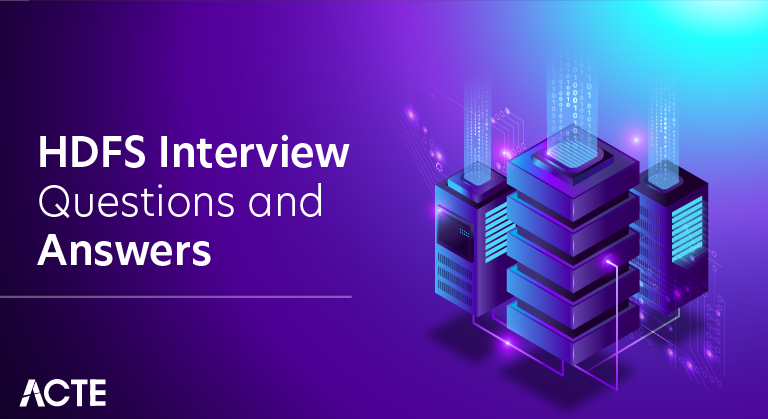
35+ POPULAR Hadoop [HDFS] Interview Questions & Answers
Last updated on 03rd Jul 2020, Blog, Interview Questions
HDFS is a file system that is distributed, scalable, and fault-tolerant designed for big data storage and processing. It serves as a fundamental part of the Apache Hadoop ecosystem. HDFS breaks large files into blocks, replicates them across a cluster of commodity hardware (DataNodes), and uses a single, centralized server (NameNode) to manage metadata and coordinate data access. HDFS is appropriate for big data analytics and processing workloads because it is optimised for handling massive data sets and provides high throughput, data redundancy, and resistance to hardware failures.
1. Describe HDFS.
Ans:
An open-source file system called Hadoop Distributed File System is a distributed storage system widely used in big data processing. It breaks large files into smaller blocks, stores them across a cluster of machines, and replicates data for fault tolerance. HDFS is a key component of the Hadoop ecosystem, enabling the storage and efficient processing of massive datasets across distributed computing environments.
2. How does Hadoop work?
Ans:
Hadoop operates on a distributed computing model for processing large datasets. It stores data in the Hadoop Distributed File System (HDFS), breaking it into blocks and replicating them across a cluster for fault tolerance. Data processing is achieved through the MapReduce programming model, enabling parallel execution of tasks across multiple nodes.
3. Compare HDFS and HBase.
Ans:
Aspect | HDFS | HBase | |
Primary Use |
Storage of large files, batch processing |
Real-time, random access to structured data | |
Data Model | File-based | Column-family-based (NoSQL) | |
Schema Flexibility | Schema-on-read | Schema-on-write | |
Data Size |
Suited for storing large files |
Suited for smaller, real-time data |
Ans:
Hadoop is an open-source framework and software ecosystem, which means it is not provided by a single commercial entity. Instead, it is developed and maintained by a global community of contributors. The Apache Software Foundation (ASF) is the organization that oversees the development of Hadoop and many other open-source projects.
5. What purpose does Hadoop serve?Ans:
Big Data Storage: Efficiently stores massive datasets using HDFS.
Data Processing: Analyzes data at scale with MapReduce.
Scalability: Scales horizontally for growing data volumes.
Fault Tolerance: Ensures data reliability and system availability.
Real-time and Batch Processing: Supports both processing modes.
6. What are the operating systems that Hadoop runs on?Ans:
- Linux
- Windows
- macOS
Hadoop is designed to be platform-independent and can run on various operating systems. However, it is primarily developed and tested on Linux-based operating systems. The most commonly used operating systems for running Hadoop clusters are:
Ans:
“Big Data” refers to the vast and complex volume of structured and unstructured data that exceeds the capabilities of traditional data processing systems.
It encompasses massive datasets with characteristics such as high volume, velocity (rapid generation), and variety (diverse data types).
8. Can you provide some Big Data examples?Ans:
- Social Media Analytics
- E-commerce Recommendations
- Financial Fraud Detection
- Healthcare Analytics
- Log and Event Analysis
9. What are the main traits of big data?
Ans:
The main traits of big data, commonly known as the “3 Vs”:
Volume: Big data entails massive volumes of data.
Velocity: Data is generated rapidly in real-time or near-real-time.
Variety: Data comes in diverse formats, including structured, semi-structured, and unstructured data.
