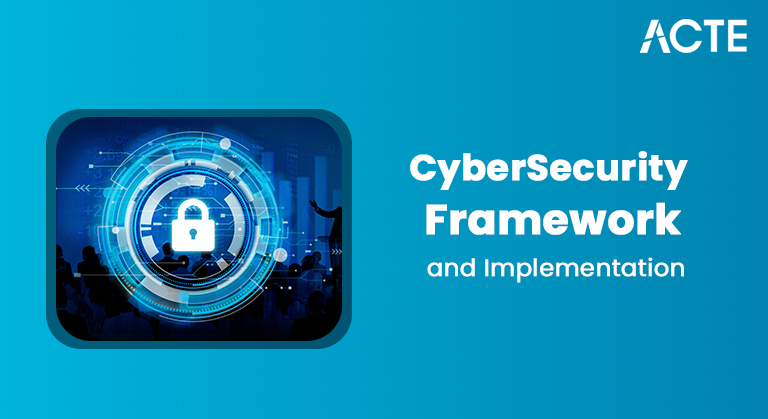
- Introduction to Healthcare Data Analytics
- Importance of Data in Healthcare
- Types of Healthcare Data: Patient, Clinical, and Operational
- Data Collection and Cleaning in Healthcare
- Predictive Analytics in Healthcare
- Applications of Machine Learning in Healthcare
- Data Privacy and Security Regulations
- Tools and Technologies in Healthcare Analytics
- Real-World Use Cases Disease Prediction, Diagnosis
- Challenges in Healthcare Data Analytics
- Benefits of Data-Driven Decision-Making
- Future of Data Analytics in Healthcare
Introduction to Healthcare Data Analytics
Healthcare Data Analytics is the process of analyzing and transforming vast amounts of healthcare data into actionable insights, empowering healthcare organizations to enhance decision-making, streamline operations, improve patient outcomes, and reduce costs. This data is collected from diverse sources such as electronic health records (EHR), patient surveys, medical imaging, laboratory results, and more. The primary objective of healthcare data analytics is to leverage this wealth of information to drive improvements in patient care, boost operational efficiency, and optimize the overall healthcare system. As the healthcare sector continues to evolve into a more data-centric industry, the importance of data analytics has grown significantly. The widespread adoption of technologies such as EHR, wearables, and Internet of Things (IoT) devices has led to an exponential increase in the volume of healthcare data being generated. To meet this growing demand, professionals are increasingly turning to Data Science Training which equips them with the skills to analyze complex datasets and extract meaningful insights. By effectively utilizing these insights, healthcare organizations can improve the quality of patient care, streamline hospital operations, and achieve substantial cost savings. The integration of data analytics into healthcare operations is becoming essential to not only address the growing demands of the healthcare industry but also to ensure that the potential of emerging technologies is fully harnessed to deliver better healthcare outcomes and create a more efficient, patient-focused system.
Would You Like to Know More About Data Science? Sign Up For Our Data Science Course Training Now!
Importance of Data in Healthcare
The importance of data in healthcare cannot be overstated. Data is at the core of nearly every aspect of healthcare, from patient care to administrative operations. Here’s why it’s so crucial:
- Improved Patient Outcomes: By analyzing patient data, healthcare providers can better understand a patient’s condition, track progress, and personalize treatment plans, leading to better health outcomes.
- Cost Efficiency: Data analytics helps organizations identify inefficiencies and areas where cost-cutting measures can be applied without compromising the quality of care. This is especially important in the face of rising healthcare costs.
- Operational Efficiency: Data analytics can help streamline hospital operations, such as improving patient flow, reducing wait times, and optimizing resource allocation.
- Predictive Capabilities: Data allows healthcare providers to predict disease outbreaks, identify at-risk patients, and even forecast potential complications, which can be proactively managed.
- Research and Development: Data is key to scientific research in healthcare, allowing researchers to analyze trends, study outcomes, and test new hypotheses in the pursuit of innovation.
- Policy Making: Health data enables governments and organizations to make informed decisions on public health policies, resource distribution, and healthcare reforms.
