce inherently supports parallel processing, enabling faster data processing on distributed systems compared to single-node databases.
45. How does MapReduce handle node failures?
Ans:
MapReduce handles node failures through fault tolerance mechanisms:
Data Replication: HDFS replicates data across multiple nodes, ensuring that data remains accessible if a node fails.
Task Redundancy: If a Mapper or Reducer task fails on a node, the JobTracker can reschedule the task on another available node.
46. Explain the difference between local and remote jobs.
Ans:
Local Job: A local job runs entirely on a single machine, typically used for development and testing purposes. The entire Hadoop framework (HDFS, MapReduce) runs locally on the developer’s machine.
Remote Job: A remote job runs on a Hadoop cluster with distributed storage (HDFS) and compute nodes (NodeManagers). Input and output data are stored in HDFS, and computation is distributed across multiple nodes in the cluster. Remote jobs are used for production deployments and large-scale data processing.
47. How can you use counters to track custom metrics?
Ans:
Counters in MapReduce can be used to track custom metrics by:
Defining custom counters using the `enum` provided by the job. Incrementing the counters in Mapper and Reducer tasks us `context.getCounter(EnumClass.CounterName).increment(1);`. Retrieving counter values after job completion to analyze job performance and specific metrics such as records processed, errors encountered, or custom business metrics.
48. What is the role of the Configuration object?
Ans:
The Configuration object in Hadoop MapReduce is used to set and retrieve job-specific configuration parameters. It includes settings such as input/output paths, Mapper and Reducer classes, input/output formats, and custom configuration properties. Configuration objects are initialized with defaults from the Hadoop configuration files and can be customized programmatically before submitting the job to the cluster.
49. How does data skew affect performance?
Ans:
Data skew in MapReduce occurs when specific keys have significantly more data than others. This can lead to performance issues:
- Uneven Workload: Reducers processing skewed keys may take longer to complete, slowing down the entire job.
- Resource Imbalance: Nodes handling skewed keys may experience high CPU and memory usage, affecting overall cluster performance.
- Straggler Tasks: Tasks processing skewed data may become stragglers, delaying job completion.
- To mitigate data skews, techniques such as custom partitioning, combiners, and data preprocessing can be used to distribute data more evenly across reducers.
50. Describe the Writable interface.
Ans:
The Writable interface in Hadoop serializes and deserializes custom Java objects for efficient data transfer between nodes in MapReduce jobs. Key and value objects in MapReduce must implement Writable or WritableComparable interfaces to be processed by Hadoop. The Writable interface defines methods for reading and writing data fields in a binary format, optimizing data serialization, and reducing network overhead in distributed computing environments like Hadoop.
51. Strategies for handling small files?
Ans:
Handling small files efficiently in Hadoop MapReduce involves:
- CombineFileInputFormat: Use CombineFileInputFormat to pack multiple small files into more significant input splits, reducing the number of Mappers.
- SequenceFileInputFormat: Convert small files into a single SequenceFile format, improving processing efficiency.
- Custom InputFormat: Implement a custom InputFormat to merge small files or aggregate data before processing.
- HDFS Configuration: Adjust HDFS block size and replication factor to optimize storage and access for small files.
52. Explain the concept of a combiner.
Ans:
A combiner in MapReduce is an optional optimization technique used to reduce the amount of data transferred between the Mapper and the Reducer phases. It is a mini-reduce operation that runs on the output of the Mapper before data is shuffled and sent to Reducers. The combiner aggregates key-value pairs with the same key locally on each Mapper node. Combiners are typically used for operations that are both associative and commutative, such as summing or counting.
53. How can you use the ToolRunner class?
Ans:
The ToolRunner class in Hadoop provides a convenient way to run MapReduce jobs from the command line, handling everyday tasks such as parsing command-line arguments and configuring job parameters. To use ToolRunner, follow these steps:
- Implement the Tool interface in your main MapReduce driver class.
- Override the run method to configure and submit your MapReduce job.
- Use the ToolRunner.run method to launch your job with command-line arguments.
