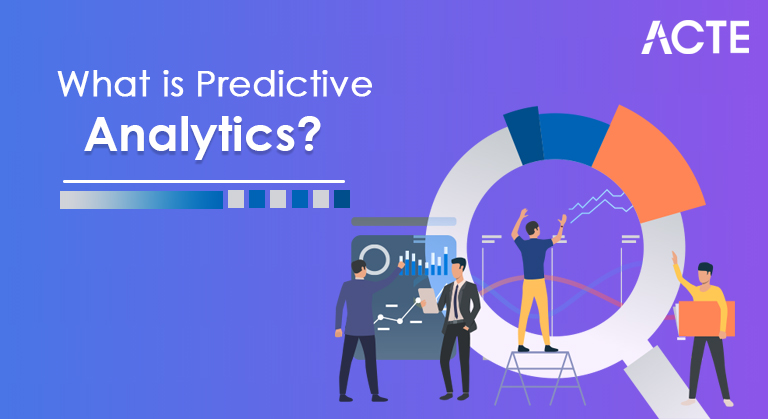
Predictive analytics is the use of data, statistical algorithms and machine learning techniques to identify the likelihood of future outcomes based on historical data. The goal is to go beyond knowing what has happened to providing a best assessment of what will happen in the future.
- Predictive Analysis
- Definition- Predictive Analysis
- Features of Predictive Analysis”
- Types of Predictive Analysis
- Applications
- Technology and big data influence
- Analytical techniques
- Tools used by Predictive Analysis
- Benefits of Predictive Analysis
- Conclusion
- Predictive analytics includes a variety of statistical techniques ranging from data mining, predictive modeling, and machine learning that analyze current and historical facts to make predictions about future or otherwise unknown events.
- In business, predictive models take advantage of patterns found in historical and transactional data to identify risks and opportunities. Models capture the relationship between a number of factors in order to assess the risk or potential associated with a particular position, guiding decision-making for candidate transactions.
- The defining functional impact of these technical approaches is that predictive analysis provides a predictive score (probability) for each individual (customer, employee, health care patient, product SKU, vehicle, component, machine, or another organizational unit). so as to be determined, informed. , or affect organizational processes that relate to large numbers of individuals, such as government operations including marketing, credit risk assessment, fraud detection, manufacturing, health care, and law enforcement.
- Predictive analytics is used in actuarial science, [4] marketing, [5] business management, sports/fantasy, [6] insurance, [7] policing, [8] telecommunications, [9] retail, [10] travel, goes. 11] Mobility, [12] Health Care, [13] Child Protection, [14] Pharmaceuticals, [15] Capacity Planning, [16] Social Networking [17] and other fields.
- One of the most well-known applications is credit scoring,[1] which is used throughout business management. Scoring models process a customer’s credit history, loan applications, customer data, etc., to rank-order individuals based on their likelihood of making timely credit payments in the future.
Predictive Analysis:
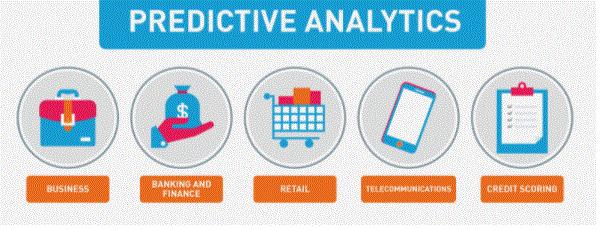
Definition- Predictive Analysis:
Predictive analytics is a field of statistics that uses it to extract information from data and predict trends and behavior patterns. The growth of predictive web analytics online calculates the statistical probabilities of future events. Predictive Analytics Statistical techniques include data modeling, machine learning, AI, deep learning algorithms, and data mining. Often the unknown event of interest is in the future, but predictive analysis can be applied to any type of unknown whether it is in the past, present or future. For example, identifying suspects after a crime has occurred, or when credit card fraud has occurred. [19] The core of predictive analysis is to capture the relationship between explanatory variables and variables predicted from past events and unknowns. Depends on exploiting them to predict the outcome. However, it is important to note that the accuracy and usefulness of the results will depend greatly on the level of data analysis and the quality of the assumptions.