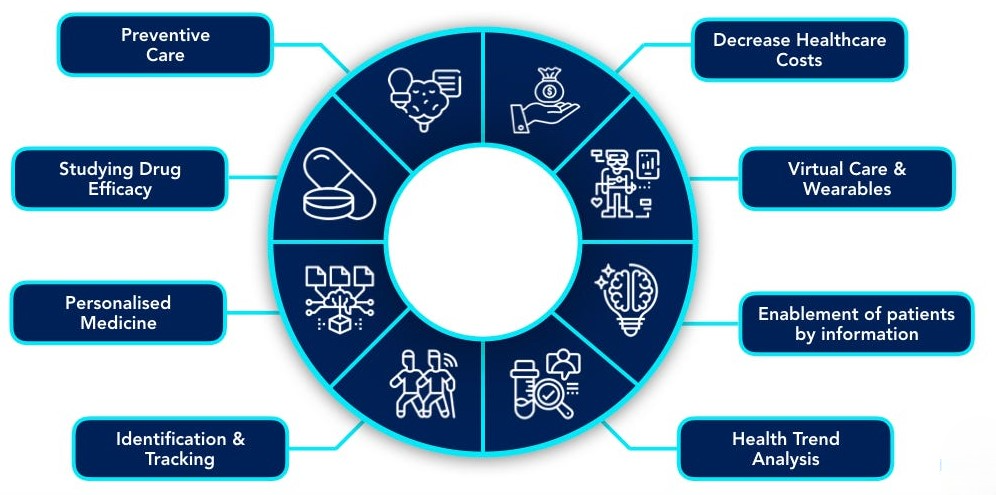
Types of Healthcare Data Patient, Clinical, and Operational
Healthcare data can be broadly classified into three categories: patient data, clinical data, and operational data. Patient data encompasses all information pertaining to the individual patient, including demographic details such as age, gender, and ethnicity, as well as medical and family histories, lifestyle choices, and vital signs like heart rate and blood pressure. This data is essential for personalizing patient care and tracking health over time. Clinical data, on the other hand, is gathered from interactions between patients and healthcare providers, such as doctor visits, diagnoses, test results, treatment plans, surgeries, medications, and patient outcomes. This type of data is vital for making evidence-based decisions and monitoring a patient’s health progress. For those interested in pursuing careers in healthcare analytics, it’s beneficial to Learn Data Science which can help professionals analyze and draw insights from healthcare data. Finally, operational data pertains to the administrative and operational aspects of healthcare organizations. It includes records related to staffing, resource management, patient throughput, billing, and financial operations. Operational data plays a crucial role in ensuring the efficient functioning of healthcare facilities, optimizing resource allocation, and supporting the overall management of healthcare delivery. Together, these three types of data, patient, clinical, and operational, provide a comprehensive view of the healthcare system, enabling organizations to deliver better patient care, improve operational efficiency, and make informed, data-driven decisions.
Data Collection and Cleaning in Healthcare
Data collection in healthcare typically involves extracting data from multiple sources like patient records, clinical databases, laboratory reports, and imaging systems. The challenge lies in the heterogeneity and volume of the data, as it often comes from various systems that use different formats and standards. Once data is collected, it needs to be cleaned and processed before analysis. Data cleaning is essential because raw healthcare data often contains errors, inconsistencies, missing values, and duplicates, which can skew analysis results. For those considering a career in healthcare data, understanding the Data Engineer Salary can provide insights into the potential earning opportunities in this field. The key steps in healthcare data cleaning include:
- Handling Missing Data: This may involve filling in missing values through imputation or simply removing rows with missing data if the impact is minimal.
- Standardizing Formats: Healthcare data often comes from different systems with inconsistent formats. Standardizing formats (e.g., date formats, measurement units) is crucial for consistency.
- Removing Duplicates: Duplicate records, which can arise when data is entered multiple times, need to be identified and removed.
- Addressing Inconsistent Data: Ensuring that data entries are consistent and reliable, such as aligning diagnostic codes or medication names to standardized lists.
Data cleaning ensures the accuracy and integrity of the data, which is fundamental for making reliable healthcare decisions.
Do You Want to Learn More About Data Science? Get Info From Our Data Science Course Training Today!
Predictive Analytics in Healthcare
Predictive analytics involves using statistical algorithms and machine learning techniques to forecast future outcomes based on historical data, and in healthcare, it plays a crucial role in anticipating patient health events, identifying at-risk populations, and guiding clinical decisions. One key application is disease prediction, where predictive models assess the likelihood of a patient developing conditions like heart disease, diabetes, or cancer by analyzing their medical history, lifestyle choices, and genetic factors. Another important use is hospital readmission prevention, where predictive analytics helps identify patients at high risk of being readmitted after discharge, enabling healthcare providers to implement targeted interventions aimed at reducing readmission rates. The development of Artificial Intelligence in India is greatly enhancing predictive analytics capabilities, especially in healthcare. Additionally, predictive analytics is instrumental in forecasting patient outcomes, allowing doctors to anticipate the potential success or complications of various treatments or surgeries, which helps in selecting the most appropriate course of action. Furthermore, predictive models can be leveraged to identify emerging health trends and disease outbreaks, offering valuable insights that allow healthcare systems to implement preventive measures and better plan for resource allocation. By providing data-driven insights, predictive analytics supports proactive healthcare, improving both patient care and operational efficiency within the healthcare system.
