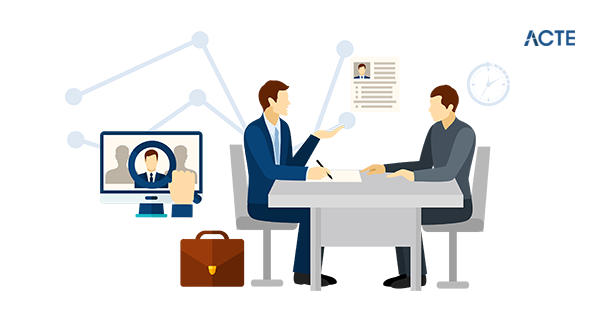
35+ [REAL-TIME] PySpark Interview Questions and Answers
Last updated on 20th Jun 2020, Blog, Interview Questions
PySpark is a powerful tool for big data processing, enabling scalable and efficient data analysis using Apache Spark and Python. It simplifies working with large datasets, providing a high-level API for distributed data processing and machine learning tasks. Ideal for data engineers and data scientists, PySpark accelerates data workflows and allows seamless integration with various data sources and big data frameworks.
1. What is PySpark?
Ans:
PySpark is the Python API for Apache Spark, a powerful distributed computing system designed for big data processing and analytics. It enables Python developers to leverage Spark’s extensive capabilities, including its high-level APIs for SQL, machine learning, graph computation, and streaming data. By using PySpark, developers can write applications to process and analyze large-scale data efficiently, utilizing Spark’s in-memory computing and fault-tolerant architecture.
2. How does PySpark relate to Apache Spark?
Ans:
PySpark is a part of Apache Spark, specifically designed to provide a Python interface for Spark. Apache Spark is a robust and versatile cluster-computing framework for large-scale data processing, and PySpark enables Python developers to utilize Spark’s distributed data processing power through Python.
3. Explain the architecture of PySpark.
Ans:
The architecture of PySpark includes several core components: the Driver Program, which is responsible for running the main application, task scheduling, and distributing work; the Cluster Manager, which handles resource management and works with the driver to allocate resources (examples include YARN, Mesos, or Spark’s standalone manager); the Executors, which are worker nodes that perform data processing tasks in parallel; and the SparkSession, which serves as the entry point for creating and managing DataFrames and offers the main interface to Spark’s functionality.
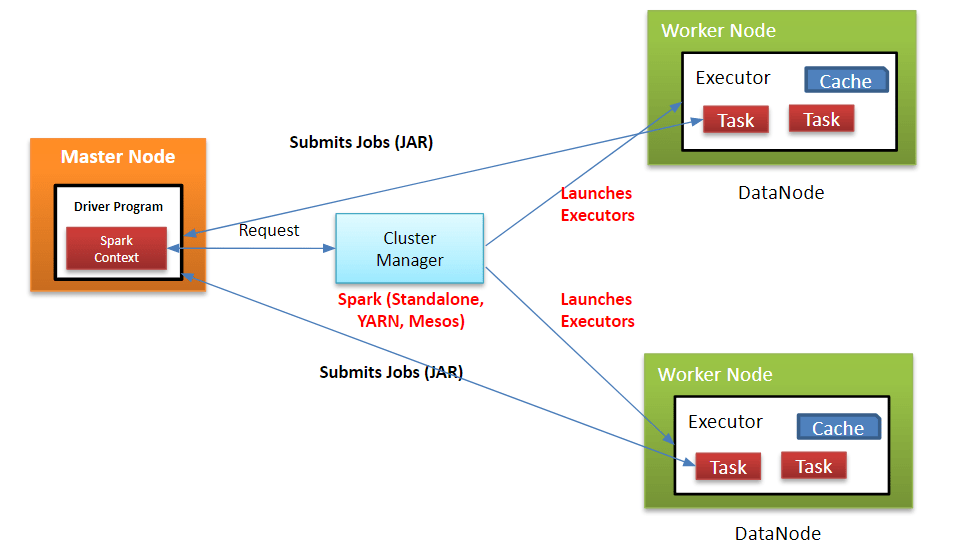
4. What are the benefits of using PySpark over traditional data processing frameworks?
Ans:
PySpark offers numerous benefits over traditional data processing frameworks, such as scalability, which allows it to handle extensive data processing across many machines; speed, thanks to in-memory computation and efficient execution plans; ease of use with high-level Python APIs; fault tolerance through resilient distributed datasets (RDDs) and DataFrames; and seamless integration with other big data tools and frameworks like Hadoop, Kafka, and various data storage systems.
5. How do you install PySpark?
Ans:
- PySpark can be installed easily using the pip package manager with the command `pip install pyspark`.
- Alternatively, for specific versions or particular installation requirements, you can download the binary package from the Apache Spark website and set it up manually.
6. How do you initialize a SparkSession in PySpark?
Ans:
You can initialize a `SparkSession` in PySpark with the following code:
- from pyspark.sql import SparkSession
- spark = SparkSession.builder \
- .appName(“example”) \
- .getOrCreate()
7. Explain the RDD (Resilient Distributed Dataset) in PySpark.
Ans:
An RDD (Resilient Distributed Dataset) in PySpark is a core data structure that represents an immutable, distributed collection of objects processed in parallel. RDDs support two types of operations: transformations, which produce a new RDD from an existing one (such as `map` or `filter`), and actions, which return a result to the driver after computing on the RDD (such as `count` or `collect`). RDDs ensure fault tolerance through lineage information, enabling them to be recomputed in case of failures.
