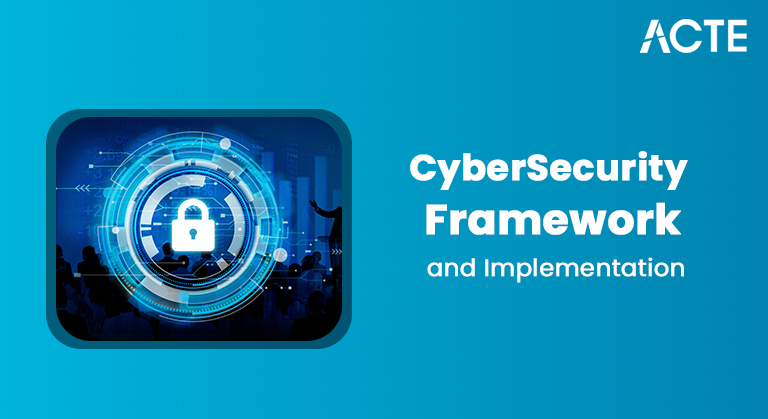
- Introduction to Data Science
- Role of Data Science in Modern Businesses
- Data-Driven Decision Making
- How Data Science Enhances Efficiency
- Impact of Data Science on Industries
- Data Science and Artificial Intelligence
- Big Data and Its Role in Data Science
- Machine Learning in Data Science
- Challenges in Data Science Adoption
- Future Scope of Data Science
- Case Studies on Data Science Implementation
- Conclusion and Key Takeaways
Introduction to Data Science
Data Science is an interdisciplinary field that utilizes scientific methods, processes, algorithms, and systems to derive knowledge and insights from both structured and unstructured data. It integrates expertise from diverse areas, including mathematics, statistics, computer science, and domain-specific knowledge, to analyze and interpret complex data sets. With the rapid expansion of data in the digital age, Data Science Training has become an essential component of decision-making across organizations and industries globally.
Data Science involves collecting, processing, analyzing, and interpreting large datasets. It is essential in uncovering patterns, making predictions, and driving decisions that can significantly impact businesses and organizations. Professionals in this field use various tools, techniques, and technologies, such as machine learning, statistical analysis, and data mining, to derive valuable insights from data.
Role of Data Science in Modern Businesses
Data has become one of the most valuable assets in today’s business environment. The role of Data Science in modern businesses is pivotal in driving growth, enhancing efficiency, and creating new opportunities. Companies can make informed decisions, improve processes, and stay competitive in their respective markets by extracting actionable insights from vast amounts of data.
Key Roles in Business:- Customer Insights: Data science helps businesses understand customer preferences, behaviors, and needs through data analysis, allowing companies to personalize their offerings.
- Operational Efficiency: Businesses can use data science to optimize operations, reduce costs, and improve workflow efficiencies.
- Market Analysis: By analyzing market trends, customer sentiments, and competitor data, businesses can identify growth opportunities and mitigate risks.
- Product Development: Data science provides insights into consumer needs and helps businesses develop products that meet market demands.
- Risk Management: It helps forecast potential risks and provides businesses with tools to manage uncertainties.
