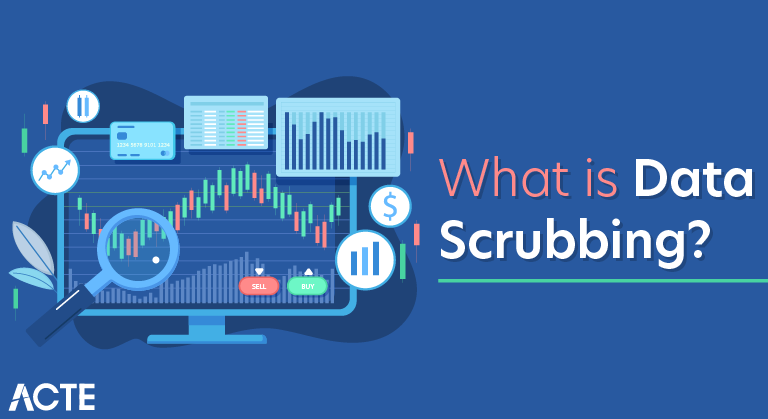
What is Data Scrubbing? All you need to know [OverView]
Last updated on 16th Jul 2020, Blog, General
- Data scrubbing refers to the procedure of modifying or removing incomplete, incorrect, inaccurately formatted, or repeated data in a database. The key objective of data scrubbing is to make the data more accurate and consistent.
- Data scrubbing is a vital strategy for ensuring that databases remain accurate. It is especially important in data-intensive industries, including telecommunications, insurance, banking and retailing. Data scrubbing systematically evaluates data for flaws or mistakes with the help of look-up tables, rules and algorithms.
- Data scrubbing is also referred to as data cleansing.
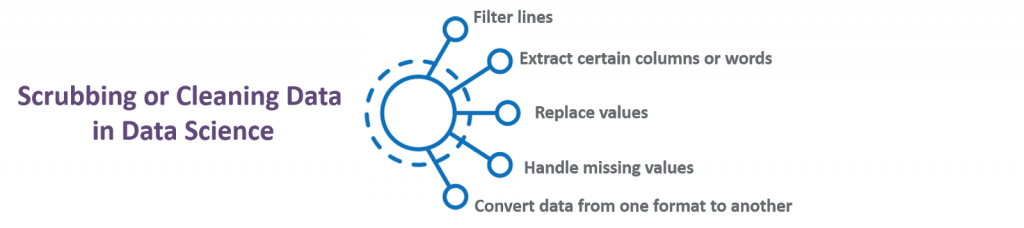
5 Simple Data Scrubbing Steps for Your Team
If there was a simple way to eliminate dirty data in your company, would you use it? Here is a practical suggestion with five simple steps to consider:
- Determine at least one area in your business with a data quality problem.
- Enlist the participation of top management by demonstrating business benefits due to data quality improvements.
- Review current data quality in the “target” area.
- Use data scrubbing software and other data scrubbing tools to produce a sample report about the current data quality in the target area.
- Within your various teams, find “Data Quality Champions” to lead the way by spreading awareness and communicating both big and small achievements in improving data quality.
Remember – you are not alone in this effort. As noted above, well over 80 percent of companies have data problems lurking somewhere within the organization.
Here’s how a typical data scrubbing process goes:
Define and Plan: Identify the data that is important in the day to day process of your operation. Identify fields that are critical to your goals, and define validation rules accordingly to standardize your data. This may include job title, email address, or zip code.
Assess: Understand what needs to be cleaned up, what information is missing, and what can be deleted. Exceptions to rules also need to be set up – that way, the data cleansing process will be easier..
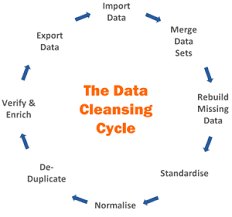
Execute: It’s time to run the cleansing process. Create workflows to standardize and cleanse the flow of data to make it easier to automate the process. Investigate, standardize, match, and survive data sets as necessary.
Review: Audit and correct data that cannot be automatically corrected, such as phone numbers or emails. Define certain fix procedures for future use.
Manage and Monitor: Evaluating the database is important after the cleansing process is complete. Track the results of any campaigns that ran with the cleansed data, such as bounced emails or returned postcards through reporting functions.
