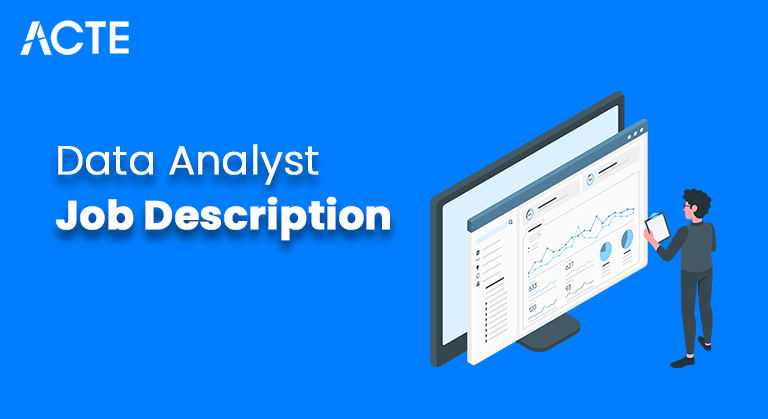
A data analyst is a person whose job is to collect and interpret data to decode a typical problem. The role includes an amount of time finished with data but entails getting findings too. Here’s what large data analysts do daily: Assemble data: Analysts continually contain data themselves.
- Introduction to Data Analyst
- What tools are used in Data Analyst?
- Features of Data Analyst
- Data Analyst Roles and Responsibilities
- Types of Data Analyst
- Syntax with examples
- How it Works
- Why Is Data Analyst Important?
- Trends of Data Analyst
- Benefits of Data Analyst
- Conclusion
Introduction to Data Analyst:
Conduct data cleaning/data matches to enhance data quality and design it for analysis and interpretation — recovering data in the correct format, deleting unneeded data, fixing spelling errors, etc. Collect data using Excel or Google Spreadsheets. This may contain editing data, making pivot tables, and more. Research and analyze data utilizing mathematical tools (i.e., finding relationships, trends, externalities, etc.).Show this data in logical ways: graphs, visuals, charts, tables, etc. Data analysts can write their completed files to project managers, department heads, and senior business leaders to help them drive findings and recognize patterns and trends. The good thing about data analysis is that it is part of the entry-level, which means you can get into basic details after accepting novice data analysis courses and hone a few key talents. (Yes, it won’t hurt if you already know how to write codes, math, or math!).
What tools are used in Data Analyst?
With the growing need for Data Analysts in the market, many tools have come up with different applications for this objective. Whether open or comfortable to use, the top market analytics tools are as follows:
R editing – This tool is a top analytics tool utilized for statistics and data modeling. R integrates and works on different platforms such as UNIX, Windows, and Mac OS. It also supplies tools to automatically install all packages according to particular user
Python – Python is an open-source, object-oriented programming language that is comfortable to read, write, and store. It delivers different task and viewing libraries like Scikit-Learn, TensorFlow, Matplotlib, Pandas, Keras, etc. It can also be incorporated into any platform such as SQL Server, MongoDB website, or JSON.
Public Tableau – This is free software that links to any data source like Excel, Company Data Warehouse, etc. Make old visuals, maps, dashboards, etc with real-time updates on the web.
SAS – Language planning and conditions for data manipulation and analysis, this tool is easily available and can interpret data from a variety of sources.
OpenRefine – Also known as GoogleRefine, this data filtering software will allow you to clean up data for analysis. It is utilized to clean dirty data, convert data, and different data from websites.
Apache Spark – Another great data processing engine, this tool makes applications in Hadoop clusters 100 times more rapid by memory and 10 times faster on disk. This tool is also famous for data piping and machine learning model product.
Features of Data Analyst:
1. Data Processing
Elements of data processing have the collection and processing of raw data to generate meaning. Data modeling creates complex data sets and displays all of them in a visual diagram or chart. This creates it easy to digest and straightforward to interpret for users who are attempting to use that data to drive decisions. The same goes for export goods – being able to take the said data sets and export them as PDFs, Excel files, Word files, or data files is necessary for operating and sharing data collected in earlier processes.