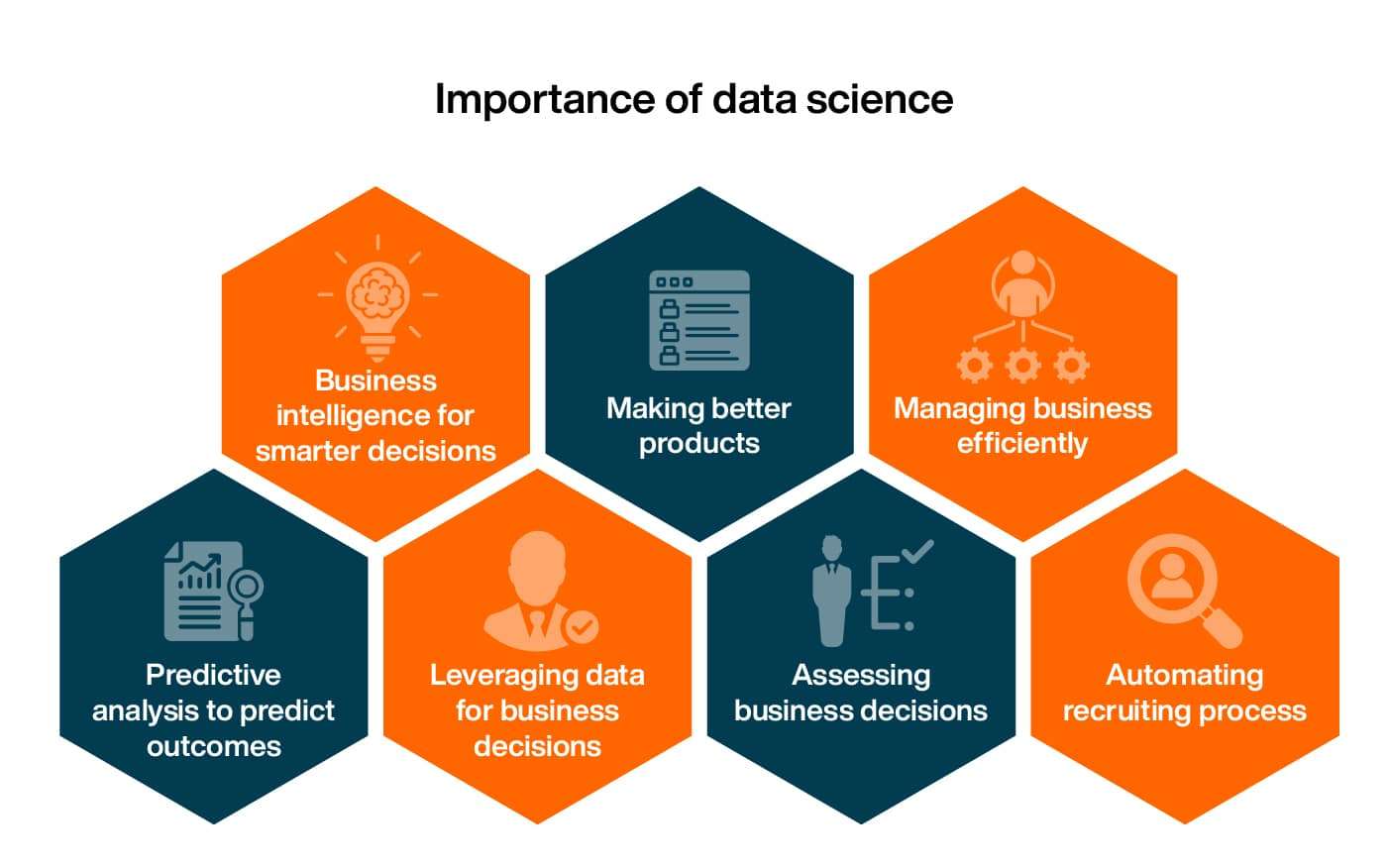
Data-Driven Decision Making
Data-driven decision-making (DM) refers to using data analysis to guide business decisions instead of relying on intuition or gut feelings. Data Science Training helps organizations make more informed and accurate decisions by providing insights grounded in empirical data rather than assumptions.
Benefits of Data-Driven Decisions:- Accuracy: Decisions based on data are often more accurate and reliable because they are grounded in evidence.
- Predictive Insights: Data Science uses historical data to predict future trends, helping businesses to make proactive decisions.
- Objective Analysis: Data analysis removes biases and emotions from decision-making, ensuring objectivity.
- Optimized Outcomes: Using data to drive decisions leads to optimized business strategies, maximizing ROI, and reducing waste.
By incorporating data-driven decision-making processes, businesses can improve their operational processes, enhance customer satisfaction, and increase profitability.
How Data Science Enhances Efficiency
Data Science enhances efficiency in various business operations, from automating routine tasks to optimizing processes and resource allocation. Applying data science techniques can help businesses streamline operations, reduce operational costs, and improve productivity.
Key Contributions to Efficiency:- Process Automation: Machine learning and predictive analytics can automate repetitive tasks, such as data entry, customer service responses, and inventory management.