8. What are the different ways to create RDDs in PySpark?
Ans:
- You can create RDDs in PySpark in multiple ways: by parallelizing an existing Python collection.
- For example, `spark.sparkContext.parallelize([1, 2, 3, 4, 5])`, or by reading external data from storage systems like HDFS, S3, or a local file system using `spark.sparkContext.textFile(“path/to/file.txt”)`.
9. What is DataFrame in PySpark? How is it different from RDD?
Ans:
Feature | DataFrame | RDD |
---|---|---|
Schema and Structure | Structured with named columns and data types | Unstructured, no schema, collection of objects |
Ease of Use | High-level API, similar to SQL | Low-level API, requires complex code |
Performance | Optimized by Catalyst and Tungsten for faster execution | Lacks built-in optimizations, generally slower |
Interoperability | Seamless integration with SQL queries and MLlib | More complex integration with external tools |
10. How do you create a data frame in PySpark?
Ans:
- You can create a DataFrame in PySpark in various ways. From an existing RDD, you can convert it using `spark.createDataFrame(rdd)`.
- From a structured data file such as CSV or JSON, you can read the data using `spark.read.csv(“path/to/file.csv”, header=True, inferSchema=True)`.
- Additionally, you can convert a pandas DataFrame to a PySpark DataFrame using `spark.createDataFrame(pandas_dataframe)`.
11. Explain the transformations and actions in PySpark.
Ans:
- In PySpark, transformations and actions are two types of operations that can be performed on RDDs and DataFrames.
- Transformations generate a new dataset from an existing one and are lazy, meaning they build up a computation plan without executing immediately.
- Examples include `map()`, `filter()`, and `groupBy()`. Actions trigger the execution of these transformations to produce a result.
- They either return a value to the driver or write data to external storage. Examples include `collect()`, `count()`, and `saveAsTextFile()`.
12. What are narrow and wide transformations in PySpark?
Ans:
Transformations in PySpark are classified into narrow and wide. Narrow transformations (like `map` and `filter`) only require data from a single partition to generate the output. They are efficient and involve minimal data shuffling. Wide transformations (like `reduceByKey` and `groupByKey`) require data from multiple partitions, causing a shuffle across nodes, which is more complex and resource-intensive.
13. How do you select columns from a DataFrame in PySpark?
Ans:
Columns can be selected from a DataFrame in PySpark using the `select()` method or by accessing them directly. For example:
- # Using select method
- df.select(“column1”, “column2”)
- # Accessing columns directly
- df[“column1”], df[“column2”]
14. Explain the difference between dropDuplicates() and dropna() in PySpark.
Ans:
- In PySpark, `dropDuplicates()` and `dropna()` are used for data cleaning. `dropDuplicates()` removes rows that are duplicates based on specified columns, ensuring each row is unique.
- If no columns are specified, it considers all columns. `dropna()` removes rows with null values.
- You can configure it to remove rows with any nulls or specify criteria for the number of null values.
15. How can you rename a column in a PySpark DataFrame?
Ans:
To rename a column in a PySpark DataFrame, use the `withColumnRenamed()` method. For example:
- df = df.withColumnRenamed(“oldColumnName”, “newColumnName”)
16. How do you handle missing or null values in PySpark DataFrames?
Ans:
Handling missing or null values in PySpark DataFrames can be done using several methods:
- `dropna()`: Removes rows with null values.
- `fillna()`: Replaces null values with a specified value.
- `replace()`: Substitutes certain values, including nulls.
Example:
- # Drop rows with any null values
- df = df.drop()
- # Fill null values with a specified value
- df = df.fillna({“column1”: 0, “column2”: “missing”})
- # Replace null values
- df = df.replace(to_replace=None, value=”default”)
17. Explain how to filter rows in a PySpark data frame.
Ans:
Rows in a PySpark DataFrame can be filtered using the `filter()` or `where()` methods, which are functionally equivalent. For example:
- # Using filter method
- df_filtered = df.filter(df[“column”] > 10)
- # Using where method
- df_filtered = df.where(df[“column”] > 10)
18. What is the significance of DataFrame caching in PySpark?
Ans:
Caching a data frame in PySpark is significant because it improves performance for iterative and interactive computations. By storing a data frame in memory, subsequent operations can access data faster, reducing the need to recompute the data frame from source data multiple times. This is particularly useful when the data frame is used repeatedly in different operations.
19. How do you join two DataFrames in PySpark?
Ans:
Two DataFrames can be joined in PySpark using the `join()` method, with various join types like inner, left, right, and outer joins. For example:
- df1 = Spark.createDataFrame([(1, “A”), (2, “B”)], [“id”, “value1”])
- df2 = Spark.createDataFrame([(1, “X”), (3, “Y”)], [“id”, “value2”])
- # Inner join on ‘id’
- df_joined = df1.join(df2, on=”id”, how=”inner”)
20. Explain the concept of DataFrame persistence in PySpark.
Ans:
- DataFrame persistence in PySpark involves storing a DataFrame in memory or on disk to optimize performance for subsequent operations.
- This can be done using the `persist()` or `cache()` methods. Persistence allows you to specify storage levels (e.g., MEMORY_ONLY, MEMORY_AND_DISK), while caching is shorthand for MEMORY_ONLY persistence.
- Persisting a data frame is particularly beneficial for iterative algorithms and repeated queries, as it prevents the need to recompute the data frame multiple times.