- Modeling
- Data Mining
- Data File Sources
- File Transfer
2. Guessful Requests
Identity management (or ownership and access management) is an organizational process that regulates who can access your data. The proprietary management function works to determine the data of everything accessed to the system including individual users, computer hardware, and software applications.
- Identity Management.
- Fraud Statistics.
3. Statistics
Big Data Analyst tools show different statistical packages and modules to give users choices. Risk statistics, for example, is a study of tension surrounding any given action. It can be utilized in conjunction with forecasting to underestimate the adverse effects of coming events. Risk statistics permit users to minimize these risks by absolutely defining and comprehending their organization’s tolerance and risk exposure.
Decision management affects decision-making methods to run a business. Decision-making modules treat findings as an asset. It includes technology in key places to make parts of that decision-making process.
Forecasting statistics is the next realistic step in analyzing statistics. This feature captures data gathered and analyzed, provides requirements for what is happening, and predicts future problems.
- Accident Statistics
- Decision Management
- Text stats
- Content statistics
- Mathematical Analysis
- Guessing statistics
- Social Media Analytics
4. Reporting Features
Reporting activities keep users at the top of their industry. Real-time reporting contains momentary data and sends it to you, usually in an intuitive dashboard format. This permits users to create faster findings in more time-limited situations and to be more ready and competitive in a fast-moving light society. Dashboards are visual recognition tools that current metrics and KPIs. They are usually customized to report to a detailed metric or data set. One example of geographically targeted data-based metrics – these data sets gathered from or locally sorted can get good knowledge about demographics.
- Real-Time Reporting
- Dashboards
- Location-Based Ideas
5. Safety Features
Maintaining your system safe is necessary for a thriving business. Big Data Analysis tools should deliver security components to provide security and safety. One such element is logging in once. Also called SSO, it is a verification service that offers users one set of login points to access numerous applications.
Data Analyst Roles and Responsibilities:
The data analyst acts as a gatekeeper for an organization’s data so stakeholders can comprehend data and use it to drive strategic business conclusions. It is a technical role that needs an undergraduate degree or master’s degree in analytics, computer modeling, science, or math.
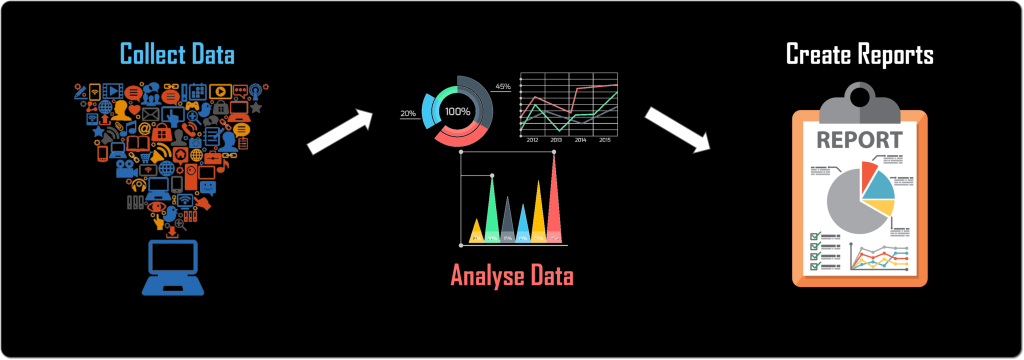
Types of Data Analyst:
Four main types of data analysis:
1. Analyze the prediction data
Guessing statistics can be the considerable widely accustomed phase of data analysis. Businesses use predictive statistics to determine trends, relationships, and causes. The class can also be divided into predictive modeling and mathematical modeling; However, it is essential to note that both are consistent.
For example, a T-shirt advertising campaign on Facebook can use predictive statistics to decide how close the conversion rate is connected to the country’s target audience area, revenue bracket, and interests. From there, the desired model can be used to analyze two (or more) target audience formations, and supply potential revenue levels for each location.