10. What use does big data analysis serve for businesses?Ans:
Big data analysis plays a pivotal role in shaping businesses by providing valuable insights and advantages. It enables data-driven decision-making by uncovering patterns, trends, and customer preferences within vast datasets. This, in turn, leads to enhanced operational efficiency, improved customer experiences, and cost reductions.
11. What core characteristics does Hadoop have?Ans:
- Distributed Storage
- Parallel Processing
- Scalability
- Fault Tolerance
- Versatile Ecosystem
Ans:
Hadoop is well-suited for handling both structured and unstructured data without requiring a predefined schema, offering flexibility in managing various data formats. In contrast, RDBMS systems are optimized for structured data with fixed schemas, making them less adaptable to unstructured or semi-structured data.
13. Who are the leading web users of Hadoop?Ans:
- Amazon
Some prominent web users of Hadoop include:
Ans:
Distributed and Scalable: HDFS stores data across a cluster, scaling by adding nodes.
Fault Tolerance: It replicates data for high reliability and fault tolerance.
High Throughput: Optimized for fast data read and write operations.
Write-Once, Read-Many: Data is typically written once and read multiple times.
Data Locality: Minimizes data transfer by placing data close to processing.
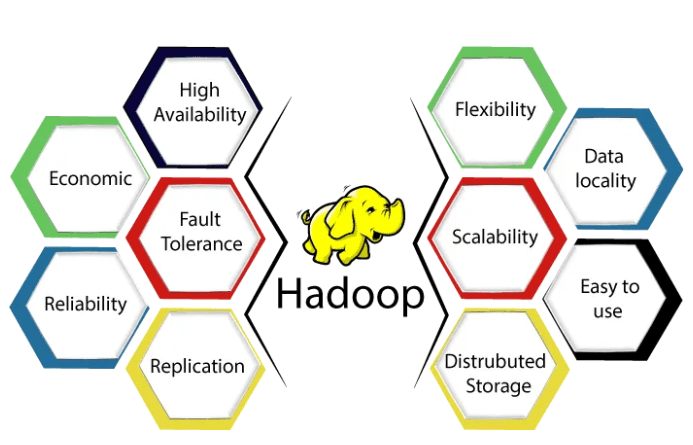
Ans:
HDFS intentionally replicates data, despite potential redundancy, to ensure fault tolerance, maintain data locality for efficient processing, enable parallel data operations, and provide quick recovery from node failures. This design choice enhances system reliability and performance, justifying the redundancy in storage.
16. What does “streaming access” mean?Ans:
“Streaming access” refers to the ability to continuously and efficiently read and process data as it is being generated or transmitted in real-time, without the need to download the entire dataset before processing begins. Streaming access is commonly used in scenarios where data is produced at a high rate and needs to be processed on-the-fly, often in a sequential manner.
17. Would the HDFS replicate the computations performed on one node to other nodes?
Ans:
No, HDFS does not replicate the computations performed on one node to other nodes. HDFS is primarily responsible for the storage and management of data. When calculations in a Hadoop cluster are carried out using MapReduce or other processing frameworks, the data is broken up into smaller chunks and sent to several nodes in parallel.
18. What does the term “commodity hardware” mean?Ans:
“Commodity hardware” refers to standard, off-the-shelf computer hardware components and equipment that are widely available in the market from various manufacturers. These components are often inexpensive, mass-produced, and not specialised or custom-built for specific uses.
19. Which HDFS node serves as the master?Ans:
In HDFS, the master node is known as the “NameNode.” The NameNode manages the file system’s metadata and namespace, keeping track of file structures and data block locations. It is crucial in organizing data storage and retrieval inside the Hadoop cluster.
20. What does “Data node” mean?Ans:
A “DataNode” in HDFS is a key component responsible for storing and managing the actual data blocks of files within the Hadoop cluster. DataNodes collaborate with the NameNode to guarantee data storage, replication, and fault tolerance, making them critical in dispersed situations for data availability and dependability.
Ans:
A daemon is a background process or service that runs on a computer or server, often without direct user interaction. Daemons perform various tasks, such as managing hardware devices, handling network requests, or executing system maintenance processes. They typically run continuously, providing essential functionality for the operating system or specific applications.
22. What does ‘job tracker’ do?Ans:
Its primary functions include scheduling, task assignment, and progress monitoring. It efficiently allocates cluster resources to execute tasks while maintaining fault tolerance through task recovery and job rescheduling. The Job Tracker ensures that MapReduce jobs are executed in a coordinated and efficient manner.
23. What function do task trackers serve?Ans:
Task Execution: Execute map and reduce tasks.
Progress Reporting: Report task progress.
