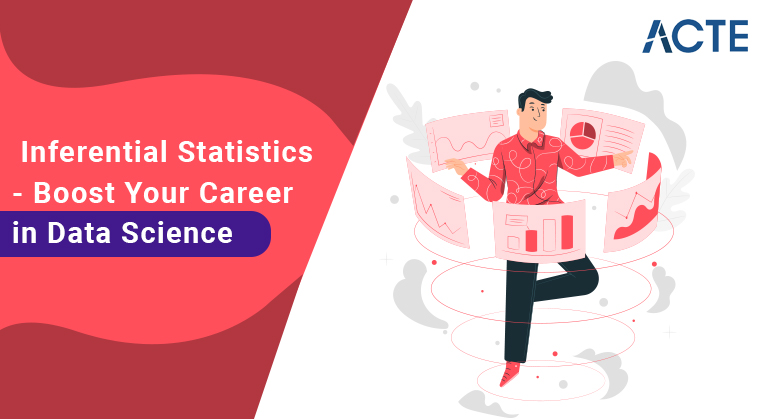
What You Need to Know About Inferential Statistics to Boost Your Career in Data Science | Expert’s Top Picks
Last updated on 25th Dec 2021, Blog, General
In simple language, Inferential Statistics is used to draw inferences beyond the immediate data available. With the help of inferential statistics, we can answer the following questions: Making inferences about the population from the sample. Concluding whether a sample is significantly different from the population.
- What Are Inferential Statistics?
- Types of Inferential Statistics
- How Analysts Use Inferential Statistics in Decision-Making
- Examples of Inferential Statistics
- What’s the Difference Between Inferential Statistics and Descriptive Statistics?
- Statistics are a Critical Backbone in a Data Science Career
- Why do we need Inferential Statistics
- Statistical terminologies
- Hypothesis testing
- Conclusion
- Knowing how to work with statistics is essential if you are pursuing a career in data science. But have you ever wondered for a moment what statistics really are? To the average person, statistics are just a series of numbers and other random information that smart people use to prove their point. However, statistics is both a subtle and complex concept that needs a closer look.
- Experts define statistics as a branch of science or mathematics that involves the collection, classification, analysis, interpretation and presentation of numerical facts and data. Statistics are especially useful when analysts must work with vast populations that are too broad for specific, detailed measurements. Statistics are necessary to draw general conclusions about the dataset taken from a data sample.
- There are two distinct branches of statistics: descriptive and deductive. Today, we look at the statistics of conjecture. This article covers the definitions, types of inference statistics, difference between descriptive statistics and inference statistics, and more.
- Do you know the difference between making a guess and a guess? Applying involves giving information, whereas estimating involves obtaining information. When a speaker implies something, they are suggesting something without saying it explicitly. When a listener infers something, they draw or come to a conclusion based on logic and evidence rather than explicit information.
- This goes a lot towards defining inferential statistics. This branch of statistics samples random data from a part of the population to make predictions, draw conclusions based on that information, and normalise the results to represent the data at hand.
- The best way to obtain accurate analysis when using inferred data is to identify the measurement or study of the population, to sample for that part of the population, and to use analysis to factor in any sampling errors.If a data analyst takes data results and makes no inferences, inferences, or generalisations, they will practice descriptive statistics. More on that later.
What Are Inferential Statistics?
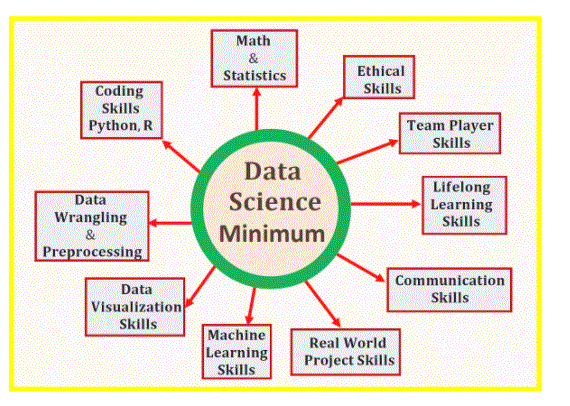
Types of Inferential Statistics:
Estimated statistics employ four different methods or types:
Parameter estimation. Analysts take a statistic from sample data and use it to make informed estimates about the mean parameter of the population. It uses estimators such as probability plotting, Bayesian estimation methods, rank regression, and maximum likelihood estimation.
Confidence interval. Analysts use confidence intervals to obtain interval estimates for chosen parameters. They are used in research to find the margin of error to determine whether it will affect the test.
Regression analysis Regression analysis is a series of statistical procedures that estimate the relationship between a dependent variable and a set of independent variables. This analysis uses hypothesis tests to determine whether the relationships observed in sample data actually exist in the population.