54. What is a custom comparator?
Ans:
A custom comparator in Hadoop MapReduce is used to define the sorting order of keys during the shuffle and sort phase before they are passed to the Reducers. By default, keys are sorted in ascending order based on their natural order (lexicographical for strings, numerical for integers). A custom comparator allows developers to define a specific order for keys that do not follow their natural order. Custom comparators are implemented by extending the WritableComparator class and overriding the compare method to provide the desired sorting logic.
55. How does MapReduce handle duplicate keys?
Ans:
In MapReduce, duplicate keys are handled based on the processing logic defined by the developer:
- Mapper: Duplicate keys emitted by the Mapper are grouped and sent to the same Reducer, preserving the order in which they were emitted.
- Reducer: During the reduce phase, all values associated with a duplicate key are passed to the Reducer’s reduce method, allowing developers to aggregate, process, or discard duplicate data as needed.
- Output: The final output of a MapReduce job can include duplicate keys if multiple Mappers emitted them. Developers can implement logic within the Reducer to handle duplicates appropriately, such as summing values or selecting the most recent value.
56. Explain task execution in MapReduce.
Ans:
Task execution in MapReduce involves several stages:
- Job Submission: The client submits a MapReduce job to the JobTracker.
- Task Assignment: The JobTracker assigns tasks (Map and Reduce) to available TaskTrackers based on data locality and resource availability.
- Task Execution: TaskTrackers execute tasks on assigned nodes. Mappers process input data and generate intermediate key-value pairs. Reducers fetch intermediate data, group by keys, and process each group to produce the final output.
- Task Completion: TaskTrackers report task status (success or failure) to the JobTracker.
57. What is the MapReduce framework, and how does it differ from core MapReduce?
Ans:
MapReduce Framework: Refers to the broader ecosystem and tools (like Hadoop MapReduce, Apache Spark, etc.) that implement the MapReduce programming model for large-scale data processing.
Core MapReduce: This term refers explicitly to the programming model itself, which consists of a map and reduce functions for processing data in parallel across a distributed cluster.
58. How can you use Hadoop’s Streaming API?
Ans:
- Mapper and Reducer Scripts: Write Mapper and Reducer logic in any scripting language (Python, Perl, etc.).
- Streaming Jar: Use the Hadoop streaming jar to submit jobs with these scripts.
- Data Formats: Input data is streamed line by line to Mapper, and output is streamed line by line from Reducer.
- Flexibility: Allows leveraging existing scripts and tools, extending MapReduce capabilities beyond Java.
59. Role of Apache Pig and Hive in relation to MapReduce?
Ans:
Apache Pig: A high-level scripting language for analyzing large datasets in Hadoop. Pig scripts are translated into MapReduce jobs automatically, abstracting the complexity of Java-based MapReduce programming. It provides a data flow language (Pig Latin) for data transformation and querying.
Apache Hive: An infrastructure for data warehouses based on Hadoop that provides data summarization, query, and analysis. Hive queries are translated into MapReduce jobs or executed directly using Tez or Spark for improved performance. HiveQL (similar to SQL) allows users to query data stored in Hadoop.
60. How does compression affect jobs?
Ans:
Compression in MapReduce can affect jobs in several ways:
- Input Compression: Compressing input data reduces storage requirements and improves input/output performance by reducing disk reads and writes.
- Intermediate Compression: Compressing intermediate data reduces the amount of data shuffled between Mappers and Reducers, reducing network traffic and improving overall job performance.
- Output Compression: Compressing output data reduces storage requirements and improves output file size, which can be beneficial for downstream processing or storage.
61. What are custom Writable and WritableComparable in MapReduce?
Ans:
Custom Writable: Custom Writable objects in MapReduce are user-defined classes that implement the Writable interface. They define how data is serialized and deserialized for efficient transfer between Mappers and Reducers. Custom Writables represent complex data types that are not natively supported by Hadoop’s default Writable types (like IntWritable and Text).