2. Written data analysis
Continuous statistics are where AI and big data come together to support predicted results and decide what steps to take. This mathematical category can also be subdivided into random development and randomized testing. With the use of advances in ML, written analysis can support answer questions such as “What if we try this?” and “What is the best action?”
3. Analyze diagnostic data
Although not as exciting as predicting the future, analyzing past data can serve a useful purpose in directing your business. Diagnostic data analysis is the process of analyzing data to understand the cause and effect or why something happened. Strategies like drag, data acquisition, data mining, and integration are often used.
Discover and notifications let you know of a potential problem before it occurs, for example, a notice about the low number of workers’ hours, which could guide to a drop in closed deals. You can also use diagnostic data statistics to “get” data like a candidate that is best suitable to get a new job in your business.
4. Descriptive data statistics
Descriptive statistics are unbelievable to have business intelligence (BI) tools and dashboards without them; it was also called the backbone of reporting. The descriptive statistics can be further split into two categories: (1) ad hoc reporting (2) canned reports. The canned report is pre-designed and includes information about a shared topic. An example of this is a monthly report sent by your ad company or ad team outlining execution metrics for your recent ad efforts.
Syntax with examples
- # Let’s create a data.frame
- df = data.frame (numbers = 1:26, characters)
- head (df)
- # number letters
- # 11a
- # 22b
- # 33c
- # 4 4d
- # 5 5 e
- # 6 6f
- #Str provides data structure. Framework, a good summary to test an object
- str (df)
- # ‘data.date’: 26 obs. of 2 changes:
- # $ numbers: int 1 2 3 4 5 6 7 8 9 10 …
- # $ characters: Feature w / 26 levels “a”, “b”, “c”, “d”, ..: 1 2 3 4 5 6 7 8 9 10 …
- # This indicates that the character vector was forced as a feature.
- # This can be defined by strings as factors = TRUE argument in data. frame
- # read? data. frame for more information
- section (df)
- # [1] “data.frame”
- #
- #
- # Index
- # Find the first line
- df [1,]
- # number letters
- # 11a
- # Used for standard editing – returns the output as a list
- df [1,, drop = TRUE]
- # $ numbers
- # [1] 1
- #
- # Books
- # [1] a
- # Levels: a b c d e f g h I j k l m n o p q r s t u v w x y z
- # Find several lines of data.due
- df [5: 7,]
- # number letters
- # 5 5 e
- # 6 6f
- # 77g
- #
- #
- # Add one column that combines a number column with a feature column
- df $ mixed = paste (df $ numbers, df $ letters, sep = ’’)
- str (df)
- # ‘data.date’: 26 obs. of the 3 variables:
- # $ numbers: int 1 2 3 4 5 6 7 8 9 10 …
- # $ characters: Feature w / 26 levels “a”, “b”, “c”, “d”, ..: 1 2 3 4 5 6 7 8 9 10 …
- # $ mixed: chr “1a” “2b” “3c” “4d” …
- #
- #
- # Find the columns
- # Get the first column
- df [, 1]
- # Returns the one-sided vector with each column
- # Find two columns
- df2 = df [, 1: 2]
- head (df2)
- # number letters
- # 11a
- # 22b
- # 33c
- # 4 4d
- # 5 5 e
- # 6 6f
- # Find the first and third columns
- df3 = df [, c (1, 3)]
- df3 [1: 3,]
- # mixed numbers
- # 1 11a
- # 2 22b
- # 3 3 3c
- #
- #
- # Index columns in their names
- words (df)
- # [1] “numbers” “characters” “mixed” “
- # This is a very good practice of making plans, as often indices change, however
- Flexible words are missing
- # We create variables with the words we want to set
- keep_vars = c (“numbers”, “mixed”)
- df4 = df [, keep_vars]
- head (df4)
- # mixed numbers
- # 1 11a
- # 2 22b
- # 3 3 3c
- # 4 44d
- # 5 5 5e
- # 6 6 6f
- #
- #
- # subset of rows and columns
- # Keep the first five lines
- df5 = df [1: 5, keep_vars]
- df5
- # mixed numbers
- # 1 11a
- # 2 22b
- # 3 3 3c
- # 4 44d
- # 5 5 5e
- # Lower set lines use logical state
- df6 = df [df $ numbers
R delivers the type of data for each type of variable. The ordered part is rarely utilized but can be made by a function feature, or an order.