- Resource Optimization: Data science models can identify the most efficient use of resources like time, labor, and money, helping businesses optimize production schedules and resource allocation.
- Supply Chain Optimization: Predictive models in data science can forecast demand, enabling businesses to manage their supply chains better and reduce stockouts and overstocking.
- Time-Saving: By analyzing large datasets efficiently, data science reduces the time needed to gather insights and speeds up the decision-making process.
Data Science tools and techniques ensure that businesses can quickly respond to market shifts and customer preferences, ensuring operational agility.
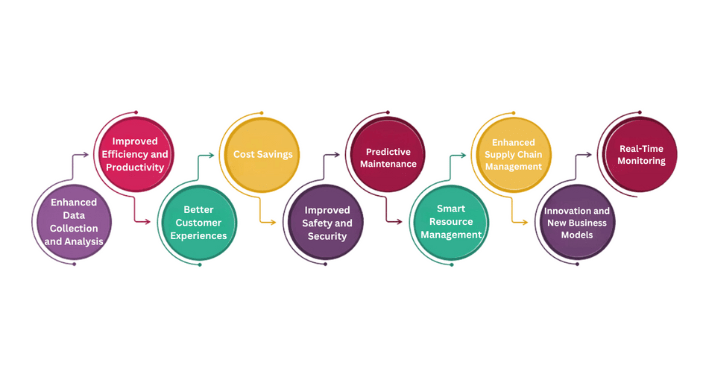
As businesses expand, the ability to manage larger and more complex data becomes critical. Lastly, data accuracy is essential, as a good extraction tool should minimize errors and ensure that the data captured is reliable and correct. These features together help ensure that the tool will be both effective and efficient in managing data extraction tasks.
Impact of Data Science on Industries
Data Science has disrupted and revolutionized various industries, enabling companies to deliver innovative products and services while improving internal processes. Data science has a profound impact across multiple sectors by enabling better decision-making and efficient operations.
Key Industry Impacts:- Healthcare: Data Science is used in predictive modeling for disease diagnosis, patient care, and treatment optimization. It helps identify trends, improve patient outcomes, and streamline healthcare services.
- Retail: Retailers use data science for inventory management, personalized marketing, demand forecasting, and pricing optimization. They also utilize data to enhance customer experiences through targeted advertising and recommendation systems.
- Finance: In finance, data science is widely used in fraud detection, algorithmic trading, credit scoring, and risk management. Financial institutions leverage big data and predictive analytics to make data-driven investment decisions. Those interested in mastering these techniques can benefit from Data Science Training, which equips professionals with the necessary skills and knowledge.
- Manufacturing: Data Science helps optimize supply chains, monitor production processes, and detect equipment malfunctions. Predictive maintenance and quality control systems are powered by data science to reduce downtime and improve product quality.
- Transportation: Data Science is used to optimize routes, predict maintenance issues, and improve the overall efficiency of transportation systems. For example, companies like Uber use data science to optimize ride-sharing logistics.
- Telecommunications: Telecommunication companies use data science to predict customer churn, optimize networks, and improve customer service through chatbots and other automated systems.
- Entertainment: Streaming services like Netflix use data science for personalized content recommendations, improving user experience and retention rates.
Data Science and Artificial Intelligence
Data Science and Artificial Intelligence (AI) are closely linked fields that often complement one another. AI uses advanced algorithms to simulate human-like decision-making, and Data Science provides the data and tools necessary for those algorithms to learn and improve.
How They Work Together:- Machine Learning is one of the key areas of AI. It is powered by large datasets and is a critical component of data science. Machine learning models are trained using historical data and can make predictions or automate tasks based on new data.
- Deep Learning is a subset of machine learning that uses neural networks and large datasets to solve complex problems like image recognition and natural language processing.
- Predictive Analytics: Data Science helps train AI models to predict future outcomes based on past data, making AI more effective in automation and decision-making processes.
AI and Data Science can solve complex problems, create new products and services, and significantly enhance business operations.
Big Data and Its Role in Data Science
Big Data refers to massive datasets that cannot be processed or analyzed using traditional data management tools. With the advent of the internet, social media, and IoT devices, the amount of data generated has increased exponentially. Data Science harnesses the power of Big Data to develop insights and make data-driven decisions at an unprecedented scale.