62. Explain the role of job setup, job execution, and job cleanup in MapReduce.
Ans:
Job Setup: The job setup phase involves configuring and initializing resources required for the MapReduce job, such as input/output paths, Mapper and Reducer classes, job-specific settings, and distributed cache files. This phase prepares the environment for job execution.
Job Execution: During job execution, Mappers read input data splits, apply the map function to each record, and produce intermediate key-value pairs. These intermediate pairs are then partitioned, sorted, and shuffled to Reducers, which use the reduce function to make the final output.
63. How does the MapReduce framework determine the number of reducers?
Ans:
The number of reducers in MapReduce can be determined by:
- Default Configuration: If not explicitly set by the user, Hadoop defaults to a single reducer.
- Configuration Parameter: Developers can specify the number of reducers using `job.setNumReduceTasks(int num)` method in the job configuration.
- Data Size: Hadoop estimates the amount of data generated by Mappers for each key and aims to assign a balanced workload to each Reducer. More reducers can improve parallelism but may increase overhead due to task scheduling and data shuffling.
- Cluster Capacity: The number of available nodes and their capacity influence the number of reducers that can run concurrently without resource contention.
64. What are skewed joins in MapReduce, and how do we handle them?
Ans:
- Custom Partitioning: Custom partitioners are implemented to distribute data more evenly among reducers based on skewed keys.
- Sampling and Preprocessing: Sampling data to identify skewed keys and applying preprocessing techniques such as data skew mitigation algorithms.
- Composite Keys: Composite keys or secondary sorting techniques are used to distribute data more evenly across reducers.
- Aggregating Data: Pre-aggregating data to reduce the number of keys and distribute work more evenly across reducers.
65. Explain chain reducer and chain mapper in MapReduce.
Ans:
Chain Reducer: Chain Reducer is a technique in MapReduce where the output of one Reducer is passed as input to another Reducer, allowing sequential execution of multiple reduce tasks. This enables complex data processing workflows where each Reducer performs a different transformation or aggregation on the data.
Chain Mapper: Chain Mapper is similar but applies to Mappers, where the output of one Mapper is passed as input to another Mapper. This allows the chaining of multiple map tasks in sequence, facilitating data transformation or preprocessing steps before the main MapReduce computation.
66. Describe the use of job chaining and job control in MapReduce.
Ans:
Job Chaining: Job chaining refers to the practice of linking multiple MapReduce jobs together in sequence, where the output of one job serves as the input to the next job. This allows developers to create complex data processing pipelines or workflows that involve multiple stages of computation or analysis. Job Control: Job control in MapReduce involves managing dependencies and execution orders between multiple jobs in a workflow. It ensures that jobs are executed in sequence based on their dependencies and that subsequent jobs start only after the successful completion of preceding jobs.
67. Write a MapReduce program to count word frequency:
Ans:
- “`Java
- public class WordCount {
- public static class TokenizerMapper extends Mapper
{ - private final static IntWritable one = new IntWritable(1);
- private Text word = new Text();
- public void map(LongWritable key, Text value, Context context) throws IOException, InterruptedException {
- StringTokenizer itr = new StringTokenizer(value.toString());
- while (itr.hasMoreTokens()) {
- word.set(itr.nextToken());
- context.write(word, one);
- }
- }
- }
- public static class IntSumReducer extends Reducer
{ - private IntWritable result = new IntWritable();
- public void reduce(Text key, Iterable
values, Context context) throws IOException, InterruptedException { - int sum = 0;
- for (IntWritable val: values) {
- sum += val.get();
- }
- result.set(sum);
- context.write(key, result);
- }
- }
- throws Exception in public static void main(String[] args). {
- Configuration conf = new Configuration();
- Job job = Job.getInstance(conf, “word count”);
- job.setJarByClass(WordCount.class);
- job.setMapperClass(TokenizerMapper.class);
- job.setCombinerClass(IntSumReducer.class);
- job.setReducerClass(IntSumReducer.class);
- job.setOutputKeyClass(Text.class);
- job.setOutputValueClass(IntWritable.class);
- FileInputFormat.addInputPath(job, new Path(args[0]));
- FileOutputFormat.setOutputPath(job, new Path(args[1]));
- System.exit(job.wait for completion(true) ? 0 : 1);
- }
- }
- “`
68. How would you optimize a sorting job?
Ans:
Custom partitioners and comparators are used to control data distribution and sorting order. Utilize combiners to reduce the amount of data shuffled across the network. Adjust the number of reducers based on data size and cluster resources. Implement secondary sorting techniques if needed. Preprocess data to reduce the amount of data being sorted.