The next area deals with the idea of identification. This is a normal process, and you face the issue of choosing parts of an object and making modifications to it.
How it Works:
- Data Needs Specification
- Data Collection
- Data Processing
- Data Cleaning
- Data Analysis
- Communication
Data Analysis is the method of collecting, converting, cleaning, and modeling data to get the necessary information. Results are communicated, suggesting conclusions, and help decision-making. Data viewing is occasionally employed to display data to create it easier to find proper patterns in data. The words Data Model and Data Analysis mean the same thing.
The Data Analysis Process consists of the next phases that repeat naturally –
Data Needs Specification
The data needed for analysis is founded on the question or test. The conditions of those who direct the analysis, the data needed as input into the analysis is determined (e.g., population). Specific interpretations in demographics (e.g., age and income) may be identified and caught. Data can be numbered or ordered.
Data Collection
Data Collection is the method of gathering information. The focus is on providing accurate and dependable data collection. Data Collection guarantees that the data contained is so real that corresponding decisions are valid. Data Collection delivers both the measurement scale and the target to be enhanced.
Data Processing
The data collected must be processed or filtered for analysis. This concerns managing the data as needed by the proper analysis tools. For example, data may require to be placed in rows and columns in a table within a Spreadsheet or Application Statistics. You may require to make a Data Model.
Data Cleaning
Processed and filtered data may be insufficient, contain duplicates, or contain errors. Data Cleaning stands are used to control and correct these errors. Several kinds of data purification depend on the type of data. For instance, when refining financial data, certain parts may be reached with the published numbers or limited limitations. Also, quantitative data techniques can be utilized to detect foreign objects that can be removed from the analysis.
Data Analysis
The data being processed, processed, and developed will be prepared for analysis. Different data analysis methods are known for comprehension, interpretation, and conclusions founded on conditions. Data Visibility can also be utilized to scan data in image format, for further knowledge of in-data messages.
Why Is Data Analyst Important?
Data analysis is required as a result of it allowing businesses to improve their performance. Applying it to a business model means companies will enable cut-back prices by distinguishing the foremost practical ways to try to do business. The company may also use detailed analysis statistics to create higher business selections and enable analyzing trends and client satisfaction, which might cause new merchandise and services — and more elevated and higher.
Trends of Data Analyst:
1.Enlecting Indigenous Languages
Indigenous language processing (NLP) is equivalent to Google’s data statistics because it permits users to ask questions in their native language, in writing, or by voice. This technology creates data statistics available to a broad assortment of experts, including former employees.
2.Augmented Analytics
Using machine learning and learning techniques, the advanced analysis supplies users with a systematic way of getting the most critical information. It does this by automatically compiling company or community data, analyzing it, and recovering potentially useful information. This process takes a short amount of time for manual analysis. It may decrease the demand for data science experts and machine learning experts while demanding experts in other fields (such as small business owners) to enhance their data knowledge.
3.Blockchain
Blockchain technology is best understood for its role in cryptocurrency but can be used to serve various roles across enterprises. Blockchain has the potential to enhance predictive analytics because it demonstrates data authenticity, containing false data from being incorporated in the analysis. The hacker will need to restore all blocks within the blockchain to disrupt the data. In many cases, this action is more severe than it deserves. Therefore, the data gathered is reliable and therefore very important.
