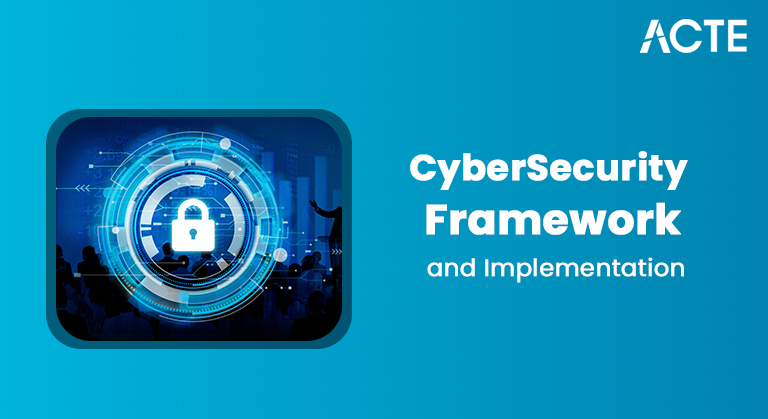
- Introduction to Data Integration
- Common Data Integration Challenges
- Key Components of a Successful Data Integration Strategy
- Data Quality: The Foundation of Effective Integration
- Tools and Technologies for Data Integration
- Best Practices for Overcoming Data Integration Challenges
- Case Studies: Real-World Examples of Data Integration Success
- Conclusion: Future of Data Integration and Its Impact
Excited to Achieve Your Business Analyst Certification? View The Business Analyst Online course Offered By ACTE Right Now!
Introduction to Data Integration
Data integration is the process of combining data from multiple sources into a unified, accessible view. This is crucial in today’s data-driven world, where businesses must consolidate disparate data from various departments, systems, and third-party services for insightful decision-making. However, data integration can often be fraught with challenges, making it a complex task for organizations to navigate successfully. In the digital age, organizations face ever-growing volumes of data from various sources such as cloud storage, legacy systems, IoT devices, and social media platforms. Effective integration becomes paramount with data in different formats, structures, and storage locations. Business Analyst Training can help you develop the skills necessary to address these integration challenges. Data integration helps companies achieve a consistent view of their operations, customers, and performance metrics. This enhances the ability to analyze data and make informed decisions. However, poor data integration practices can result in data silos, inconsistent information, and inefficient workflows, which can impede business success. This blog post will explore the common challenges faced during data integration, strategies to overcome them, and best practices to ensure successful data integration projects.
Common Data Integration Challenges
Despite the clear benefits of data integration, there are numerous challenges that organizations face:
1. Data SilosMany organizations still struggle with data silos, where different departments or teams store and manage their data independently. This makes integrating information across the business challenging, leading to inconsistent and incomplete views of the organization’s data.
2. Data Quality IssuesData coming from multiple sources often has quality problems. These include missing values, duplicate records, incorrect formats, and inconsistent data standards. Poor data quality leads to inaccurate insights, hampering decision-making.
3. Data CompatibilityData from various systems might use different formats, standards, or structures. Integrating these incompatible data sets can result in information loss or errors during processing. If you’re interested in exploring potential career opportunities in this field, the Business Analyst Career Path can guide you in understanding the skills and progression required for success.
4. Scalability and VolumeWith the growing volume of data, businesses face challenges in scaling their data integration processes. Traditional data integration tools may not be equipped to handle big data or rapidly growing datasets, which can lead to performance issues.
5. Real-Time IntegrationMany businesses now require real-time data for decision-making. Integrating data in real-time across multiple systems is a significant challenge, especially when dealing with legacy systems or slow data pipelines.
6. Security and Privacy ConcernsIntegrating sensitive or personal data introduces security and privacy risks. Ensuring compliance with regulations like GDPR and HIPAA while integrating data is a significant challenge, especially when data is transferred across different platforms.
7. Complexity of Managing Multiple SystemsOrganizations often use on-premise systems, cloud-based solutions, and third-party applications. Managing and integrating these disparate systems seamlessly can be complex and resource-intensive.