69. Steps to set up a MapReduce environment:
Ans:
- Install Hadoop on a cluster or a single node.
- Configure core-site.xml, hdfs-site.xml, and mapred-site.xml for Hadoop settings.
- Set up HDFS for distributed storage and configure data nodes.
- Ensure proper network connectivity and security settings.
- Write MapReduce programs using Java, configure job settings, and submit jobs using Hadoop CLI or APIs.
- Monitor job execution and manage cluster resources using Hadoop Web UI or command-line tools.
70. Explain log analysis using MapReduce:
Ans:
Use MapReduce to parse log files and extract relevant information (e.g., IP addresses, timestamps, URLs). Count occurrences of specific events or errors. Aggregate statistics such as request counts per hour, top URLs accessed, or user behavior patterns. Perform anomaly detection or pattern recognition based on log data. Output results to HDFS or other storage for further analysis or visualization.
71. Implement distributed grep using MapReduce:
Ans:
- Mapper: Search for a specific pattern (e.g., a word or phrase) in each line of input data.
- Reducer: Collect and output lines that contain the specified pattern.
- Use Hadoop’s TextInputFormat for input and TextOutputFormat for output.
- Handle distributed file paths and input splits to process data across multiple nodes.
- Customize Mapper and Reducer logic to filter and process data efficiently.
72. Create an application to determine the average temperature:
Ans:
- “`Java
- public class AverageTemperature {
- public static class TemperatureMapper extends Mapper
{ - private Text year = new Text();
- private IntWritable temperature = new IntWritable();
- public void map(LongWritable key, Text value, Context context) throws IOException, InterruptedException {
- String[] fields = value.toString().split(“,”);
- year.set(fields[0]);
- temperature.set(Integer.parseInt(fields[1]));
- context.write(year, temperature);
- }
- }
- public static class AverageReducer extends Reducer
{ - private DoubleWritable result = new DoubleWritable();
- public void reduce(Text key, Iterable
values, Context context) throws IOException, InterruptedException { - int sum = 0;
- int count = 0;
- for (IntWritable val: values) {
- sum += val.get();
- count++;
- double average = (double) sum/count;
- result.set(average);
- context.write(key, result);
- }
- }
- public static void main(String[] args) throws Exception {
- Configuration conf = new Configuration();
- Job job = Job.getInstance(conf, “average temperature”);
- job.setJarByClass(AverageTemperature.class);
- job.setMapperClass(TemperatureMapper.class);
- job.setReducerClass(AverageReducer.class);
- job.setOutputKeyClass(Text.class);
- job.setOutputValueClass(IntWritable.class);
- FileInputFormat.addInputPath(job, new Path(args[0]));
- FileOutputFormat.setOutputPath(job, new Path(args[1]));
- System.exit(job.wait for completion(true) ? 0 : 1);
- }
- }
- “`
73. Handling skewed data in a job:
Ans:
- Identify skewed keys by analyzing job logs or using profiling tools.
- Implement custom partitioners to distribute data more evenly among reducers.
- Use combiners to reduce data volume early in the Map phase.
- Preprocess data to reduce skewness before it enters the MapReduce job.
- Adjust the number of reducers dynamically based on data skewness.
- Implement data skew handling algorithms such as adaptive partitioning or skewed join techniques.
74. Process web server logs using MapReduce:
Ans:
- Mapper: Extract relevant information from each log entry (e.g., IP address, timestamp, URL).
- Reducer: Aggregate data based on keys (e.g., count occurrences of each URL, summarize traffic per IP address).
- Use Hadoop’s TextInputFormat to read log files and TextOutputFormat to write results.