Data Locality: Optimize data processing by prioritizing local data.
Task Recovery: Support task recovery in case of failure.
Resource Management: Efficiently manage CPU and memory resources.
24. What does “heartbeat” in HDFS mean?Ans:
In HDFS (Hadoop Distributed File System), a “heartbeat” is a regular signal or status update sent by DataNodes to the NameNode. These heartbeats serve as a way for DataNodes to communicate their current health and availability to the NameNode. Heartbeats are a critical mechanism for ensuring the proper functioning and fault tolerance of HDFS.
25. What is the HDFS indexing procedure?Ans:
HDFS (Hadoop Distributed File System) does not provide built-in indexing procedures. To enable indexing and efficient data retrieval, organizations often use higher-level tools like HBase or Apache Solr, which are integrated with Hadoop and designed for indexing and search capabilities. These tools allow users to query and retrieve data efficiently based on specific use cases.
26. What does ‘block’ in HDFS mean?Ans:
In HDFS, a “block” refers to a fixed-size unit into which a large file is divided for storage. The default block size in HDFS is typically 128 megabytes or 256 megabytes, although it can be customized. Each block is an independent unit of storage that is distributed across the nodes of the Hadoop cluster.
27. Is it required to have the Name node and the task tracker on the same host?Ans:
No, it is not required to have the NameNode and the Task Tracker on the same host in Hadoop. In fact, it is common practice to have them on separate nodes in a Hadoop cluster. Separating the NameNode from Task Trackers helps distribute the workload and ensures better fault tolerance.
28. How is a data node detected as saturated?Ans:
A data node in Hadoop’s HDFS is considered saturated when it approaches its storage capacity limit or experiences high resource utilization. Detection involves monitoring storage capacity, disk I/O, CPU and memory usage, network bandwidth, and DataNode health checks. Custom monitoring scripts or third-party solutions can also be used.
29. What kind of data is handled by Hadoop?Ans:
- Structured Data
- Unstructured Data
- Semi-structured Data
- Multimedia Data
- Time Series Data
Ans:
In Hadoop’s HDFS, the NameNode is responsible for managing the metadata of the file system, including the locations of data blocks. When a client wants to write data, it contacts the NameNode to request a list of available DataNodes.
The client then chooses a DataNode based on criteria like proximity, network stability, and data distribution and writes the data directly to that DataNode.
31. What is the ‘user’ in HDFS?
Ans:
In Hadoop’s HDFS, a “user” refers to an entity or an individual who interacts with the Hadoop cluster. Users are often individuals or applications that utilize Hadoop to do different tasks such as reading and writing data, performing MapReduce jobs, and maintaining HDFS files and directories.
32. How does the client interface with the Name and Data nodes in HDFS?
Ans:
NameNode Interaction: Client requests file metadata from NameNode.
Data Retrieval: Client communicates with DataNodes for data retrieval.
Data Storage: For writing, client sends data to selected DataNodes.
33. What does an HDFS rack mean?
Ans:
A rack represents a group of interconnected data nodes within a data center. It is a method of organizing nodes based on physical proximity and network connectivity. Rack awareness enhances fault tolerance by separating data copies to reduce the chance of rack-level failures.
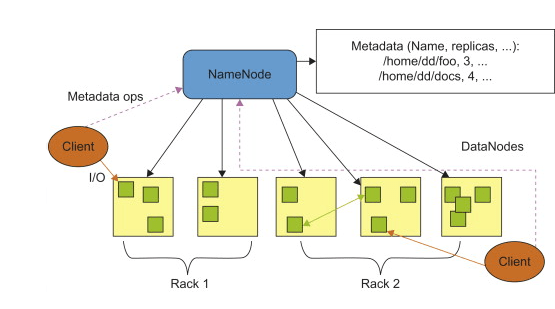
34. What distinguishes HDFS from a standard filesystem?
Ans:
Aspect | HDFS (Hadoop Distributed File System) | Standard Filesystem |
Scalability |
Highly scalable, distributed across multiple nodes |
Typically limited by the capacity of a single server |
Fault Tolerance | Fault-tolerant through data replication | Vulnerable to data loss in case of hardware failures |
Data Distribution | Optimized for distributed computing and big data applications | Not inherently optimized for distributed computing |
35. Why is HDFS tolerant of faults?
Ans:
HDFS exhibits fault tolerance primarily through data replication and self-healing mechanisms. It replicates data across multiple nodes, ensuring redundancy. In case of hardware failures or unresponsive nodes, HDFS can seamlessly access data from its replicas.