Predictive analytics is often defined as making predictions at a more detailed level of granularity, that is, generating predictive scores (probabilities) for each individual organizational element. This separates it from forecasting. For example, “predictive analysis—technology that learns from experience (data) to predict the future behavior of individuals in order to make better decisions.” [20] In industrial systems of the future, the value of predictive analysis will be to predict and prevent potential issues and to be further integrated into prescriptive analysis for decision optimization to achieve near-zero break-down.
- The first priority of any predictive analytics project is a clear understanding of the business objective being supported. Predictive analytics is applied to customer/potential identification, attrition/retention estimates, fraud detection, and credit/default estimates.
- The common feature of these opportunities is the individual tendency of individuals to exhibit a behavior that affects a business objective.
- Traditional statistical analysis is best suited for occasions where an increased understanding of the population is desired. The best predictive analysis opportunities involve the identification of extremes of behavioral trends.
- A key factor for the successful development of predictive analytics projects is a well-defined set of business performance metrics specific to the organization’s business objectives.
- Models developed in predictive analytics should be evaluated in the context of these performance metrics. Relying on traditional statistical performance metrics, such as R2 or Lift, results in models that appear viable but ultimately fail to perform in business decision environments.
- This behavior of interest is what we are coding into our historical data as a “desired output” variable. Indicates whether the behavior was exhibited for each record in our data set.
- It is important that we take the time to ensure that this behavior is defined in a way that supports our business objectives and is consistent with our assessed performance metrics. Failure to correctly define and code the behavior of our interest virtually guarantees significantly reduced performance or complete project failure.
- As an example, most fraud detection projects are actually meant to identify instances where additional investigation is needed. Many organizations are willing to allocate their investigative resources to more important cases to avoid additional investigations to relatively minor errors.
- Too often, we get caught up in the technical aspects of an opportunity and lose sight of the business motivation. In general, the single most effective characteristic of concrete business opportunities for predictive analysis is the identification of a decision process that involves the allocation of scarce resources.
- Whenever we are faced with the allocation of time or money in our business relationships, there are some relationships that will benefit us and others that are either less productive or that negatively impact our performance metrics. These are ideal opportunities for the application of predictive analysis.
- By effectively handling the complexity of the large number of features associated with each of these relationships, we often obtain a scoring system that serves as a relative ranking of different groups based on their propensity to be of interest. Demonstrated behavior allows us to adjust our resource allocation strategies and enhance business performance.
Features of Predictive Analysis”:
Feature #1: A Clear Business Purpose
Feature #2: Defined Performance Metrics
Feature #3: Distinctive Behavior of Interest
Feature #4: Identified Resource Allocation Decisions
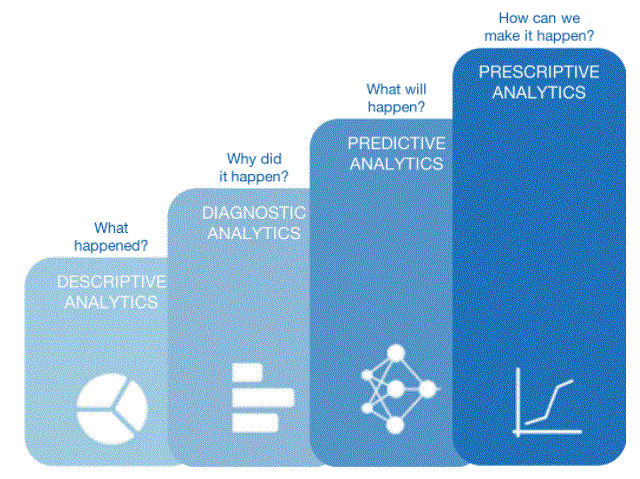
Types of Predictive Analysis:
Generally, the term predictive analytics is used for predictive modeling, “scoring” data with predictive models, and forecasting. However, people are increasingly using the term to refer to related analytical disciplines, such as descriptive modeling and decision modeling or optimization. These disciplines also include rigorous data analysis, and are widely used for segmentation and decision-making in business, but have different purposes and differ statistical techniques. Predictive modeling uses predictive models to analyze the relationship between the specific performance of an entity in a sample and one or more known features or characteristics of that entity.
The purpose of the model is to assess the probability that a similar entity in a different sample will exhibit typical performance. This category includes models in many areas, such as marketing, where they look for subtle data patterns to answer questions about customer performance, or fraud detection models. Predictive models are often calculated during live transactions, for example, to evaluate the risk or opportunity of a given customer or transaction to guide a decision. With advances in computing speed, individual agent modeling systems have become capable of simulating human behavior or responses to given stimuli or scenarios.