21. What is Spark SQL, and how is it used in PySpark?
Ans:
- Spark SQL is a component of Apache Spark that is designed to work with structured data.
- It provides an interface for executing SQL queries and integrates seamlessly with Spark’s DataFrame API.
- In PySpark, Spark SQL can be utilized by creating a `SparkSession`, which offers methods to read data, run SQL queries, and manipulate DataFrames.
22. How do you run SQL queries on a DataFrame in PySpark?
Ans:
To run SQL queries on a DataFrame in PySpark, you first create a temporary view of the DataFrame and then execute SQL queries on that view. For example:
- # Create a temporary view
- df.createOrReplaceTempView(“table_name”)
- # Run SQL query
- result = spark.sql(“SELECT * FROM table_name WHERE column > 10”)
23. What are UDFs (User Defined Functions) in PySpark? How do you create one?
Ans:
UDFs (User Defined Functions) in PySpark allow you to define custom functions to apply to DataFrame columns. You create UDFs using the `udf` function from `pyspark.sql.functions`. For example:
- from pyspark.sql.functions import udf
- from pyspark.sql.types import IntegerType
- # Define a Python function
- def square(x):
- return x * x
- # Create a UDF
- square_udf = udf(square, IntegerType())
- # Use the UDF
- df = df.with column(“squared”, square_udf(df[“column”]))
24. Explain the difference between createOrReplaceTempView and createGlobalTempView in PySpark.
Ans:
- In PySpark, `createOrReplaceTempView` creates a temporary view limited to the current SparkSession.
- This view is not accessible from other SparkSessions. `createGlobalTempView`, however, creates a global temporary view accessible across all SparkSessions within the application and persists until the Spark application terminates.
- The global temporary view is stored in an international database called `global_temp`.
25. How do you read data from external sources like JSON and CSV into PySpark DataFrame?
Ans:
To read data from external sources like JSON or CSV into a PySpark DataFrame, you use the `read` method of the `SparkSession` object. Examples:
- # Reading JSON
- df_json = spark.read.json(“path/to/json/file”)
- # Reading CSV
- df_csv = spark.read.csv(“path/to/csv/file”, header=True, inferSchema=True)
26. Explain the concept of partitioning in PySpark.
Ans:
Partitioning in PySpark involves dividing data into smaller, manageable chunks called partitions. Each partition is processed in parallel on different nodes of the cluster. Effective partitioning can significantly enhance the performance of Spark jobs by enabling efficient parallel processing and minimizing data shuffling. PySpark automatically partitions data when loading it, but you can also specify the number of partitions using methods like `repartition()` and `coalesce()`.
27. How do you optimize PySpark jobs for better performance?
Ans:
- To optimize PySpark jobs for better performance, you can:
- Ensure appropriate partitioning to balance data distribution and parallel processing.
- Cache or persist frequently accessed data to minimize recomputation.
- Broadcast small datasets to reduce data shuffling in joins.
- Utilize built-in functions instead of UDFs, as they are more optimized.
28. What is lazy evaluation in PySpark? Why is it important?
Ans:
- Lazy evaluation in PySpark means that transformations on RDDs and DataFrames are not executed immediately.
- Instead, they build up a logical plan of operations, which is executed only when an action is called.
- This approach is crucial because it optimizes the overall execution plan, reduces redundant computations, and enhances performance by scheduling operations more efficiently.
29. Explain the concept of Spark lineage.
Ans:
Spark lineage refers to the record of transformations applied to an RDD or DataFrame, which Spark uses to reconstruct lost data in case of a failure. Each transformation creates a new dataset, and Spark keeps track of these transformations to rebuild data if necessary. This lineage information ensures fault tolerance and data recovery without requiring checkpoints.
30. What are the ways to optimize PySpark shuffle operations?
Ans:
- Increase the number of shuffle partitions to distribute the load more evenly.
- Use the `repartition()` method to evenly redistribute data before shuffling.
- Broadcast small tables to avoid large shuffle operations during joins.
- Enable Tungsten optimization for better memory and CPU efficiency.
- Optimize data serialization by using efficient formats like Kryo.
- Minimize broad transformations when possible or optimize their logic to reduce data shuffling.
31. How can you avoid data skewness in PySpark?
Ans:
Avoiding data skewness in PySpark can be achieved through several methods:
- Salting: Add random noise to keys to distribute skewed data more evenly across partitions.
- Increase partition count: Distribute data across more partitions to mitigate the effects of skew.
- Broadcast joins: Use broadcast variables to manage small tables and minimize the shuffling of skewed data.
- Custom partitioning: Create custom partitioners to control how data is distributed based on specific criteria.
- Aggregation with combiner: Use combiners to reduce data size before shuffling.
32. What is a broadcast variable in PySpark? When do you use it?
Ans:
- A broadcast variable in PySpark is a read-only variable that is cached on each machine in the cluster rather than being sent with each task.
- It is used to efficiently distribute large data sets (that fit in memory) across the cluster, which helps reduce the amount of data shuffled during joins or other operations involving small datasets.
33. Explain the concept of window functions in PySpark.
Ans:
Window functions in PySpark allow you to perform calculations across a set of table rows related to the current row. They are helpful for tasks like ranking, cumulative sums, and moving averages. Window functions are defined using the `Window` class along with specifications for partitioning, ordering, and frame boundaries. For example:
- from pyspark.sql.window import Window
- from pyspark.sql.functions import rank
- window_spec = Window.partitionBy(“column1”).orderBy(“column2”)
- df.withColumn(“rank”, rank().over(window_spec))
34. How do you handle time-based data in PySpark?
Ans:
- Reading time-based data: Ensure timestamps are correctly parsed when loading data from sources like CSV or JSON.