36. Describe the HDFS architecture.
Ans:
NameNode: Manages metadata and file structure, but is a single point of failure.
DataNode: Stores data blocks, communicates with NameNode, and handles replication.
Blocks: Fixed-size units of data, replicated for fault tolerance.
Checkpointing & Backup: Improve fault tolerance through metadata maintenance and failover support.
Client: Interacts with HDFS for metadata and data operations.
37. What two kinds of metadata do NameNode servers store?
Ans:
Filesystem Namespace Metadata: This type of metadata includes information about the file and directory structure within HDFS. It tracks details such as file names, directory structure, permissions, and timestamps.
Block Location Metadata: This metadata keeps track of the physical location of data blocks within the cluster. It records which DataNodes (worker nodes) hold copies of each data block.
38. What distinguishes federation from high availability?
Ans:
HDFS Federation scales namespace and metadata management by allowing multiple independent namespaces within one cluster. It enhances efficiency but doesn’t guarantee NameNode fault tolerance. In contrast, High Availability (HA) ensures continuous access by eliminating a single point of failure with Active and Standby NameNodes, providing fault tolerance and uninterrupted data availability.
39. How does HDFS’s rack awareness function?
Ans:
Rack Information: HDFS knows the physical network layout, including racks and DataNodes.
Replication Strategy: When storing data, HDFS spreads replicas across different racks for fault tolerance.
Data Locality: It aims to read data from nearby racks to minimize network traffic and improve access speed.
Rebalancing: Periodically, HDFS may move data blocks to maintain resource balance across racks.
40. What would happen if too many little files were kept together in a cluster on HDFS?
Ans:
Storing too many small files in a cluster on HDFS can lead to inefficient resource utilization and increased metadata overhead. Each file incurs its own metadata entry, straining the NameNode and consuming excessive memory. This can degrade HDFS performance and hinder overall cluster scalability.
41. How may data from the local system be copied onto HDFS?
Ans:
To copy data from a local system to Hadoop Distributed File System, you can use the ‘hadoop fs’ command-line tool with the ‘copyFromLocal’ command. Alternatively, you can use programming APIs (e.g., Java, Python) to interact with HDFS and transfer data programmatically. Tools like Sqoop and Flume are also handy for importing external data into HDFS for big data processing.
42. What crucial setup settings are necessary for a MapReduce program?
Ans:
Setting up a MapReduce program in Hadoop involves key steps. First, define input and output data locations. Then, specify Mapper and Reducer classes for data processing. Determine input and output data formats, optionally use a combiner and partitioner, set reducer count, and provide the JAR file with custom configurations for efficient execution within the Hadoop cluster.
43. What is the block size for HDFS?
Ans:
The default block size for Hadoop Distributed File System (HDFS) is typically 128 MB or 256 MB, although it can be configured to different values depending on the Hadoop distribution and cluster configuration.
44. What are all the HDFS commands?
Ans:
hadoop fs -ls: List the files and folders in HDFS.
hadoop fs -mkdir: Create a directory in HDFS.
hadoop fs -copyFromLocal: Copy files from the local file system to HDFS.
hadoop fs -copyToLocal: Copy files from HDFS to the local file system.
hadoop fs -mv: Move or rename files and directories in HDFS.
45. What is the replication factor by default?
Ans:
The default replication factor in Hadoop’s HDFS is 3. This means that each data block is replicated three times by default, providing fault tolerance and data redundancy in the cluster. However, this replication factor can be configured to a different value based on the specific needs of the Hadoop cluster.
46. What differences exist between the NAS (Network Attached Storage) and HDFS (Hadoop Distributed File System)?
Ans:
NAS (Network Attached Storage):
NAS is a traditional storage system designed for file-level storage. It uses a hierarchical file structure and isn’t inherently scalable, making it less suitable for big data storage and processing.
HDFS (Hadoop Distributed File System):
HDFS is a distributed file system specifically designed for big data. It stores data in fixed-size blocks across a cluster of machines, providing high scalability and fault tolerance.
47. How do I maintain a balanced HDFS cluster?
Ans:
To maintain a balanced HDFS cluster, ensure an appropriate block size to control data distribution. Monitor data distribution regularly, add or decommission DataNodes as needed, and use HDFS’s balancer utility to redistribute data. Implement rack awareness, leverage HDFS Federation, and schedule routine maintenance tasks to optimize data distribution and performance.