Hypothesis testing. Analysts attempt to answer research questions by using sample data and making assumptions that incorporate population parameters. This test determines whether the measured population has a higher value than any other data point in the analysis. In this exercise, you are trying to find the error margin by multiplying the standard error of the mean by the z-score.
- Estimated statistics have two primary purposes:
- Make estimates related to population groups
- Test hypothesis to draw conclusions related to population
- For example, a data analyst might randomly sample a group of 11th graders in a given field and collect SAT scores and other personal information. Using estimated data and data samples, the researcher can estimate and test the hypothesis about 11th grade across the country.
- Or a political advisor may collect voter information from a specific area and establish how many people voted for each presidential candidate. Armed with that information, the consultant can project how voters will vote for a particular referendum question.
- Analysts can also use predictive statistics to predict which movies or television shows have a higher chance of success. Data from test screenings and focus groups helps analysts predict how viewers will react to a new program and its potential nationwide audience. We will revisit this idea later.
How Analysts Use Inferential Statistics in Decision-Making:
- Sample mean.
- Sample standard deviation.
- Creating a Boxplot or Bar Chart.
- Description of the shape of the probability distribution of the sample.
Examples of Inferential Statistics:
Estimated statistics use statistical models to help data analysts compare their sample data with other samples or already related research. Most analysts use statistical models called generalised linear models, which include methods such as ANOVA (analysis of variance), t-tests, regression analysis, and others that generate linear or straight-line probabilities and outcomes.
Let’s say, for example, that you have sample data about an upcoming new television show, drawn from a sample of the population that has watched an “as-yet-unpublished” TV pilot episode. You can use that data to create a set of descriptive statistics that describe your sample, including:
- Now that we know what inferential statistics are, how is it different from descriptive statistics? We have already pointed out that descriptive statistics present data clearly and directly without any speculation on other analytical possibilities, so this is a start.
- Inferential statistics take random samples of data from a segment of a population and make inferences about the population as a whole. So, if you asked 100 people whether they preferred Cola A or Cola B, and 60 of them chose Cola A, the estimated figures build on that and assume that those survey results are soda in general. Will be valid for the drinking population.
- On the other hand, descriptive statistics never take things that far. This tells you that, in a survey conducted at one location, 60 percent of those surveyed liked Cola A better, and that is it.
- If it appears that descriptive statistics is a more complex concept than descriptive statistics, that is because it is. Descriptive statistics tell you how things are based on your data. Predictive statistics use that data to make a logical leap in predicting future outcomes. Naturally, heuristic statistics require more tools to accomplish this ambitious goal, and some of the tools are very complex and involve difficult number-crunching, graphing, and charting.
- In short, descriptive statistics give you a single, clear snapshot of your current data findings. Estimated statistics takes that same data and makes an estimate based on the results of the data.
- Incidentally, we should note that the two statistics share a similar characteristic – they both depend on the same dataset.
What’s the Difference Between Inferential Statistics and Descriptive Statistics?
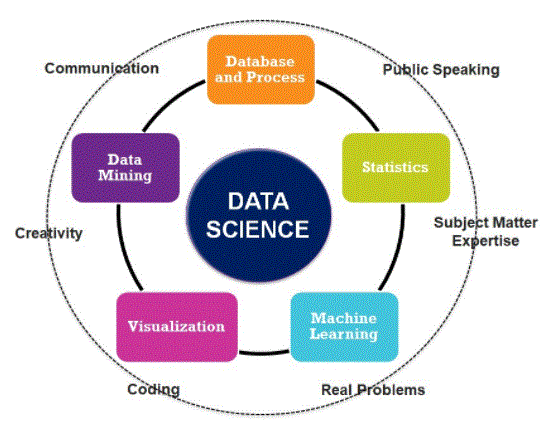
- Whether you are interested in descriptive or inferential statistics, the fields of data science and data analysis offer many opportunities for motivated professionals. It’s good to learn both.
- To further your statistics and mathematical training and boost your career, Simpilarn’s Post Graduate Program in Data Science opens the door to important data science concepts and tools such as Python, R, machine learning, and more. The acclaimed program offers practical laboratories and project work to bring ideas to life with the help of skilled instructors and teaching assistants who guide and mentor you.
- This rigorous and comprehensive bootcamp, organised in partnership with Purdue University and in collaboration with IBM, offers the ideal mix of theory, case studies and extensive practical practice.
- According to Glassdoor, data scientists earn an average of US$113,309 annually. Payscale shows that a data scientist in India earns an average of ₹817,366 annually. Data Science is an ideal and timely career option if you want a challenge in a demanding business that also provides you with financial security.