4.Continuous wisdom
Continuous intelligence can also be called real-time intelligence. This kind of data query is becoming more and more every day as technology such as cloud, live streaming software, machine learning, and Internet of Things (IoT) are producing more and more connected and connected. According to Dataversity, “It processes historical and present data to deliver automated decision-making support,” and, “Guides actions based on both recorded and real-time data.”
5.Cloth of Data
Fabric data enables seamless data sharing across the whole spread network. Gartner describes it as “a custom plan that delivers usable data services, pipelines, semantic tiers, or APIs with a combination of data integration methods.” In other words, data analysis applications can combine data from various sources and create significant connections utilizing all data streams.
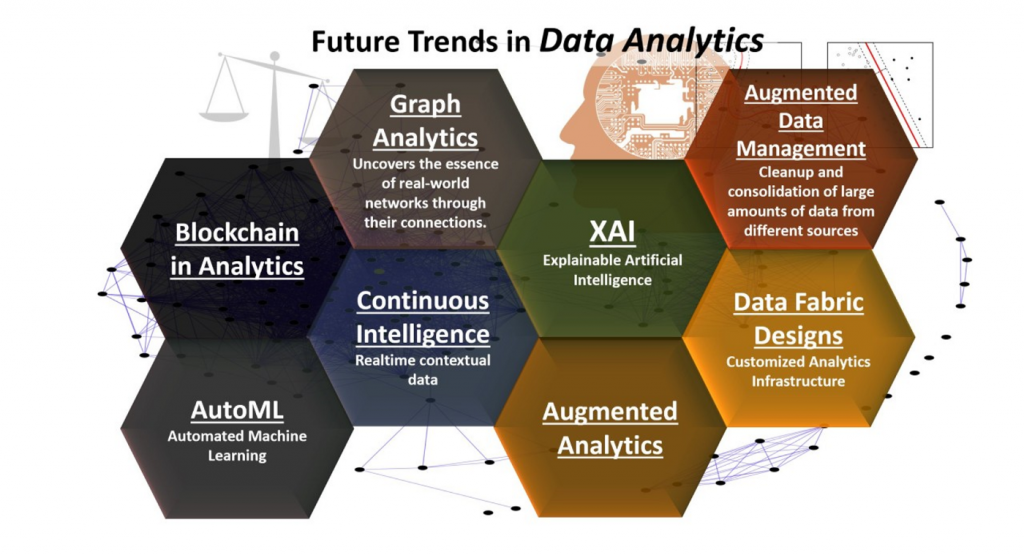
Benefits of Data Analyst
1. Customize the customer’s feeling
Businesses gather consumer data from a wide assortment of channels, including prostitution, e-commerce, and social media. By utilizing data statistics to build complete customer profiles from this data, businesses can obtain data about customer behavior to deliver personalized feedback.
The unit may analyze its sales data and data from its social media pages and develop targeted social media campaigns to boost e-commerce sales of product classes that customers are already curious about. For example, an entity may model differences in prices or product offerings to choose how those differences may affect consumer demand.
3. Prepare tasks
Associations can enhance efficiency via data analysis. Gathering and analyzing data about supply chains can show where production delays or issues start and support predict where future problems may arise. If a market forecast shows that a certain seller will not be able to control the needed volume during the holidays, the group may add or replace this seller to evade production delays.
4. Reduce risk and catch obstacles
Risks are about in business. It has a customer or employee theft, uncollected receipts, employee safety, and legal liability. Data analysis can assist an organization to comprehend the threats and take preventive measures. For example, a sales chain can use a trend model – a statistical model that can anticipate future actions or events – to decide which stores are most at risk of theft. A commodity may use this data to select the amount of security needed in stores, or whether it should be from any location.
5. Improve security
All companies face data security threats. Communities can use data analysis to define the reasons for past data violations by processing and determining correlations.
Conclusion
Big Data is changing the game. Many institutions use additional analysis to guide strategic actions and deliver better client knowledge. A small change in inefficiency or extremely small savings can lead to huge profits, which is why many communities move on to big data.