48. How does HDFS Federation work?
Ans:
HDFS Federation is an enhancement to the Hadoop Distributed File System (HDFS) that improves its scalability and manageability. It achieves this by partitioning the file system’s namespace into multiple independent namespaces, each governed by its dedicated NameNode.
49. Why is HDFS High Availability important?
Ans:
HDFS High Availability (HA) is vital for continuous, reliable data access, minimizing downtime, and enhancing fault tolerance in Hadoop clusters. It’s crucial for mission-critical applications, operational efficiency, and scaling Hadoop environments while ensuring data integrity.
50. What does “rack-aware replica placement policy” mean?
Ans:
A “rack-aware replica placement policy” is a strategy used in distributed file systems like Hadoop’s HDFS to optimize data placement. It ensures that replicas of data blocks are stored across different racks within a data center to improve fault tolerance. This decreases the possibility of data loss as a result of rack-level failures and enhances data availability.
51. What does a DataNode block scanner perform?
Ans:
Periodic Scanning: Conducts regular scans of data blocks on local DataNodes.
Checksum Verification: Verifies block health by comparing computed checksums with stored metadata.
Reporting: Reports corrupt blocks to the NameNode for swift action.
Corruption Handling: Enhances overall data reliability by proactively detecting and addressing corruption or hardware issues in Hadoop’s HDFS.
52. How does a client application communicate with the NameNode?
Ans:
A client application communicates with the NameNode in Hadoop’s HDFS (Hadoop Distributed File System) through a network protocol.
It sends requests to the NameNode for operations like file read, write, or metadata retrieval.
53. What are NameNode’s primary features?
Ans:
- Metadata Management
- Block Management
- Client Coordination
- Fault Tolerance
- Heartbeat and Block Reports
54. What does the administrative tool serve?
Ans:
Administrative tools in Hadoop serve as essential utilities for managing and maintaining Hadoop clusters efficiently. They provide capabilities for monitoring cluster health, resource allocation, security configuration, and performance tuning. These tools empower administrators to ensure smooth cluster operation, optimize performance, and enhance security while simplifying cluster management tasks.
55. How does HDFS handle large files?
Ans:
- Data Block Division
- Data Replication
- Parallel Processing
- Efficient Replication
- Streaming Read/Write
- Block Size Optimization
HDFS efficiently handles large files through these strategies:
56. What is the purpose of the Secondary NameNode?
Ans:
The Secondary NameNode in HDFS aids in periodic consolidation of file system metadata and edit logs from the primary NameNode. This process helps maintain file system consistency and recovery capabilities, enhancing system reliability. However, it does not serve as a standby or backup NameNode for failover.
57. What are the advantages of a smaller block size in HDFS?
Ans:
- Reduced Storage Overhead
- Improved Data Locality
- Efficient Storage for Small Files
- Optimized for Random Access
- Reduced Data Transfer Overhead
- Enhanced Fault Tolerance for Small Files
58. Explain the process of data read and write in HDFS.
Ans:
Data Write in HDFS: When data is written to HDFS, it is split into fixed-size data blocks. The client communicates with the NameNode for block placement, then writes data blocks to DataNodes. Replication ensures data durability.
Data Read in HDFS: For data retrieval, the client queries the NameNode for block locations, accesses DataNodes in parallel for block retrieval, and reconstructs the file. Replication provides fault tolerance and availability.
59. How is data stored and replicated in HDFS?
Ans:
In HDFS, data is stored in fixed-size data blocks, typically 128 MB or 256 MB each. These blocks are replicated across multiple DataNodes, typically three times, to ensure fault tolerance and data availability. The replication process involves placing copies of data blocks on separate DataNodes, distributed across the cluster, reducing the chance of data loss as a result of node failures or hardware issues.
60. What is block locality in HDFS, and why is it important?
Ans:
- Block locality in HDFS means processing data on nodes where data blocks are stored, reducing network data transfer.
- It’s vital in HDFS for efficient data access, reducing network congestion, and enhancing performance, especially in large-scale data processing.
61. What command is used to list files and directories in HDFS?
Ans:
The command used to list files and directories in HDFS is:
- hadoop fs -ls
You can use this command to view the contents of the Hadoop Distributed File System and retrieve a list of files and directories along with their details.
62. What is the purpose of the ‘-put’ and ‘-copyFromLocal’ commands in HDFS?
Ans:
The ‘-put’ and ‘-copyFromLocal’ commands in HDFS are used for the same purpose: Copying files or directories from the local file system of the client machine into HDFS. They enable data ingestion into HDFS, making it available for processing by Hadoop applications. These commands simplify the process of transferring data, allowing users to specify the source and destination paths, and facilitating efficient data import into the Hadoop ecosystem.