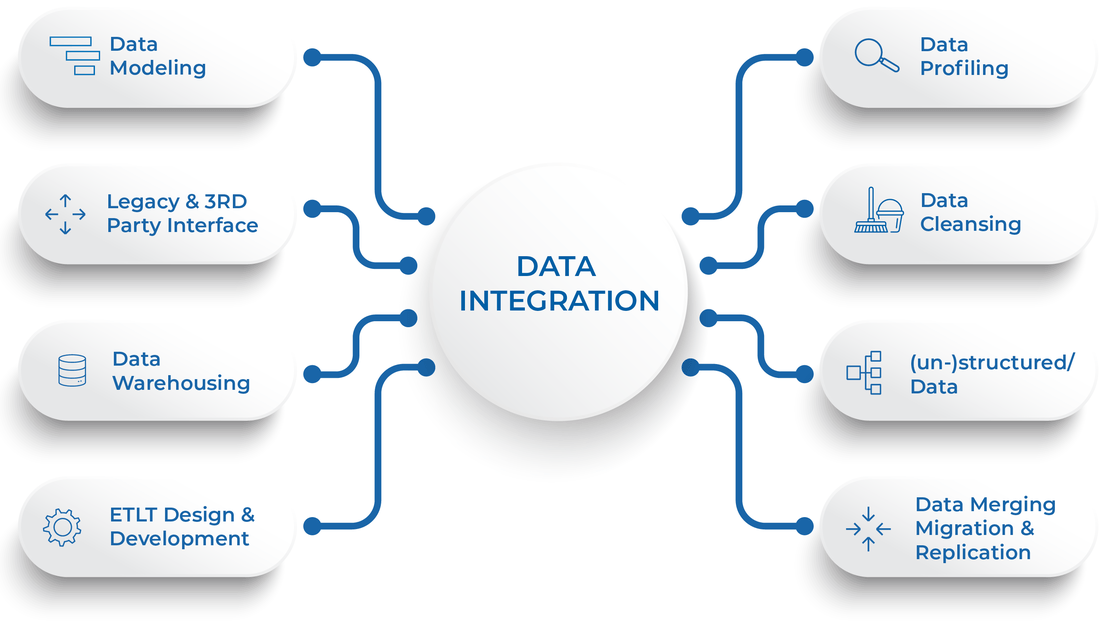
Key Components of a Successful Data Integration Strategy
To overcome data integration challenges, businesses should adopt a strategic approach that includes the following key elements:
1.Data Governance- Ensures data is accurate, consistent, and compliant with regulations.
- Defines rules for data quality, security, access control, and privacy.
- Simplifies data management across systems through a structured framework. 2.Data Mapping and Transformation
- Maps data from different sources to a common format.
- Involves converting data types, removing inconsistencies, and aligning with the target system’s schema. Understanding this process is crucial in the evolving Business Intelligence Landscape where accurate and consistent data is key to effective analysis and decision-making.
- Utilizes transformation tools to automate the process. 3.Centralized Data Repository
- Stores data in a centralized warehouse or cloud-based system.
- Enhances efficiency in data access, storage, and analysis.
- Reduces complexity by eliminating reliance on multiple systems. 4.Automation and Orchestration
- Automates integration tasks to minimize human error and speed up data flows.
- Orchestrates the entire data pipeline to ensure seamless data movement between systems.
Data Quality: The Foundation of Effective Integration
Data quality is a critical factor that directly impacts the success of data integration. Without high-quality data, even the best integration tools and technologies will fall short. To ensure effective integration, businesses must focus on the following aspects of data quality:
1. Data AccuracyData should reflect the actual state of the real-world entities they represent. Inaccurate data can lead to poor decision-making and incorrect analysis.
2. Data CompletenessMissing or incomplete data can hinder effective integration. Before integrating the data into the system, it’s essential to identify and resolve any gaps in the data.
3. Data ConsistencyData should be consistent across systems. Inconsistencies in data, such as using different units of measurement or formats for dates, must be addressed before integration to avoid errors. Leveraging tools like SAS Analytics is Essential for Data-Driven Decision can help ensure data consistency and support accurate, informed business decisions.
4. Data TimelinessOutdated or stale data reduces the relevance of insights. Ensuring data is current and regularly updated is critical for businesses that need real-time analytics.
Excited to Obtaining Your Business Analyst Certificate? View The Business Analyst Training Offered By ACTE Right Now!
Tools and Technologies for Data Integration
Several tools and technologies are available to help businesses overcome data integration challenges. These include:
1. ETL (Extract, Transform, Load) ToolsETL tools are essential for data extraction, transformation, and loading into centralized systems like data warehouses. Popular ETL tools include Apache Nifi, Talend, and Informatica. They automate much of the process, ensuring data is integrated quickly and accurately. When combined with Big Data Visualization Tools these ETL solutions help organizations not only process data efficiently but also present it in meaningful, actionable ways.
2. API IntegrationAPIs (Application Programming Interfaces) facilitate data exchange between different systems. They allow systems to communicate in real-time, making API-based integration a suitable solution for businesses requiring up-to-date data.
3. Data VirtualizationData virtualization provides a way to access and integrate data without physically moving it. It abstracts the data and allows it to be accessed as if it were in a single location, making it a good option for integrating data from multiple sources.