- Using time functions: For date and time manipulation, utilize PySpark functions like `to_date`, `to_timestamp`, `date_add`, `date_sub`, etc.
- Time window operations: Apply window functions to perform operations over sliding time windows.
- Time zone handling: Manage time zones appropriately to maintain consistency in time-based data processing.
35. What are accumulators in PySpark? How do you use them?
Ans:
Accumulators in PySpark are variables used to aggregate information across tasks. They are initialized on the driver and updated by functions running on the cluster. Accumulators are helpful for counting or summing values across multiple nodes. Example of using an accumulator:
- from pyspark import SparkContext
- sc = SparkContext.getOrCreate()
- acc = sc.accumulator(0)
- def add_to_acc(x):
- acc.add(x)
- rdd = sc.parallelize([1, 2, 3, 4])
- rdd.foreach(add_to_acc)
- print(acc.value) # Output: 10
36. Explain the checkpointing mechanism in PySpark.
Ans:
Checkpointing in PySpark is a method to truncate the lineage of RDDs, aiding fault tolerance by saving the state of an RDD to reliable storage like HDFS. This is useful for long-running jobs or jobs with complex dependency chains. Checkpointing is implemented by calling `rdd.checkpoint()` after setting a checkpoint directory with `sc.setCheckpointDir(path)`.
37. What are the different ways to deploy PySpark applications?
Ans:
- Standalone mode: Using Spark’s built-in cluster manager.
- YARN: Running on Hadoop’s YARN cluster manager.
- Mesos: Running on Apache Mesos cluster manager.
- Kubernetes: Using Kubernetes for containerized deployments.
- Local mode: Running locally on a single machine, mainly for development and testing.
38. How do you handle schema evolution in PySpark?
Ans:
- Schema merging: Enable schema merging when reading data from sources like Parquet, which supports schema evolution.
- Explicit schema definition: Define the schema explicitly when reading data to handle changes.
- Casting and transformations: Use DataFrame transformations to adjust for schema changes by casting columns or adding/removing fields as necessary.
39. Explain the use case of repartition and coalesce in PySpark.
Ans:
- Repartition: Used to increase or decrease the number of partitions of an RDD or DataFrame. It performs a complete shuffle of the data, making it suitable for significantly changing the number of partitions.
- Coalesce: Used to decrease the number of partitions by combining partitions without a complete shuffle. It is more efficient than repartition when reducing the number of partitions because it avoids a complete data shuffle.
40. What is the significance of SparkContext in PySpark?
Ans:
The SparkContext is the entry point for any PySpark application. It represents the connection to the Spark cluster and is used to create RDDs, broadcast variables, and accumulators, as well as manage cluster resources. It provides methods to interact with other Spark components like Spark SQL, Spark Streaming, and MLlib. When you create a `SparkSession`, it automatically initializes a SparkContext internally.
41. What does PySpark support serialization formats?
Ans:
PySpark supports multiple serialization formats for efficient data storage and processing. These include Java Serialization (default but less efficient), Kryo Serialization (optimized for performance), Avro Serialization (compact and schema-based), Parquet Serialization (optimized for columnar storage and analytics), and ORC Serialization (similar to Parquet with different optimizations). The choice of serialization format depends on factors such as performance requirements, data size, and compatibility with existing systems.
42. Explain the difference between Parquet, Avro, and ORC file formats in PySpark.
Ans:
- Parquet is a columnar storage format designed for efficient analytics and data warehousing, supporting complex nested data structures and efficient compression.
- Avro is a compact binary format with schema evolution support that is suitable for data serialization and exchange between systems.
- ORC (Optimized Row Columnar) is another columnar storage format offering similar benefits to Parquet, optimized for fast reads and efficient compression.
- Each format has its strengths, making them suitable for different use cases based on performance, storage efficiency, and compatibility requirements.
43. How do you write data to external storage (like HDFS) in PySpark?
Ans:
- To write data to external storage such as HDFS in PySpark, you use the `write` method available on DataFrame or RDD objects.
- For instance, to save a DataFrame as Parquet files in HDFS, you would use `df.write.parquet(“hdfs://namenode/path/to/output”)`.
- PySpark handles the partitioning and distribution of data automatically, making it straightforward to store data in various formats (e.g., CSV, JSON, ORC) on HDFS or other supported filesystems.
44. What is the role of Hadoop InputFormat and OutputFormat in PySpark?
Ans:
Hadoop InputFormat and OutputFormat in PySpark define how to read data from and write data to Hadoop-compatible filesystems like HDFS. They encapsulate logic for reading input data in specific formats, managing splits for parallel processing, and formatting output data for storage. PySpark uses these formats internally to interact with Hadoop-based storage systems, ensuring compatibility with existing Hadoop ecosystem tools and efficient data processing capabilities.
45. How does PySpark integrate with Hive?
Ans:
PySpark integrates with Apache Hive, a data warehouse infrastructure built on Hadoop, through various mechanisms. This includes using the `HiveContext` in PySpark to interact with Hive metastore and execute HiveQL queries directly from PySpark. PySpark can read and write data in Hive tables using Hive’s SerDe (Serializer/Deserializer) for data serialization and deserialization. Additionally, PySpark supports Hive UDFs (User Defined Functions), allowing users to leverage existing Hive functions within PySpark SQL queries seamlessly.