63. How does HDFS ensure data replication?Ans:
HDFS ensures data replication by creating multiple copies (usually three) of each data block. When a file is written to HDFS, the NameNode identifies suitable DataNodes and instructs them to store replicas of the data block. These replicas are distributed across different DataNodes, racks, and clusters, providing fault tolerance and data availability.
64. How can you delete a file or directory in HDFS?Ans:
To delete a file or directory in HDFS, you can use the following command:
For a file:
- hadoop fs -rm
For a directory and its contents:
- hadoop fs -rm -r
Replace ‘
Ans:
The ‘-get’ and ‘-copyToLocal’ commands in HDFS copy files or directories from HDFS to the local file system. They simplify the extraction of data, making it accessible for local processing and analysis, enabling users to work with HDFS data on their client machines.
66. What is the impact of changing the replication factor in HDFS?Ans:
- Data Availability and Redundancy
- Improved Fault Tolerance
- Increased Storage Overhead
- Network Traffic
- Reduced Data Loss Risk
Changing the replication factor in HDFS has several significant impacts:
67. Can you set different replication factors for different files in HDFS?
Ans:
No, in HDFS, you typically set the same replication factor for all files within the same cluster. HDFS uses a uniform replication factor to maintain data consistency and simplicity in cluster management. However, different clusters within the same Hadoop ecosystem can have distinct replication factors based on their specific requirements and use cases.
68. What is a data block in HDFS?
Ans:
A data block in HDFS is a fixed-size, contiguous unit of data storage. It is the smallest logical division of data within HDFS and typically has a default size of 128 MB or 256 MB, although this size can be configured.
69. How are data blocks managed in HDFS?Ans:
Data blocks in HDFS are managed by dividing large files into fixed-size blocks (e.g., 128 MB). These blocks are replicated across multiple nodes in the cluster to ensure fault tolerance and data availability. HDFS automatically distributes and places replicas on suitable nodes, monitors cluster health, and recovers data in case of failures, providing a robust and scalable storage solution for big data.
70. What happens if a data block is corrupt or lost in HDFS?Ans:
If a data block is corrupt or lost in HDFS:
Replicas: HDFS stores multiple copies of each block, so it can still access data from healthy replicas.
Automatic Recovery: HDFS detects and replaces corrupt or missing replicas automatically.
Checksums: Checksums help verify data integrity, and HDFS reads from healthy replicas if there’s a checksum mismatch.
Data Node Decommission: If a DataNode is problematic, it can be removed from the cluster to prevent further issues.
Ans:
- Prepare new hardware.
- Install Hadoop software on the new machines.
- Configure HDFS settings and data directories.
- Update cluster configuration and replication settings.
- Start DataNode services on the new machines.
- Monitor and ensure the new DataNodes are healthy.
- Optionally, balance data distribution.
- Test cluster functionality.
- Update documentation and monitoring systems.
Ans:
Decommissioning a DataNode in HDFS is a process where the administrator marks the node for removal, triggering data replication to maintain redundancy. Once all data is replicated, the DataNode is safely removed from the cluster, ensuring data availability and fault tolerance.
73. How do you upgrade the HDFS version in a Hadoop cluster?Ans:
Backup Data and Configurations: Create backups of data and configuration files to prevent data loss during the upgrade.
Check Compatibility: Verify compatibility between the current and target HDFS versions.
Test Upgrade: Set up a test environment to validate the upgrade process.
Upgrade HDFS: Install the new Hadoop version on all nodes in the cluster.
Testing and Rollout: Thoroughly test the cluster, address issues, and plan a production upgrade during a maintenance window.
74. What is rack awareness in HDFS?Ans:
Rack awareness is a feature in Hadoop Distributed File System (HDFS) that helps optimize data placement and improve fault tolerance within a Hadoop cluster. It is a strategy for organizing DataNodes (storage nodes) within the cluster into racks, where a rack typically represents a physical network switch or a group of closely connected nodes.
75. What is the purpose of the HDFS balancer tool, and when should it be used?Ans:
The HDFS balancer tool in Hadoop is used to balance the distribution of data blocks across DataNodes within an HDFS cluster. Its primary purpose is to ensure that data is evenly spread across all DataNodes in the cluster, thereby optimizing storage capacity and performance.