- Perform data preprocessing as needed to handle log format variations.
75. Perform ETL operations using MapReduce:
Ans:
- Extract: Read data from input sources (e.g., files, databases).
- Transform: Apply business logic or data transformations (e.g., filtering, aggregation) in Mapper and Reducer tasks.
- Load: Write transformed data to output destinations (e.g., files, databases).
- Use custom input formats or connectors to extract data from different sources.
- Implement appropriate logic in MapReduce jobs for transforming and loading data.
76. Write a job to find the top N records:
Ans:
- “`Java
- public class TopNRecords {
- public static class TopNMapper extends Mapper
{ - private TreeMap
topRecords = new TreeMap<>(); - public void map(LongWritable key, Text value, Context context) throws IOException, InterruptedException {
- // Parse input and extract necessary fields
- // Example: extract a numeric value for comparison (like a score)
- int score = Integer.parseInt(value.toString().split(“,”)[1]);
- // Add a record to TreeMap with the score as a key to keep top N records
- top records.put(score, new Text(value));
- // Keep only top N records
- if (top records. size() > N) {
- top records.remove(top records.first());
- }
- }
- protected void cleanup(Context context) throws IOException, InterruptedException {
- // Emit all top N records from TreeMap
- for (Text record: top records. values()) {
- context.write(NullWritable.get(), record);
- }
- }
- }
- public static class TopNReducer extends Reducer
{ - private TreeMap
topRecords = new TreeMap<>(); - public void reduce(NullWritable key, Iterable
values, Context context) throws IOException, InterruptedException { - for (Text value: values) {
- // Parse input and extract necessary fields
- // Example: extract a numeric value for comparison (like a score)
- int score = Integer.parseInt(value.toString().split(“,”)[1]);
- // Add a record to TreeMap with the score as a key to keep top N records
- top records.put(score, new Text(value));
- // Keep only top N records
- if (top records. size() > N) {
- top records.remove(top records.first());
- // Emit all top N records from TreeMap
- for (Text record: top records.descendingMap().values()) {
- context.write(NullWritable.get(), record);
- }
- }
- }
- public static void main(String[] args) throws Exception {
- Configuration conf = new Configuration();
- Job job = Job.getInstance(conf, “top N records”);
- job.setJarByClass(TopNRecords.class);
- job.setMapperClass(TopNMapper.class);
- job.setReducerClass(TopNReducer.class);
- job.setOutputKeyClass(NullWritable.class);
- job.setOutputValueClass(Text.class);
- FileInputFormat.addInputPath(job, new Path(args[0]));
- FileOutputFormat.setOutputPath(job, new Path(args[1]));
- System.exit(job.wait for completion(true) ? 0 : 1);
- }
- }
- “`
77. Describe the process of debugging a failing MapReduce job:
Ans:
- Check Logs: Review Hadoop job logs for errors, exceptions, and stack traces.
- Input Data: Verify input data format, quality, and accessibility (HDFS or local).
- Configuration: Ensure job configuration settings (e.g., mapper/reducer classes, input/output paths) are correct.
- Resource Availability: Check cluster resource availability (e.g., nodes, memory) and job queue status.
78. How do you join two datasets using MapReduce?
Ans:
- Mapper: Emit key-value pairs where the key is the join key and the value indicates the origin of the record (e.g., from dataset A or B).
- Reducer: For each key, iterate over the list of values (from both datasets) and perform the join operation (e.g., inner join, outer join, etc.).
- Implement custom logic in MapReduce jobs to handle different join types (e.g., using secondary sort or composite keys for efficient processing).