46. Explain how PySpark can interact with streaming data sources like Kafka.
Ans:
- PySpark can interact with streaming data sources like Apache Kafka using its structured streaming API or the `spark-sql-kafka` library.
- By defining a Kafka source in the structured streaming API, PySpark can read data from Kafka topics as streaming DataFrames.
- This enables real-time processing and analysis of streaming data, where each record in the Kafka topic becomes a row in the DataFrame.
- PySpark’s integration with Kafka facilitates scalable and fault-tolerant stream processing, making it suitable for applications requiring real-time analytics and event-driven architectures.
47. What are the different ways to integrate PySpark with databases like MySQL or PostgreSQL?
Ans:
- Integrating PySpark with databases like MySQL or PostgreSQL involves using JDBC connectors.
- PySpark supports reading and writing data to/from databases using its DataFrame API with JDBC connectors.
- Developers can specify JDBC URL, database credentials, and query details to read data into PySpark DataFrames (`spark.read.jdbc`) or write DataFrames back to databases (`df.write.jdbc`).
- This approach leverages PySpark’s distributed computing capabilities for scalable data processing while integrating seamlessly with relational databases for data analytics, reporting, and data migration tasks.
48. How do you work with PySpark on a cluster manager like YARN or Mesos?
Ans:
To work with PySpark on cluster managers like YARN or Mesos, you configure Spark to connect to the cluster manager using `spark-submit` or `SparkSession` configuration settings. You specify the cluster mode (`yarn-client`, `yarn-cluster`, `mesos`, etc.) and allocate resources (e.g., cores, memory) using configuration parameters like `–executor-cores` and `–executor-memory`. PySpark abstracts the complexities of cluster management, allowing developers to submit PySpark applications (`spark-submit) to the cluster for distributed data processing.
49. What are common errors you encounter in PySpark jobs?
Ans:
- Memory-related issues (e.g., out-of-memory errors).
- Serialization problems (e.g., incompatible data types).
- Data skew (uneven distribution impacting performance).
- Dependency conflicts (e.g., library versions).
- Resource allocation challenges (under/over-allocation).
- Network issues (e.g., connectivity problems).
50. How do you debug PySpark applications?
Ans:
- Utilizing logging (`logger.info`, `logger. debug`) for outputting debug information.
- Monitoring Spark UI (`localhost:4040`) for job progress and stages.
- Inspecting driver and executor logs for errors or exceptions.
- Using interactive Python shells (`python) or debuggers (`pdb`) for interactive debugging sessions.
- Wrapping critical operations in try-except blocks to handle exceptions gracefully.
51. What is SparkUI, and how is it helpful in debugging PySpark jobs?
Ans:
SparkUI serves as a web-based interface provided by Apache Spark, enabling users to monitor the progress and details of Spark jobs. It plays a crucial role in debugging PySpark jobs by offering insights into job execution metrics such as task statuses, stage details, executor memory usage, and input/output statistics. SparkUI helps pinpoint performance bottlenecks, understand job dependencies, and optimize resource allocation.
52. How do you handle out-of-memory (OOM) errors in PySpark?
Ans:
Handling Out Of Memory (OOM) errors in PySpark involves adopting various strategies to optimize memory usage and improve job stability. One approach is to adjust executor memory allocation using the `–executor-memory` parameter during job submission. Optimizing data partitioning across executors helps distribute workload evenly and alleviates memory pressure. Utilizing caching (`cache()`, `persist()`) for intermediate DataFrames or RDDs can reduce recomputation and enhance performance.
53. How do you perform feature engineering in PySpark?
Ans:
- Feature engineering in PySpark involves transforming raw data into meaningful features suitable for machine learning models.
- This process includes tasks such as data cleaning to handle missing values (`fillna()`), outliers, and normalization (`StandardScaler`).
- Categorical features are typically encoded using techniques like one-hot encoding (`OneHotEncoder`) or indexed encoding (`StringIndexer`).
- New features may be derived based on domain knowledge or statistical operations.
54. Explain the lifecycle of a machine learning model in PySpark.
Ans:
The lifecycle of a machine learning model in PySpark encompasses several stages, beginning with data preparation, where raw data is loaded and preprocessed using PySpark DataFrame operations. Feature engineering transforms data into suitable features for model training, followed by model selection involving the choice of an algorithm (`pyspark. ml`) and training it using methods like `fit()`. The evaluation assesses model performance using metrics (`RegressionEvaluator`, `BinaryClassificationEvaluator`).
55. What are the steps involved in building a machine learning pipeline in PySpark?
Ans:
Constructing a machine learning pipeline in PySpark involves:
- Defining stages for data preprocessing (`VectorAssembler`, `StringIndexer`).
- Assembling these stages into a `Pipeline`.
- Fitting the pipeline to training data.
Data transformation through the pipeline generates features used to train a machine learning model (`LogisticRegression`, `RandomForest`). Model performance is evaluated using metrics (`Evaluator` classes), and hyperparameter tuning (`ParamGridBuilder`, `CrossValidator`) optimizes model parameters. Trained pipelines are deployed for predictions (`transform()`).
56. How do you evaluate model performance in PySpark?
Ans:
Evaluating model performance in PySpark involves selecting appropriate evaluation metrics (`RegressionEvaluator`, `BinaryClassificationEvaluator`) based on the specific machine learning task, such as regression or classification. Evaluators compare model predictions against actual values on test data using methods like `evaluate()`. Cross-validation (`CrossValidator`) ensures robust evaluation by partitioning data into multiple folds for training and validation.