The HDFS balancer should be used when data block distribution becomes uneven due to node additions or other factors, as it helps optimize storage and prevent performance degradation in Hadoop clusters.
76. How does HDFS use rack awareness to optimize data placement?Ans:
- Organizing nodes into racks based on network topology.
- Preferring local rack storage for data replicas.
- Ensuring cross-rack replicas for fault tolerance.
- Smartly balancing data to reduce network traffic.
HDFS optimizes data placement with rack awareness by:
Ans:
Rack awareness is essential for HDFS as it optimizes data placement by considering the physical network topology. It reduces network overhead by placing data replicas within the same rack, improving data locality and minimizing data transfer times. Rack awareness also enhances fault tolerance by ensuring replicas are distributed across different racks, reducing the risk of data loss due to rack failures.
78. What security mechanisms are available in HDFS?Ans:
- Authentication and Authorization
- Encryption
- Auditing and Logging
- Pluggable Authentication and Authorization
- Delegation Tokens
Ans:
To secure data in transit in HDFS, enable SSL/TLS encryption. Configure HDFS to use SSL/TLS for data transfer by modifying Hadoop configuration files like ‘hdfs-site.xml’. Ensure that SSL/TLS certificates are generated and deployed across the cluster. Thoroughly test and monitor the SSL/TLS setup to guarantee secure communication between HDFS clients and servers, safeguarding data during transmission.
80. What is the purpose of Kerberos authentication in HDFS?Ans:
Purpose of Kerberos Authentication in HDFS:
User Verification: Verify user and service identities within the Hadoop cluster.
Authorization: Ensure authorized access to HDFS resources.
Single Sign-On (SSO): Enable seamless access to multiple Hadoop services.
Credential Security: Protect against credential theft with encrypted tickets.
Centralized Management: Simplify user authentication in large clusters.
81. How does HDFS Federation differ from a traditional HDFS cluster?Ans:
HDFS Federation allows a single Hadoop cluster to support multiple namespaces, each with its own directory structure and block pools. It provides enhanced scalability, isolation, and high availability, making it suitable for large organizations with diverse data needs.
In contrast, a traditional HDFS cluster operates with a single shared namespace and block pool. It is simpler and well-suited for smaller to mid-sized clusters with less complex data requirements.
82. What is the role of the Standby NameNode in HDFS High Availability?Ans:
Failover: Acts as a backup for the Active NameNode.
Metadata Sync: Synchronizes metadata with the Active NameNode.
Checkpointing: Periodically creates metadata snapshots for faster recovery.
State Transition: Takes over as Active NameNode during failure.
Cluster Monitoring: Monitors DataNodes for cluster health during failover.
83. What is checkpointing in HDFS, and why is it necessary?Ans:
Checkpointing in HDFS involves periodically creating a snapshot of the file system metadata, including the namespace and file-to-block mappings. It’s necessary to reduce the time required for the NameNode to recover in case of a failure.
84. How does the checkpointing process work in HDFS?Ans:
- Trigger: Secondary NameNode initiates checkpoint periodically.
- Merge: Merges current fsimage with edits log.
- Upload: Uploads the new fsimage to the NameNode.
- Restart: NameNode restarts using the updated fsimage for quicker recovery.
- Edits Log: Truncates or clears the edits log up to the checkpointed point.
The checkpointing process in HDFS works as follows:
85. How can you backup data in HDFS?
Ans:
To back up data in HDFS, several approaches are available. HDFS Snapshots can be used to create read-only copies of directories or files at specific points in time, enabling data recovery. Additionally, the Hadoop DistCP (Distributed Copy) tool allows for the efficient copying of data between HDFS clusters, facilitating cross-cluster backups. Maintaining data replication across multiple HDFS clusters provides built-in redundancy and backup, enhancing both data availability and protection.
86. What strategies can be employed for data recovery in HDFS?Ans:
- Replication
- Checkpointing
- DataNode Block Recovery
- Secondary NameNode
- High Availability (HA)
- Snapshots
- DistCP (Distributed Copy)
Ans:
To optimize HDFS performance for data processing workloads, consider adjusting block sizes to align with processing requirements, optimizing hardware resources by adding more DataNodes and ensuring sufficient storage, and implementing data compression techniques to reduce storage and transfer overhead. Additionally, fine-tune configuration parameters like the replication factor to balance redundancy and performance for efficient data processing.
88. What factors can impact the performance of HDFS?Ans:
Block Size: Inadequate or excessive block sizes can affect read and write performance.
Hardware Resources: The availability of sufficient memory, CPU, and disk resources on NameNodes and DataNodes is crucial.