Statistics are a Critical Backbone in a Data Science Career:
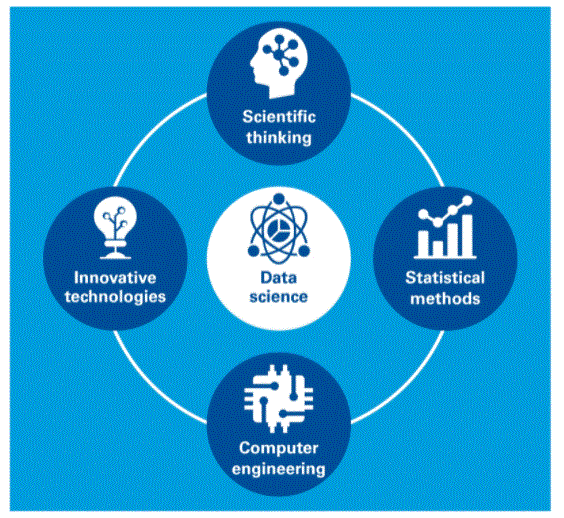
- First, take a few samples and try to find one that most accurately represents the entire population.
- Next, test the sample and use it to make generalisations about the entire population.
Why do we need Inferential Statistics:
Unlike descriptive statistics, instead of having access to the entire population, we often have a limited amount of data. In such cases, approximate statistics come into action. For example, we might be interested in finding the average of test scores for an entire school. This is not advisable as it may seem impractical to obtain the data we need.
So, instead of getting test scores for the entire school, we measure a small sample of students (for example, a sample of 50 students). This sample of 50 students would now describe the entire population of all students in that school. Simply put, inferential statistics make predictions about a population based on a sample of data taken from that population.
The technique of inferential statistics includes the following steps:
There are two main purposes of inferential statistics:
Estimated parameter: We take a statistic, such as the standard deviation, from the collected data, and use it to define a more general parameter, such as the standard deviation of the entire population.
Hypothesis testing: Very beneficial when we want to gather data on something that can only be administered to a very limited population, such as a new drug. If we want to know whether this drug will work for all patients (the “full population”), we can use the collected data to estimate it (often by calculating the z-score).
Statistical terminologies:
Throughout the article, I will use the following statistical terminology frequently:
Data: A single measure of some characteristics of a sample. For example, the mean/mean/mode of a sample of data scientists in Bangalore.
Population data: The total population data in context. For example, the population means the salary of the entire population of data scientists across India.
Sample Data: The data of a group taken from the population. For example, the average salary of all data scientists in New York.
Standard Deviation: This is the amount of variation in the population data, given by.
Standard Error: This is the amount of variation in the sample data. It is related to the standard deviation as /√n, where n is the sample size.
Possibility: Probability of an event refers to the probability of that event happening.
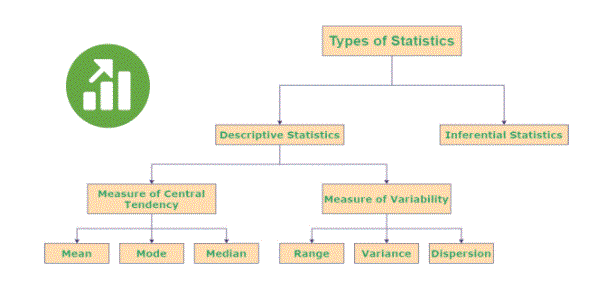
- The population from which the sample comes follows a normal distribution of scores.
- The sample size is large enough to represent the population
- Variation, a measure of prevalence, is the same between each group
- When your data violates any of these assumptions, non-parametric tests are more appropriate.
- Non-parametric tests are called “distribution-free tests” because they assume nothing about the distribution of the population data.
Hypothesis testing:
Hypothesis testing is a formal process of statistical analysis using inferential statistics. The goal of hypothesis testing is to compare populations or assess relationships between variables using samples. Hypotheses, or predictions, are tested using statistical tests. Statistical tests also estimate sampling errors so that valid conclusions can be drawn. Statistical tests can be parametric or non-parametric. Parametric tests are considered statistically more powerful because they are more likely to detect an effect if one is present.
Parametric tests make assumptions that include the following:
Conclusion:
When it comes to inferential statistics, there are two main limitations.
The first limitation comes from the fact that since the data being analysed is from a population that has not been fully measured, data analysts can never be 100% sure that the data being calculated is accurate. Since inferential analysis is based on the process of using the values measured in a sample to eliminate the values measured from the total population, there will always be some level of uncertainty regarding the results.
The second limitation is that some heuristic tests require the analyst or researcher to make an educated guess based on the principles for running the test. As with the first limitation, there will be uncertainty about these estimates, which will also mean some impact on the reliability of the results of some statistical tests.
Inferential statistical analysis is the method that will be used to draw conclusions. It allows users to predict trends or draw conclusions about a larger population based on the samples analysed. Basically, it takes data from a sample and then draws conclusions about a larger population or group.