79. Write a MapReduce program to find the maximum value for each key:
Ans:
- “`Java
- public class MaxValueByKey {
- public static class MaxValueMapper extends Mapper
{ - private Text word = new Text();
- private IntWritable value = new IntWritable();
- public void map(LongWritable key, Text input, Context context) throws IOException, InterruptedException {
- String[] tokens = input.toString().split(“\\s+”);
- word.set(tokens[0]);
- value.set(Integer.parseInt(tokens[1]));
- context.write(word, value);
- }
- }
- public static class MaxValueReducer extends Reducer
{ - private IntWritable result = new IntWritable();
- public void reduce(Text key, Iterable
values, Context context) throws IOException, InterruptedException { - int maxValue = Integer.MIN_VALUE;
- for (IntWritable val: values) {
- maxValue = Math.max(maxValue, Val.get());
- }
- result.set(maxValue);
- context.write(key, result);
- }
- }
- public static void main(String[] args) throws Exception {
- Configuration conf = new Configuration();
- Job job = Job.getInstance(conf, “max value by key”);
- job.setJarByClass(MaxValueByKey.class);
- job.setMapperClass(MaxValueMapper.class);
- job.setReducerClass(MaxValueReducer.class);
- job.setOutputKeyClass(Text.class);
- job.setOutputValueClass(IntWritable.class);
- FileInputFormat.addInputPath(job, new Path(args[0]));
- FileOutputFormat.setOutputPath(job, new Path(args[1]));
- System.exit(job.wait for completion(true) ? 0 : 1);
- }
- }
- “`
80. How would you implement a word count program using a combiner?
Ans:
- “`Java
- public class WordCountWithCombiner {
- public static class TokenizerMapper extends Mapper
{ - private final static IntWritable one = new IntWritable(1);
- private Text word = new Text();
- public void map(LongWritable key, Text value, Context context) throws IOException, InterruptedException {
- StringTokenizer itr = new StringTokenizer(value.toString());
- while (itr.hasMoreTokens()) {
- word.set(itr.nextToken());
- context.write(word, one);
- }
- }
- }
- public static class IntSumReducer extends Reducer
{ - private IntWritable result = new IntWritable();
- public void reduce(Text key, Iterable
values, Context context) throws IOException, InterruptedException { - int sum = 0;
- for (IntWritable val: values) {
- sum += val.get();
- }
- result.set(sum);
- context.write(key, result);
- }
- }
- public static void main(String[] args) throws Exception {
- Configuration conf = new Configuration();
- Job job = Job.getInstance(conf, “word count with combiner”);
- job.setJarByClass(WordCountWithCombiner.class);
- job.setMapperClass(TokenizerMapper.class);
- job.setCombinerClass(IntSumReducer.class); // Set combiner
- job.setReducerClass(IntSumReducer.class);
- job.setOutputKeyClass(Text.class);
- job.setOutputValueClass(IntWritable.class);
- FileInputFormat.addInputPath(job, new Path(args[0]));
- FileOutputFormat.setOutputPath(job, new Path(args[1]));
- System.exit(job.wait for completion(true) ? 0 : 1);
- }
- }
- “`
These examples cover a range of practical scenarios and implementations using MapReduce, from basic data processing tasks to more complex operations such as join operations, top N records, and log analysis.
81. How does MapReduce handle data locality?
Ans:
- MapReduce strives to process data where it resides to minimize network traffic and maximize performance.
- Hadoop’s HDFS stores data in blocks across the cluster. MapReduce attempts to schedule tasks (Mappers and Reducers) on nodes where data blocks are stored (data locality).
- Tasks are scheduled preferentially on nodes with local data blocks. If this is not possible (e.g., due to node failure or high resource demand), tasks may be scheduled on nodes with replica blocks.
- Data locality optimization reduces network overhead and improves overall job performance in Hadoop MapReduce.
82. Design patterns used in MapReduce:
Ans:
- Map-only: Tasks that only require a mapping phase are helpful for data extraction or filtering.
- Reduce-side join: Joining datasets based on a shared key during the reduce phase.
- Secondary sort: Sorting records within reducers to achieve a secondary sorting order.
- Partition pruning: Filtering data at the mapper stage based on partitioning logic.
- Counters: Tracking job-specific metrics like the number of records processed.
- Combiner: Reducing data volume transferred between mappers and reducers by performing partial aggregation.
- Custom partitioner: Controlling data distribution across reducers based on custom logic.
83. Pros and cons of using MapReduce:
Ans:
Pros:
- Scalability: Handles large-scale data processing across distributed clusters.