Network Bandwidth: Network speed and bandwidth affect data transfer rates within the cluster.
Data Replication: The replication factor chosen affects data redundancy and network resources.
Cluster Size: The number of nodes in the HDFS cluster influences overall performance.
89. What are some popular tools and frameworks that work with HDFS?Ans:
- Apache MapReduce
- Apache Spark
- Apache Hive
- Apache Pig
- Apache HBase
- Apache Sqoop
Ans:
Hadoop Distributed File System (HDFS) works seamlessly with Apache MapReduce. HDFS stores distributed data, and MapReduce processes it efficiently by leveraging data locality, dividing input data into splits aligned with HDFS blocks, and storing output back in HDFS for persistence and analysis. This integration forms the foundation of Hadoop’s data processing capabilities.
91. Can you explain the role of HDFS in Big Data analytics and processing pipelines?Ans:
Hadoop Distributed File System serves as the fundamental storage infrastructure for Big Data analytics and processing pipelines. It offers scalable and distributed storage, ensuring fault tolerance and data durability.
HDFS enables efficient data processing by optimizing data locality and acts as the backbone for processing frameworks like Apache Spark and MapReduce, allowing organizations to analyze and extract insights from vast datasets in the Big Data landscape.
92. What are some alternatives to HDFS in the Hadoop ecosystem?Ans:
- Apache HBase: NoSQL database for real-time access.
- Apache Cassandra: Scalable NoSQL database.
- Amazon S3: Cloud-based object storage.
- Alluxio (formerly Tachyon): In-memory data storage layer.
- Apache Kudu: Columnar storage engine for fast analytics.
- GlusterFS: Distributed file system.
Alternatives to Hadoop Distributed File System (HDFS) in the Hadoop ecosystem:
Ans:
Decommissioning a DataNode in Hadoop Distributed File System (HDFS) does not significantly impact data availability. HDFS maintains data availability by replicating data across multiple DataNodes. When decommissioning, HDFS ensures that data blocks on the decommissioned node are still available on other active nodes, preserving data redundancy and uninterrupted access.
94. What is the purpose of adding more DataNodes to a Hadoop cluster?Ans:
Adding more DataNodes to a Hadoop cluster aims to increase storage capacity, enhance data distribution for improved performance, and strengthen fault tolerance by replicating data across nodes. This scalability strategy accommodates larger datasets and processing demands in the cluster.
95. How can you monitor the progress of a DataNode decommissioning operation?Ans:
- Use NameNode web UI.
- Run ‘hdfs dfsadmin -report’ command.
- Review DataNode logs.
- Consider third-party monitoring tools for more comprehensive insights.
To monitor DataNode decommissioning in HDFS:
Ans:
When new DataNodes are added to Hadoop Distributed File System (HDFS), data rebalancing occurs to distribute existing data evenly across all DataNodes. HDFS employs a block replication and deletion process, creating new replicas on the added DataNodes and gradually balancing the cluster’s data distribution. This ensures optimal data placement and enhances cluster performance.
97. How can you scale an HDFS cluster to accommodate growing data volumes?Ans:
Add DataNodes: Expand storage and data distribution.
Adjust Block Size: Optimize for larger files.
Upgrade Hardware: Improve cluster performance.
Review Replication: Balance redundancy and capacity.
Monitor and Tune: Optimize configuration.
Consider Data Tiering: Manage data based on access patterns.
98. What is the purpose of checksums in HDFS?Ans:
The purpose of checksums in Hadoop Distributed File System (HDFS) is to ensure data integrity. Checksums are used to detect and prevent data corruption or errors that may occur during storage and data transfer. By calculating and comparing checksums, HDFS can identify and recover from data inconsistencies, ensuring the reliability and accuracy of stored data.
99. How can you manually trigger data block verification in HDFS?Ans:
To manually trigger data block verification in HDFS, use the ‘hdfs fsck’ command with the ‘-verify’ option, specifying the path to the file or directory you want to verify. This command checks the integrity of data blocks and reports verification results, helping ensure data reliability in the Hadoop cluster.
100. Can you explain the term “edit log” in the context of the NameNode?Ans:
In the context of the NameNode in HDFS, the “edit log” is a crucial component that records all metadata modifications, such as file creations and deletions. It ensures data consistency and recoverability by capturing changes before they are applied to the file system image, facilitating quick recovery in case of NameNode failures. The edit log plays a pivotal role in maintaining the integrity of HDFS’s metadata.