Key Features of Big Data:- Volume: Refers to the vast amount of data generated from various sources.
- Velocity: Refers to the speed at which data is generated and needs to be processed.
- Variety: Refers to the different types of data, including structured, semi-structured, and unstructured data.
- Veracity: Refers to the uncertainty and reliability of the data.
Big Data tools such as Hadoop and Spark empower data scientists to process, analyze, and visualize vast datasets, offering businesses invaluable insights that were previously unattainable. Learn more about the Necessary Skills required to master these tools.
Machine Learning in Data Science
Machine Learning (ML) is a critical subset of AI in data science. ML algorithms allow systems to automatically learn from data and improve over time without being explicitly programmed.
Key Types of Machine Learning:- Supervised Learning: The model is trained using labeled data to predict new, unseen data outcomes.
- Unsupervised Learning: This model identifies patterns in data without predefined labels. It is often used in clustering and association problems.
- Reinforcement Learning: The model learns by interacting with an environment and receiving feedback (rewards or penalties) to improve its decision-making over time.
Machine learning models are widely used in data science to improve predictions, enhance customer experience, and automate decision-making processes.
Challenges in Data Science Adoption
Despite its many benefits, the adoption of Data Science in businesses faces several challenges, including
- Data Quality and Integrity: Poor data quality can lead to inaccurate insights and decisions. Businesses must invest in data cleaning and preparation to ensure high-quality data.
- Skill Gap: The demand for skilled data scientists is high, but there is a shortage of professionals with the right expertise in statistics, machine learning, and data engineering.
- Data Privacy and Security: With increasing concerns over data breaches and privacy regulations like GDPR, businesses must handle sensitive data responsibly and securely.
- Integration with Existing Systems: Integrating data science solutions into legacy systems can be complex and time-consuming.
- Cost of Implementation: Implementing data science infrastructure and technologies can be costly, especially for small businesses.
Overcoming these challenges requires the right strategy, tools, and a skilled workforce capable of effectively using data science.
Future Scope of Data Science
The future of Data Science is auspicious, with continuous advancements in technology and data collection methods. Some areas where Data Science is expected to grow include:
- AI-Powered Analytics: AI and machine learning will continue to advance, enabling even more sophisticated analytics and decision-making processes.
- Real-Time Analytics: The ability to analyze data in real-time will become more prevalent, allowing businesses to respond more quickly to changing conditions.
- Edge Computing: With the rise of IoT, data analysis will increasingly happen on devices rather than relying solely on centralized servers, enabling faster and more efficient processing.
- Ethical AI: As AI continues to evolve, there will be a growing emphasis on creating ethical and transparent AI systems that can be trusted by businesses and consumers alike.
The future scope of data science looks expansive, especially with emerging technologies like quantum computing, blockchain, and augmented reality, all of which will rely heavily on data analytics.
Case Studies on Data Science Implementation
1. Netflix- Netflix uses data science extensively for personalized recommendations, content optimization, and customer retention. Netflix provides tailored content suggestions by analyzing user behavior, viewing history, and ratings, contributing significantly to its success. 2. Amazon
- Amazon uses Machine Learning Algorithms to optimize its supply chain, recommend products to customers, and forecast demand. Its recommendation engine is among the most significant contributors to sales and user engagement. 3. Uber
- Uber uses data science to optimize ride-sharing algorithms, predict ride demand, set dynamic pricing, and map the most efficient routes. This data-driven approach has allowed Uber to expand rapidly in global markets.
Conclusion and Key Takeaways
Data Science has transformed businesses’ operations by providing insights that drive more intelligent decision-making, increase efficiency, and uncover new opportunities. With the increasing availability of big data and advancements in machine learning and Artificial Intelligence, the role of data science in businesses is becoming more crucial than ever.
As businesses face challenges in adopting data science, overcoming these hurdles will require investment in the right tools, skilled professionals, and a robust data strategy. The future of data science looks bright, with an expanding scope in various industries, and its potential to shape the future of business is immense.