- Fault tolerance: Automatically recovers from node failures by re-executing failed tasks.
- Flexibility: Supports a variety of data processing tasks through customizable Mappers and Reducers.
- Ecosystem: Integrates with various tools and frameworks within the Hadoop ecosystem (e.g., HDFS, YARN, Hive, Pig).
Cons:
- Overhead: High latency due to the batch-oriented processing model.
- Complexity: Requires understanding of distributed computing concepts and Java programming (for Java-based MapReduce).
- Resource utilization: Inefficient for iterative or real-time processing compared to newer frameworks like Apache Spark.
84. Difference between synchronous and asynchronous jobs:
Ans:
Synchronous jobs Execute tasks in a sequential order, with each task requiring completion before the next one begins. The caller waits for the job to finish and receives a response once it’s done. Asynchronous jobs: Submit tasks or jobs that run independently and may be completed at different times. The caller doesn’t wait and typically receives a job ID or handle to monitor progress or retrieve results later.
85. Configuring memory settings for a job:
Ans:
Adjust memory settings in the job configuration using `mMapReducemap.memory.mb` and `MapReduce.reduce.memory.mb` for Mapper and Reducer memory allocation, respectively. Configure Java heap sizes using `mapreduce.map.java.opts` and `mapreduce.reduce.java.opts` for JVM options. Set container sizes and memory overhead using `yarn.scheduler.minimum-allocation-mb` and `yarn.scheduler.maximum-allocation-mb` in YARN configuration. Consider task-specific memory requirements and adjust settings based on job characteristics and cluster resources to optimize performance.
86. Security features in Hadoop MapReduce:
Ans:
- Authentication: Supports Kerberos authentication for secure access to Hadoop services.
- Authorization: Access control lists (ACLs) and file permissions ensure authorized access to data stored in HDFS.
- Data encryption: Data transmission between nodes and storage in HDFS can be encrypted using SSL/TLS.
87. Handling large-scale data sorting:
Ans:
- Distribution: Distribute data across multiple nodes in the cluster using HDFS.
- MapReduce Sorting: Utilize MapReduce framework for distributed sorting.
- Secondary Sort: Implement secondary sorting techniques within reducers to achieve the desired sorting order.
- Custom Partitioning: Control data distribution across reducers based on custom partitioners.
- Optimization: Adjust memory settings, tune cluster resources, and optimize job configurations for efficient sorting performance.
- Scalability: Hadoop MapReduce scales horizontally to handle large datasets and sorts them in parallel across nodes in the cluster.
88. Architecture of a typical cluster:
Ans:
Controller Node:
- NameNode: Manages HDFS file system metadata, handles file operations, and maintains data block locations.
- ResourceManager: Manages resource allocation, job scheduling, and task tracking in YARN.
Worker Nodes:
- DataNode: Stores data blocks in HDFS and manages block replication.
- NodeManager: Manages resources (CPU, memory) on a node and executes tasks (containers) assigned by ResourceManager.
- Networking: High-speed interconnects facilitate communication between nodes for data transfer and job coordination.
89. Unit testing for MapReduce jobs:
Ans:
- Frameworks: Use testing frameworks like MRUnit, which provides utilities for testing MapReduce jobs in a simulated environment.
- Mocking: Mock input data and output formats to simulate different scenarios and edge cases.
- Validation: Validate Mapper and Reducer logic, input splits, key-value pairs, and output formats.
90. Role of HDFS in MapReduce:
Ans:
- Storage: HDFS serves as the primary storage layer for input data, intermediate data, and output data generated by MapReduce jobs.
- Data Locality: HDFS stores data blocks across nodes in the cluster and enables MapReduce tasks to run close to the data they process, optimizing data locality.
- Fault Tolerance: HDFS replicates data blocks across multiple nodes to ensure data availability and reliability, which is critical for fault tolerance in MapReduce jobs.
- Scalability: HDFS scales horizontally by adding more nodes to the cluster, accommodating large-scale data processing requirements of MapReduce jobs.