4. Data Integration PlatformsPlatforms like MuleSoft, Microsoft Power Automate, and Dell Boomi allow easy integration across cloud-based and on-premise systems. These platforms offer pre-built connectors and templates for seamless integration.
Interested in Pursuing Business Intelligence Master’s Program? Enroll For Business Intelligence Master Course Today!
Best Practices for Overcoming Data Integration Challenges
To successfully overcome data integration challenges, businesses should adopt a set of best practices that enhance efficiency, accuracy, and compliance. The first step is to standardize data formats and structures across all data sources to ensure compatibility and minimize integration errors. Investing in regular data cleansing is equally important, as it helps eliminate duplicates, correct errors, and often maintain overall data quality through automated cleansing tools. Organizations should also implement scalable data integration solutions that can grow alongside increasing data volumes, ensuring long-term effectiveness and adaptability. Continuous monitoring and auditing of data integration processes are vital for identifying and resolving issues early, enabling ongoing improvements. If you’re looking to gain more insights into these practices, Business Analyst Training can provide the skills needed for successful implementation. Lastly, ensuring security and compliance is essential. Businesses must adhere to regulations like GDPR or CCPA and safeguard sensitive data through encryption, secure APIs, and robust access controls.
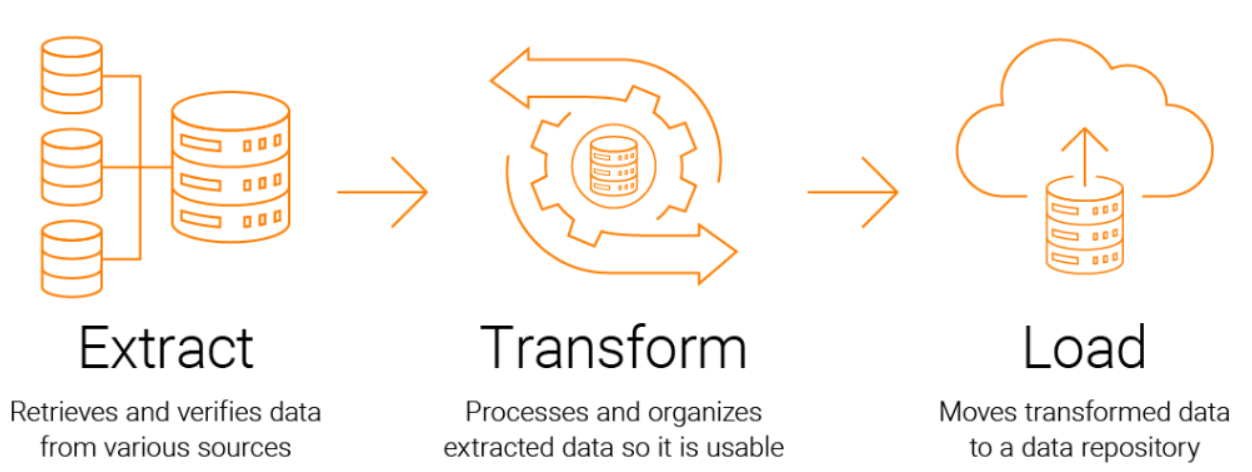
Case Studies: Real-World Examples of Data Integration Success
Case Study 1: Retail Business Optimizing Customer DataA large retail chain faced challenges integrating customer data from various touchpoints, including in-store, online, and mobile apps. They implemented an integrated customer data platform (CDP) that consolidated all customer interactions into a single view. This allowed them to deliver personalized marketing campaigns, resulting in a 25% increase in customer engagement.
Case Study 2: Healthcare Provider Improving Patient OutcomesA healthcare provider struggled with integrating data from different systems, such as electronic health records (EHR) and laboratory information management systems (LIMS). By using an integrated data management platform, the provider successfully linked patient records, lab results, and treatment plans, improving patient outcomes and operational efficiency.
Getting Ready for a Business Analyst Job Interview? Check Out Our Blog on Business Analyst Interview Questions & Answer
Conclusion: Future of Data Integration and Its Impact
The future of data integration looks promising as businesses continue to adopt advanced technologies like artificial intelligence (AI), machine learning (ML), and blockchain. These innovations make data integration faster, more accurate, and more secure. As businesses embrace digital transformation, overcoming data integration challenges will remain a priority. With the right tools, strategies, and practices in place, organizations can unlock the full potential of their data, leading to improved decision-making, better customer experiences, and enhanced business outcomes. If you’re looking to expand your expertise in this area, Business Analyst Training can provide you with the necessary skills. Data integration is not just necessary for modern businesses; it’s a strategic advantage that will continue to shape the future of business operations across industries.