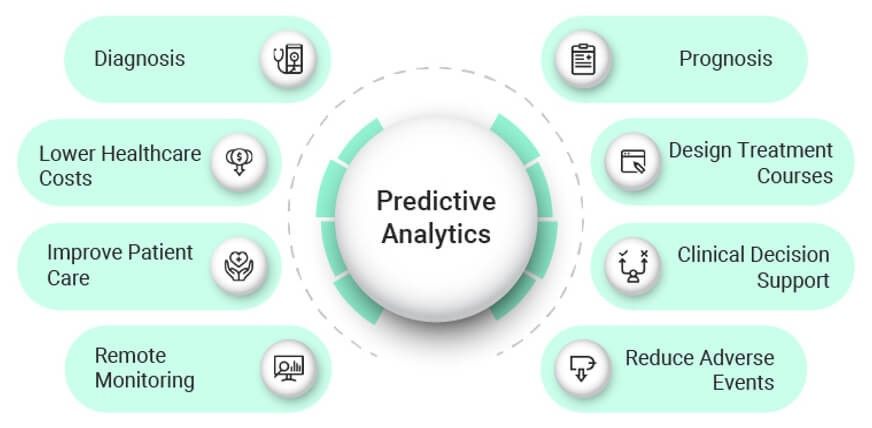
Applications of Machine Learning in Healthcare
Machine learning (ML), a subset of artificial intelligence (AI), has numerous applications in healthcare. By training models on large datasets, machine learning algorithms can uncover patterns that are too complex for traditional analytical methods to detect. Some key applications of machine learning in healthcare include:
- Medical Imaging Analysis: ML algorithms can analyze medical images such as X-rays, CT scans, and MRIs to identify abnormalities, diagnose diseases, and even detect early signs of conditions like cancer.
- Personalized Medicine: ML can help tailor treatment plans to individual patients based on their medical history, genetics, and response to previous treatments.
- Natural Language Processing (NLP): NLP helps process and analyze unstructured data from patient records, clinical notes, and research papers, allowing healthcare providers to gain insights from vast amounts of text data.
- Predicting Disease Progression: ML models can predict how certain diseases, like Alzheimer’s or cancer, may progress in a patient, aiding in timely interventions.
- Drug Discovery: ML algorithms are being used to analyze molecular data to identify potential new drugs, speeding up the drug discovery process.
Data Privacy and Security Regulations
Given the sensitivity of healthcare data, maintaining privacy and security is of utmost importance. Several regulations govern the collection, storage, and sharing of healthcare data to protect patient privacy and ensure compliance with ethical standards. One of the most significant regulations is HIPAA (Health Insurance Portability and Accountability Act) in the United States, which ensures the confidentiality and security of healthcare data by setting strict standards for electronic health information management. In the European Union, the GDPR (General Data Protection Regulation) provides a framework for safeguarding personal data, including healthcare data, while giving patients control over their data and ensuring transparency in data processing practices. Professionals pursuing Data Science Training are better equipped to understand these regulations while working with healthcare data. In the U.S., the HITECH Act promotes the adoption of health information technology and the meaningful use of Electronic Health Records (EHR) systems, while also ensuring that privacy and security standards are upheld. Beyond these, various countries have their own privacy and security regulations tailored to their healthcare systems, such as Canada’s PIPEDA (Personal Information Protection and Electronic Documents Act) and India’s Data Protection Bill. These laws collectively aim to create a secure environment for healthcare data, balancing the need for technological advancement with patient protection while fostering trust and ensuring that ethical standards are met in the management of sensitive health information across global healthcare systems.
Want to Pursue a Data Science Master’s Degree? Enroll For Data Science Masters Course Today!
Tools and Technologies in Healthcare Analytics
To handle the vast amounts of healthcare data and derive meaningful insights, various tools and technologies are employed, including:
- Data Warehousing: Tools like Amazon Redshift and Google BigQuery help aggregate and store large volumes of healthcare data for easier analysis.
- Business Intelligence Tools: Tools such as Tableau, Power BI, and Qlik Sense are commonly used for data visualization and reporting, enabling healthcare professionals to understand trends and make data-driven decisions.
- Data Integration Tools: Healthcare data often comes from multiple sources and formats, so data integration platforms like Apache Nifi, Talend, or MuleSoft are used to combine and standardize data.
- Machine Learning Platforms: Tools like TensorFlow, PyTorch, and Scikit-learn are popular for developing predictive models and AI applications in healthcare.