57. How do you write unit tests for PySpark applications?
Ans:
Writing unit tests for PySpark applications involves using testing frameworks (`playtest, `unit test`) to validate DataFrame transformations (`assertDataFrameEqual`) against expected outputs. Mocking Spark context and sessions isolates tests from cluster dependencies. Testing custom functions and transformations ensures functional correctness. Setup and teardown methods initialize and clean up Spark sessions (`spark session. stop()`).
58. What are the best practices for maintaining a PySpark codebase?
Ans:
- Modularizing code into reusable functions and modules.
- Extensively documenting code for clarity and future reference.
- Conducting code reviews to uphold quality standards.
- Using version control systems (e.g., Git) for change management.
- Optimizing performance through efficient data processing and resource allocation.
- Implementing robust error handling and logging mechanisms.
59. How do you monitor PySpark jobs in production?
Ans:
- Monitoring PySpark jobs in production involves utilizing SparkUI to track job progress, executor metrics, and task performance.
- Logging (`log4j`, `logging` module) captures job-specific information, errors, and warnings.
- Monitoring tools (e.g., Prometheus, Grafana) collect and visualize Spark job metrics such as CPU usage, memory utilization, and shuffle data.
- Alerts (e.g., Slack, email notifications) notify critical job failures or performance degradation.
60. How do you secure PySpark applications?
Ans:
Securing PySpark applications involves implementing encryption (SSL/TLS) for secure communication between Spark components, user authentication using mechanisms like Kerberos or LDAP, role-based access control (RBAC) to restrict data access, secure configuration management (e.g., encrypted credentials), enabling audit logging to track user activities and configurations, and deploying applications in safe environments (e.g., containerization with Docker, Kubernetes).
61. What authentication mechanisms does PySpark support?
Ans:
PySpark supports several authentication mechanisms that are primarily integrated with underlying cluster managers like YARN or Mesos. The most commonly used mechanisms include Kerberos, which provides strong authentication through secret-key cryptography, ensuring secure client-server interactions within the cluster environment. LDAP (Lightweight Directory Access Protocol) is another option that offers centralized authentication and authorization services.
62. Explain the concept of serialization in PySpark.
Ans:
- Serialization in PySpark refers to the process of converting data structures or objects into a format that can be easily transported or stored, typically for distributed processing.
- In PySpark, serialization plays a crucial role in efficiently transmitting data between nodes in a cluster.
- PySpark supports various serialization formats such as Pickle, JSON, Parquet, Avro, and ORC, each suited for different use cases based on factors like data size, performance requirements, and interoperability with other systems.
63. What are the advantages and disadvantages of using different serialization formats in PySpark?
Ans:
- Different serialization formats in PySpark offer distinct advantages and disadvantages based on their characteristics and intended use cases.
- Pickle serialization provides flexibility in serializing Python objects but may not be as efficient or interoperable as other formats like JSON or Parquet.
- JSON serialization offers human-readability and interoperability benefits across different platforms but may result in larger file sizes and slower serialization/deserialization processes compared to binary formats.
64. How do you manage resources in PySpark?
Ans:
Managing resources in PySpark involves optimizing CPU, memory, and network usage to ensure efficient execution of distributed data processing tasks across a cluster. Memory management is critical, where configuring memory allocation for executors (`spark.executor.memory`), driver (`spark.driver.memory`), and managing memory fractions (`spark.memory.fraction`) are essential for preventing out-of-memory errors and maximizing performance.
65. What are the best practices for optimizing PySpark jobs?
Ans:
- Data Partitioning: Properly partition data using `repartition()` or `coalesce()` to optimize parallelism and reduce shuffle operations.
- Caching and Persistence: Cache intermediate results (`cache()` or `persist()`) in memory to avoid recomputation and expedite iterative algorithms.
- Use of Broadcast Variables: Use’ broadcast () ‘ to broadcast read-only variables to all nodes to minimize data transfer overhead during join operations.
- Memory Management: Tune memory settings (`spark.executor.memory`, `spark.driver.memory`) based on workload characteristics and cluster resources.
66. How do you configure a PySpark cluster?
Ans:
- Cluster Manager: Specify the cluster manager (`spark.master`) such as `yarn`, `mesos`, or `local` for local testing.
- Executor Memory and Cores: Configure executor memory (`spark.executor.memory`) and cores (`spark.executor.cores`) based on workload requirements and cluster capacity.
- Driver Memory: Set driver memory (`spark.driver.memory`) to allocate sufficient resources for the Spark driver to execute tasks and manage job orchestration.
- Resource Allocation: Adjust parameters like `spark.executor.instances`, `spark.executor.memoryOverhead`, and `spark.driver.memoryOverhead` to optimize resource utilization and prevent resource contention.
67. What parameters are necessary to tune in Spark configuration for optimal performance?
Ans:
- Memory Management: Configure memory settings such as `spark.executor.memory`, `spark.driver.memory`, and `spark.memory.fraction` to balance memory allocation between executors and the driver, preventing out-of-memory errors and improving performance.
- Concurrency and Parallelism: Adjust parameters like `spark.executor.cores`, `spark.task.cpus`, and `spark.default.parallelism` to optimize task scheduling and parallel execution based on CPU core availability and workload characteristics.
- Shuffle Tuning: Optimize shuffle operations using parameters like `spark.shuffle.file.buffer`, `spark.reducer.maxSizeInFlight`, and `spark.shuffle.io.maxRetries` to minimize data movement and enhance performance during data shuffling and aggregation tasks.