How do you clean data?
While the techniques used for data cleaning may vary according to the types of data your company stores, you can follow these basic steps to map out a framework for your organization.
Step 1: Remove duplicate or irrelevant observations
Remove unwanted observations from your dataset, including duplicate observations or irrelevant observations. Duplicate observations will happen most often during data collection. When you combine data sets from multiple places, scrape data, or receive data from clients or multiple departments, there are opportunities to create duplicate data. De-duplication is one of the largest areas to be considered in this process.
Irrelevant observations are when you notice observations that do not fit into the specific problem you are trying to analyze. For example, if you want to analyze data regarding millennial customers, but your dataset includes older generations, you might remove those irrelevant observations. This can make analysis more efficient and minimize distraction from your primary target—as well as creating a more manageable and more performant dataset.
Step 2: Fix structural errors
Structural errors are when you measure or transfer data and notice strange naming conventions, typos, or incorrect capitalization. These inconsistencies can cause mislabeled categories or classes. For example, you may find “N/A” and “Not Applicable” both appear, but they should be analyzed as the same category.
Step 3: Filter unwanted outliers
Often, there will be one-off observations where, at a glance, they do not appear to fit within the data you are analyzing. If you have a legitimate reason to remove an outlier, like improper data-entry, doing so will help the performance of the data you are working with. However, sometimes it is the appearance of an outlier that will prove a theory you are working on.
Remember: just because an outlier exists, doesn’t mean it is incorrect. This step is needed to determine the validity of that number. If an outlier proves to be irrelevant for analysis or is a mistake, consider removing it.
Step 4: Handle missing data
You can’t ignore missing data because many algorithms will not accept missing values. There are a couple of ways to deal with missing data. Neither is optimal, but both can be considered.
- As a first option, you can drop observations that have missing values, but doing this will drop or lose information, so be mindful of this before you remove it.
- As a second option, you can input missing values based on other observations; again, there is an opportunity to lose integrity of the data because you may be operating from assumptions and not actual observations.
- As a third option, you might alter the way the data is used to effectively navigate null values.
Step 4: Validate and QA
At the end of the data cleaning process, you should be able to answer these questions as a part of basic validation:
- Does the data make sense?
- Does the data follow the appropriate rules for its field?
- Does it prove or disprove your working theory, or bring any insight to light?
- Can you find trends in the data to help you form your next theory?
- If not, is that because of a data quality issue?
False conclusions because of incorrect or “dirty” data can inform poor business strategy and decision-making. False conclusions can lead to an embarrassing moment in a reporting meeting when you realize your data doesn’t stand up to scrutiny.
Before you get there, it is important to create a culture of quality data in your organization. To do this, you should document the tools you might use to create this culture and what data quality means to you.
Benefits of data cleaning
Having clean data will ultimately increase overall productivity and allow for the highest quality information in your decision-making. Benefits include:
- Removal of errors when multiple sources of data are at play.
- Fewer errors make for happier clients and less-frustrated employees.
- Ability to map the different functions and what your data is intended to do.
- Monitoring errors and better reporting to see where errors are coming from, making it easier to fix incorrect or corrupt data for future applications.
- Using tools for data cleaning will make for more efficient business practices and quicker decision-making.
Filtering the Data
Data cleansing is the procedure that filters out irrelevant data. Irrelevant data usually includes duplicate records, missing or incorrect information and poorly formatted data sets. A business can expand this option furthermore by eliminating the data records that are not really necessary for certain business processes. While what gets filtered out depends on the discretion of the business, some basic points like outdated data or details that are not verified can be removed.
Though the data cleansing process takes a good chunk of your time as well as resources to complete, it undermines some potential of receiving major insights from the big data.
Need for Clean Data
Inaccurate data analytics result into misguided decision making which can expose the industry to compliance issues since many decisions are subject to requirements in order to make sure that their data is accurate and current.
Though the reduction of the potential for bad data quality can be taken care of by the process management and process architecture, it cannot be completely eliminated. The only B2B solution left is to detect and remove or correct the errors and inconsistencies in a database or data-set and make the bad data usable.