- Data Quality and Consistency: Data may be incomplete, inaccurate, or inconsistent, leading to flawed analyses and potentially incorrect conclusions.
- Data Privacy and Security: Ensuring the confidentiality of sensitive patient data is a significant challenge, particularly with increasing concerns about cyber threats.
- Integration of Data: Healthcare data often resides in multiple systems (EHRs, labs, imaging, etc.), and integrating it into a unified system for analysis can be complex.
- Regulatory Compliance: Adhering to data privacy regulations (like HIPAA and GDPR) while analyzing and sharing healthcare data can be cumbersome.
- Interoperability: Different healthcare organizations may use different systems that aren’t always compatible, making data sharing and collaboration difficult.
Real-World Use Cases: Disease Prediction, Diagnosis
Machine learning algorithms play a crucial role in healthcare by aiding in disease prediction and diagnosis. In disease prediction, these algorithms analyze patient data to assess the likelihood of developing conditions such as heart disease, diabetes, or hypertension. Predictive models can evaluate various factors like age, weight, smoking history, and cholesterol levels to identify patients at risk, enabling early intervention. To embark on this exciting field, understanding the Data Science Learning Path is key for those interested in machine learning and healthcare. In diagnosis assistance, machine learning models, trained on extensive datasets of medical images, help doctors diagnose diseases more accurately and swiftly. For example, ML algorithms can detect early-stage cancers in medical images, identifying abnormalities even before symptoms appear. This capability allows for earlier treatment, improving patient outcomes. By leveraging machine learning, healthcare systems can enhance predictive capabilities, offer more personalized care, and improve diagnostic accuracy, ultimately leading to better health management and timely interventions.
Challenges in Healthcare Data Analytics
While the benefits are clear, there are several challenges in healthcare data analytics:
Benefits of Data-Driven Decision-Making
The transition to data-driven decision-making in healthcare offers a wide range of benefits that significantly enhance both patient care and operational processes. One of the most notable advantages is improved quality of care, as data allows healthcare professionals to make more informed decisions based on accurate, real-time information. This leads to better treatment outcomes, as clinicians can tailor their approaches to individual patient needs. Moreover, understanding how data is gathered is crucial for these advancements. Understanding What is Data Collection is a foundational step in utilizing this information effectively. Additionally, data analytics plays a key role in improving operational efficiency within healthcare organizations. By analyzing data, hospitals and clinics can optimize resource allocation, reduce waste, and streamline patient flow, ensuring that staff and facilities are used effectively. Another important benefit is cost savings, as predictive analytics helps healthcare providers identify at-risk patients early, enabling them to implement preventive measures that reduce the likelihood of costly hospital readmissions. This not only saves money but also improves patient outcomes by addressing potential health issues before they escalate. Moreover, data-driven approaches enhance the patient experience by reducing wait times and streamlining administrative processes, allowing for more efficient and personalized care. By leveraging data, healthcare organizations can not only improve the overall quality of care but also increase operational efficiency, reduce costs, and ensure a better, more customized experience for patients, ultimately leading to a more effective healthcare system.
Future of Data Analytics in Healthcare
The future of healthcare data analytics holds immense potential, with several transformative developments on the horizon that promise to revolutionize the way healthcare is delivered and managed. One of the most significant trends is the integration of artificial intelligence (AI) and machine learning (ML), which will enable the creation of increasingly sophisticated predictive models and highly personalized treatment plans tailored to individual patient profiles. As AI continues to evolve, it will enhance the precision and efficiency of clinical decision-making, leading to better patient outcomes. In parallel, the widespread adoption of Internet of Things (IoT) devices and wearable technologies will drive the growth of real-time analytics, allowing for continuous health monitoring and immediate clinical interventions based on live data streams. For professionals looking to stay ahead in this evolving field, pursuing Data Science Training can help them develop the necessary skills to leverage these technologies effectively. This real-time capability will be crucial for managing chronic conditions and preventing acute health events. Additionally, the rise of precision medicine, fueled by advancements in genomics and bioinformatics, will see data analytics playing a central role in developing highly customized treatment strategies that consider a patient’s genetic makeup, lifestyle, and environment. The expansion of telemedicine, accelerated by the COVID-19 pandemic, will also benefit greatly from data analytics, which can help optimize remote healthcare delivery, track patient engagement, and ensure high-quality virtual care. Furthermore, big data analytics will contribute significantly to global health initiatives by enabling real-time surveillance and tracking of disease outbreaks, supporting early interventions, and strengthening public health responses. Altogether, these advancements mark a future where healthcare data analytics not only enhances individual patient care but also contributes to more efficient, responsive, and globally connected health systems.