68. How do you determine the optimal number of partitions in a PySpark data frame?
Ans:
The optimal number of partitions in a PySpark DataFrame is determined based on factors like data size, cluster resources, and workload characteristics. A general guideline is to start with partitions that are 2-4 times the number of CPU cores available in your cluster. You can check the current number of partitions using `df.rdd.getNumPartitions()` and adjust them using `df.repartition(num_partitions)` or `df.coalesce(num_partitions)` as needed.
69. What strategies would you use to scale PySpark jobs?
Ans:
- Horizontal Scaling: Increase the number of executor instances (`spark.executor.instances`) to distribute workload across more nodes and enhance parallelism, accommodating larger datasets and processing requirements.
- Dynamic Resource Allocation: Enable dynamic allocation (`spark.dynamicAllocation.enabled`) to scale executor instances dynamically based on workload demand, optimizing resource
71. How do you deploy PySpark applications in a production environment?
Ans:
- Build Application JAR: Package PySpark application code into an executable JAR file using build tools like `sbt` or `Maven`, including all dependencies and configurations required for execution.
- Configure Cluster: Based on workload requirements and organizational policies, set up the Spark cluster environment (e.g., YARN, Mesos, standalone) with appropriate resources (executors, memory, cores) and security settings (authentication, encryption).
- Submit Application: Use the `spark-submit` command to submit the packaged JAR file along with Spark configurations (`–master`, `–deploy-mode`, `–executor-memory`, etc.) to initialize the application driver and executors on the cluster.
72. What is the role of executors in a Spark application?
Ans:
Executors in a Spark application are responsible for executing tasks on worker nodes within the cluster. They are launched by the Spark Context (or SparkSession in PySpark) and are allocated resources such as CPU cores and memory from the cluster manager (e.g., YARN, Mesos, Kubernetes). Executors run computations and store data for RDDs or DataFrames that are cached in memory or persisted on disk.
73. How does task scheduling work in Spark?
Ans:
Task scheduling in Spark orchestrates the execution of tasks across executors in a distributed environment. When a Spark application is submitted, the driver program divides the job into stages consisting of tasks. These tasks are scheduled to run on executors based on data locality and available resources. Spark employs a DAG (Directed Acyclic Graph) scheduler to optimize the task execution order and minimize data shuffling.
74. Compare and contrast RDD, DataFrame, and Dataset APIs in PySpark.
Ans:
RDD (Resilient Distributed Dataset):
- RDD is the foundational data abstraction in Spark, representing a distributed collection of objects.
- RDDs are immutable and can contain any Python, Java, or Scala objects.
DataFrame:
- A data frame is a distributed collection of data organized into named columns, similar to a table in a relational database.
- DataFrames are built on top of RDDs but provide higher-level
Dataset:
- Dataset API combines the benefits of RDDs and DataFrames, offering type-safe, object-oriented programming interfaces in Python, Java, or Scala.
- Datasets provide compile-time type safety and leverage Spark’s Catalyst optimizer for efficient execution.
75. How do you convert between RDD and DataFrame in PySpark?
Ans:
RDD to DataFrame: Convert an RDD to a DataFrame using the `toDF()` method on the RDD. For example:
- rdd = sc.parallelize([(1, ‘Alice’), (2, ‘Bob’)])
- df = rdd.toDF([‘id’, ‘name’])
DataFrame to RDD: Convert a DataFrame to an RDD using the `rdd()` method on the DataFrame. For example:
- df = Spark.createDataFrame([(1, ‘Alice’), (2, ‘Bob’)], [‘id’, ‘name’])
- rdd = df. rdd
76. Explain the differences between various serialization formats used in PySpark.
Ans:
Java Serialization:
- Platform-independent serialization format.
- Supports complex object graphs and circular references.
- May result in slower serialization/deserialization speeds and larger serialized data sizes.
Kryo Serialization:
- Fast and compact serialization format.
- Provides quicker serialization/deserialization speeds and smaller serialized data sizes.
- Requires explicit registration of custom classes and may have limitations with complex object graphs.
77. What are the key configurations you would consider for optimizing PySpark jobs?
Ans:
- Memory Management: Configure executor memory (`spark.executor.memory`) and memory overhead (`spark.executor.memoryOverhead`).
- CPU Cores: Allocate executor cores (`spark.executor.cores`) based on workload requirements.
- Shuffle Partitions: Adjust shuffle partitions (`spark.sql.shuffle.partitions`) to optimize data shuffling operations.
- Serialization: Choose the serialization format (`spark. serializer` or `spark.kryo.registrator`) based on performance needs.
78. DataFrame Caching and Persistence in PySpark:
Ans:
DataFrame caching and persistence in PySpark are techniques employed to enhance performance by storing DataFrames in memory or disk across Spark jobs. Caching is achieved using methods like `cache()` or `persist()` on DataFrames, allowing them to be stored in memory with various storage levels (`MEMORY_ONLY`, `MEMORY_AND_DISK`, etc.) based on memory availability and performance requirements.
79. What are the critical features of PySpark MLlib?
Ans:
- Scalability: Distributed algorithms for large-scale data processing on Spark clusters.
- ML Algorithms: Classification, regression, clustering, collaborative filtering, and dimensionality reduction algorithms.
- Pipeline API: High-level API for constructing ML workflows with feature transformers, estimators, and evaluators.
- Model Selection: Tools for hyperparameter tuning, cross-validation, and model evaluation.