Descriptive model
Descriptive models measure relationships in data in a way that is often used to classify customers or prospects into groups. Unlike predictive models, which focus on predicting single customer behavior (such as credit risk), descriptive models identify many different relationships between customers or products. Descriptive models do not rank-order customers based on their likelihood of taking a particular action the way predictive models do. Instead, descriptive models can be used, for example, to classify customers based on their product preferences and standard of living. Descriptive modeling tools can be used to further develop models that can simulate a large number of individual agents and make predictions.
Decision model
Decision models describe the relationships between all the elements of a decision – known data (including the outcome of the predictive model), the decision, and the predictive outcome of the decision – to predict the outcomes of decisions with multiple variables. These models can be used in optimization, maximizing some outcomes while minimizing others. Decision models are typically used to develop decision logic or a set of business rules that will produce the desired action for each customer or situation.
Applications:
Although predictive analysis can be used in many applications, we outline a few examples where predictive analysis has shown a positive impact in recent years.
Business
Analytical Customer Relationship Management (CRM) is a frequent business application of predictive analytics. Predictive analytics methods are applied to customer data to build a holistic view of the customer. CRM uses predictive analytics in applications for marketing campaigns, sales and customer services. Analytical CRM can be applied across the entire lifecycle of customers (acquisition, relationship growth, retention, and win-returns).
Child protection
Some child welfare agencies have started using predictive analysis to characterize high-risk cases. For example, in Hillsborough County, Florida, the use of predictive modeling tools by the Child Welfare Agency has prevented abuse-related child deaths in the target population.
Edit Clinical Decision Support System
Predictive analysis is used primarily in health care to determine which patients are at risk of developing conditions such as diabetes, asthma or heart disease. Additionally, sophisticated clinical decision support systems incorporate predictive analysis to aid in medical decision making. A 2016 study of neurodegenerative disorders provides a powerful example of a CDS platform to diagnose, track, predict and monitor the progression of Parkinson’s disease.
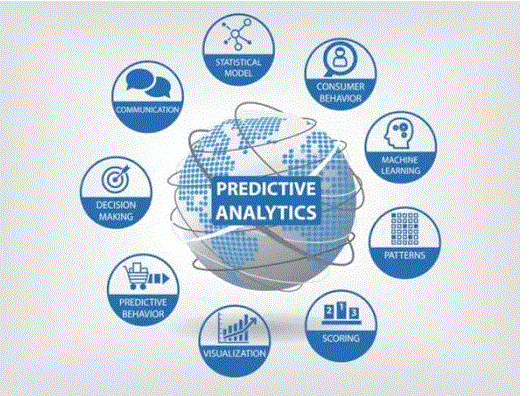
Technology and big data influence:
Big data is a collection of data sets that are so large and complex that they become awkward for traditional database management tools to use. The volume, diversity and velocity of big data present challenges across the board for capture, storage, search, sharing, analysis and visualization. Examples of big data sources include web logs, RFID, sensor data, social networks, Internet search indexing, call detail records, military surveillance, and complex data in astronomical, biogeochemical, genomics and atmospheric sciences.
Big data is the core of most predictive analytical services offered by IT organizations. [29] Thanks to technological advances in computer hardware – faster CPUs, cheaper memory, and MPP architectures – and new technologies such as Hadoop, MapReduce, and in-database and text analytics for processing big data, the aggregating now, It is possible to analyze and mine. Huge amounts of structured and unstructured data for new insights. [30] It is also possible to run predictive algorithms on streaming data. [31] Today, exploring big data and using predictive analytics is within the reach of more organizations than ever before and new methods capable of handling such datasets are proposed.
Analytical techniques:
The approaches and techniques used to perform predictive analysis can be broadly divided into regression techniques and machine learning techniques.
Regression techniques
Regression models are the mainstay of predictive analysis. The focus is on setting up a mathematical equation as a model to represent the interactions between the various variables under consideration. Depending on the situation, there are different types of models that can be applied when performing predictive analysis. Some of them are briefly discussed below.
Linear regression model
The linear regression model predicts the response variable as a linear function of the parameters with unknown coefficients. These parameters are adjusted so that a measure of fit is optimized. Most of the effort in model fitting is focused on reducing the size of the residual as well as ensuring that it is randomly distributed with respect to the model predictions. The goal of regression is to select the parameters of the model in order to minimize the sum of square residuals. This is known as the Ordinary Least Squares (OLS) estimation.