80. How do you build a machine learning pipeline in PySpark?
Ans:
- Data Preparation: Load data from sources like CSV or JSON into DataFrames using `spark.read` methods.
- Feature Engineering: Transform and preprocess data using DataFrame API for feature extraction and transformation.
- Pipeline Construction: Using the’ Pipeline’ and’ PipelineStage’ classes, define a pipeline with stages (transformers, estimators).
- Model Training: Fit the pipeline to training data using the `Pipeline.fit()` method, which sequentially applies defined stages.
81. Handling Data Skewness in PySpark:
Ans:
Addressing data skewness in PySpark is critical for optimizing job performance by ensuring even distribution of workload across Spark executors. Strategies to mitigate skewness include optimizing data partitioning using methods like `repartition(bucket bucket ()` during data ingestion to distribute data evenly across partitions. Sampling techniques such as `sample()` help identify skewed partitions for targeted adjustments.
82. Types of Joins in PySpark DataFrames:
Ans:
PySpark supports various types of joins to merge data from different data frames based on specified conditions. These include inner joins, which return rows with matching keys in both DataFrames; outer joins (full joins), which return all rows from both DataFrames along with nulls where there is no match; left joins, returning all rows from the left DataFrame and matching rows from the right DataFrame; and right joins, which do the reverse.
83. How do you handle different data sources (e.g., CSV, JSON, Parquet) in PySpark?
Ans:
- CSV: Read CSV files using `spark.read.csv()` and specify options such as delimiter and header.
- JSON: Read JSON files using `spark.read.json()` with options for multi-line, sampling, and schema inference.
- Parquet: Read Parquet files using `spark.read.parquet()` for efficient columnar storage with schema preservation.
- Other Formats: Handle formats like ORC, Avro, JDBC, and Hive tables using respective read methods (`spark.read.format()`).
84. Memory Management in PySpark:
Ans:
Efficient memory management in PySpark is essential for maximizing resource utilization across Spark executors within distributed computing environments. Executors manage JVM heap memory allocated by the cluster manager (`spark.executor.memory`) and utilize off-heap memory (`spark.memory.offHeap.size`) for internal Spark data structures. Key memory components include storage memory for caching RDDs or DataFrames (`spark.memory.storageFraction`) and execution memory for task processing and shuffle operations (`spark.memory.fraction`, `spark.memory.shuffleFraction`).
85. Role of Garbage Collection in PySpark:
Ans:
Garbage collection (GC) in PySpark manages memory allocation and reclamation within Spark executors, ensuring efficient resource utilization and application performance. As Spark tasks execute within JVM heap memory, GC processes actively reclaim memory occupied by unreferenced objects, preventing memory leaks and optimizing memory usage. Effective GC configuration (`Spark.executor.extraJavaOptions`) involves tuning parameters to balance GC overhead and application responsiveness, aligning with workload demands.
86. Creating an RDD in PySpark:
Ans:
- In PySpark, Resilient Distributed Datasets (RDDs) are fundamental for distributed data processing and can be created using various methods.
- One common approach is to parallelize a local collection (e.g., Python list) into an RDD using `sc.parallelize()` from the SparkContext (`sc`).
- For example, `sc.parallelize([(1, ‘Alice’), (2, ‘Bob’)])` converts a Python list into an RDD where each element is a tuple.
- Alternatively, data can be loaded from external storage such as HDFS or S3 using methods like `sc.textFile()` or `sc.sequenceFile()`, transforming data into RDDs.
87. Commonly Used DataFrame Operations in PySpark:
Ans:
- PySpark DataFrames provide a versatile set of operations for efficient data manipulation and analysis within distributed computing environments.
- Key operations include filtering rows based on conditions (`filter()`, `where()`), selecting specific columns (`select()`, `selectExpr()`), aggregating data using grouping operations (`groupBy()`, `agg()`), sorting rows (`orderBy()`, `sort()`), and merging DataFrames (`join()`, `crossJoin()`).
- Additional functionalities encompass window functions for advanced analytics (`over()`, `rank()`), pivoting data (`pivot()`), handling missing values (`na.drop()`, `na.fill()`), and type conversion (`cast()`, `withColumn()`).
88. Running SQL Queries in PySpark:
Ans:
Executing SQL queries on DataFrames in PySpark facilitates declarative and SQL-like data manipulation and analysis. Begin by registering DataFrames as temporary views using `createOrReplaceTempView()` or `createTempView()`, making them accessible for querying within Spark SQL. For instance, `df.createOrReplaceTempView(‘people’)` creates a temporary view named ‘people’. Execute SQL queries using the `spark.sql()` interface, passing SQL statements as strings.
89. Creating and Using UDFs in PySpark:
Ans:
User Defined Functions (UDFs) extend PySpark DataFrame APIs with custom Python functions for specialized data transformations and computations. Define UDFs by annotating Python functions with the `udf()` decorator from `pyspark.sql.functions`. For example, `@udf` decorates a function to square input values. Register UDFs to apply functions on DataFrame columns using `withColumn()`.
90. Spark Executors and Their Role in a Spark Application:
Ans:
Spark executors are worker processes responsible for executing tasks on worker nodes within a Spark cluster, managed by the SparkContext or SparkSession. Executors allocate CPU cores and memory (`spark.executor.memory`) from the cluster manager (e.g., YARN, Mesos) for task execution and data processing. They communicate with the driver program, receiving task assignments and executing transformations and actions on distributed datasets (RDDs or DataFrames).