Discrete choice model
Multiple regression (above) is typically used when the response variable is continuous and has an infinite range. Often the response variable may be discrete but not continuous. While it is mathematically possible to apply multiple regression to discrete ordered dependent variables, some of the assumptions behind the theory of multiple linear regression no longer hold, and there are other techniques such as discrete choice models that are better suited for this type of analysis. are favorable. If the dependent variable is discrete, some of the better methods are logistic regression, multinomial logit, and probit models. Logistic regression and probit models are used when the dependent variable is binary.
Logistic regression
In a classification setting, outcome probabilities can be specified for observations through the use of a logistic model (also called a logic model), which transforms information about the binary dependent variable into an unbounded continuous variable. and estimates a regular multivariate model. Wald and likelihood-ratio tests are used to test the statistical significance of each coefficient b in the model (corresponding to the t tests used in OLS regression; see above). One test estimating the goodness-of-fit of a classification model is the “percentage of correctly predicted”.
Probit regression
Probit models provide an alternative to logistic regression for modeling categorical dependent variables.
Multinational Logistics Regression
An extension of the binary logit model in cases where the dependent variable has more than 2 categories is the multinomial logit model. In such cases it may not make sense to divide the data into two categories or there may be loss in the richness of the data. The multinomial logit model is the appropriate technique in these cases, especially when the dependent variable categories are not ordered (eg colors such as red, blue, green). Some authors have extended multinomial regression to include feature selection/significance methods such as random multinomial logit.
- Historically, using predictive analytics tools—as well as understanding the results they produce—required advanced skills. However, modern predictive analytics tools are no longer limited to IT specialists. [citation needed] As more organizations adopt predictive analytics in decision-making processes and integrate it into their operations, they are creating a shift in the market toward business users as the primary. consumers of information. Business users want tools they can use on their own.
- There are many tools available in the Marketplace that help in the execution of predictive analytics. These range from those that require little user sophistication to those designed for the expert practitioner. The difference between these tools often lies in the level of optimization and the heavy data lifting allowed.
Tools used by Predictive Analysis:
PMML
Predictive Model Markup Language (PMML) was proposed as the standard language for expressing predictive models. Such an XML-based language provides a way for different devices to define and share predictive models. PMML 4.0 was released in June, 2009.- There are many benefits to using predictive analysis. As mentioned above, using this type of analysis can help entities when you need to make predictions about outcomes when no other (and obvious) answers are available.
- Investors, financial professionals and business leaders have been able to use the model to help reduce risk. For example, an investor and their advisor may use certain models to help the investor design an investment portfolio with minimal risk, such as taking into account age, capital, and goals.
- Cost reduction has a significant impact when the model is used. Businesses can determine the probability of success or failure of a product before it is launched. Or they may set aside capital to improve production using predictive techniques before the manufacturing process begins.
Benefits of Predictive Analysis:
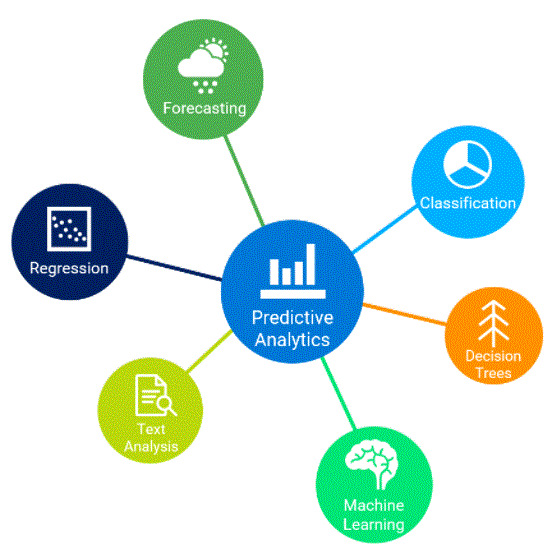
Conclusion:
Predictive analysis has been plagued by exaggerated expectations, hype and excessive emphasis on sophisticated quantitative techniques. Appropriate identification of opportunities where predictive analysis can be successfully applied is often the determining factor in the success of projects.
Predictive analytics is the branch of advanced analytics used to make predictions about unknown future events. Predictive analytics uses a range of techniques from data mining, statistics, modeling, machine learning and artificial intelligence to analyze current data to make predictions about the future.