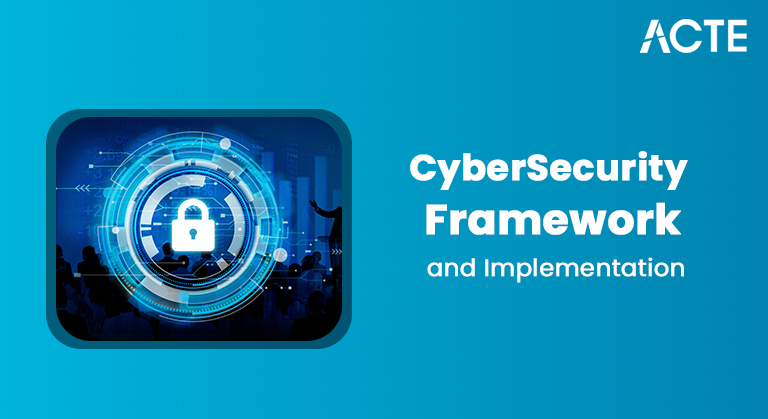
- Introduction to the Data Analyst Career Path
- Skills and Technologies for Data Analysts
- Educational Requirements and Certifications
- Entry-Level Data Analyst Roles
- Mid-Level Data Analyst Roles
- Senior-Level Data Analyst Roles
- Transitioning to Data Scientist or Data Engineer
- Key Tools: SQL, Excel, Python, and Power BI
- Industry-Specific Data Analyst Roles
- Freelance and Remote Opportunities
- Salary Trends and Career Growth
- Future Scope and Emerging Trends
Introduction to the Data Analyst Career Path
The career path of a data analyst blends analytical prowess, business insight, and technical knowledge, making it a vital role in today’s data-driven world. Data analysts are responsible for interpreting vast amounts of information to support organizational decision-making processes and play a crucial role across a wide range of industries, including finance, healthcare, marketing, and technology, as the exponential growth of data has created an increasing demand for professionals capable of turning raw information into actionable insights. Many aspiring professionals begin their journey through Data Science Training which helps build foundational skills in data collection, cleaning, trend analysis, and the use of tools like Excel, SQL, and data visualization platforms to identify patterns and present findings effectively. As analysts gain experience, they may take on more complex projects, contribute to predictive modeling, or transition into roles such as data scientists or data engineers, which require advanced knowledge of programming languages like Python or R, along with expertise in machine learning and big data technologies. This career path not only enhances technical expertise but also sharpens communication and problem-solving skills, which are essential for influencing business strategies. With significant opportunities for growth whether through specialization or leadership becoming a data analyst is a dynamic and rewarding choice in the age of information.
Would You Like to Know More About Data Science? Sign Up For Our Data Science Course Training Now!
Skills and Technologies for Data Analysts
To succeed in the data analyst career, professionals must develop a diverse skill set that combines technical and analytical capabilities. Some essential skills and technologies include:
- Data Manipulation and Analysis: Data analysts should be proficient in handling large volumes of data, cleaning it, and organizing it for further analysis. Familiarity with data manipulation tools and techniques is crucial.
- Statistical Analysis: Knowledge of statistical techniques, including hypothesis testing, regression analysis, and probability, is vital for interpreting data.
- Database Management: A data analyst needs to understand relational databases, including how to design and query databases using languages such as SQL. Understanding how to optimize database performance is also crucial.
- Data Visualization: The ability to present data clearly and understandably is a vital skill for a data analyst. Tools like Power BI, Tableau, and Excel are commonly used to create reports and dashboards.
- Programming Languages: While SQL is the primary language for data analysts, knowledge of Python or R is increasingly important for performing more complex analysis, automation, and data processing tasks.
- Business Acumen: Data analysts must understand the business they work for and the key metrics that drive the organization’s success. This helps in translating data into actionable business insights.
- Excel: Excel remains one of the most widely used tools for data analysis. A data analyst must be comfortable using Excel functions, pivot tables, and other advanced features.
- Communication: A data analyst must possess strong communication skills to convey complex findings in a way that stakeholders can understand.
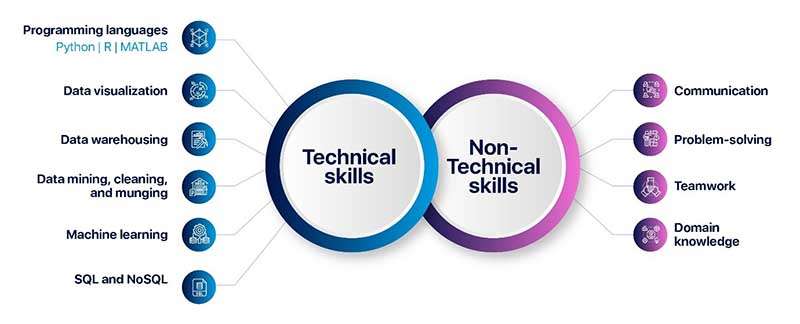
Educational Requirements and Certifications
Entering the field of data analysis generally requires a strong educational foundation in disciplines such as mathematics, statistics, computer science, or business analytics, though it is possible to break into the field without a formal degree specifically in data analytics if the candidate acquires relevant and practical knowledge. A bachelor’s degree is typically the minimum educational requirement, with preferred majors including mathematics, statistics, computer science, or related areas. Within these programs, coursework in statistics, data management, programming languages such as Python or R, and business management can be particularly beneficial for aspiring analysts. Many individuals also explore a structured Data Science Learning Path to guide their development, combining formal education with targeted skill-building in essential tools and techniques. In addition to undergraduate education, some professionals choose to pursue a master’s degree in data science, business analytics, or other closely related fields to deepen their understanding of advanced analytical techniques, enhance their expertise, and improve their competitiveness in the job market. For those looking to further demonstrate their skills and commitment, earning industry-recognized certifications can be highly advantageous. Certifications help professionals stay updated with current tools and technologies while signaling proficiency to employers. Notable certifications in the field include the Microsoft Certified: Data Analyst Associate (focused on Power BI), the Certified Analytics Professional (CAP), the Google Data Analytics Professional Certificate, the SAS Certified Data Scientist, and the IBM Data Science Professional Certificate. These credentials cover a wide range of essential data analysis competencies, from data visualization and statistical analysis to machine learning and data management, thereby helping candidates stand out in a competitive job landscape.
Entry-Level Data Analyst Roles
Entry-level data analysts typically work with more structured data and are responsible for basic data analysis tasks. These roles are a starting point to understand data operations within a business. Responsibilities at this level include:
- Cleaning and organizing raw data.
- Creating basic reports and visualizations.
- Assisting senior analysts in more complex tasks.
- Generating insights from historical data.
- Maintaining and updating data in databases.
- Junior Data Analyst
- Data Analyst Intern
- Research Analyst
Common titles for entry-level positions include:
At this stage, analysts are gaining foundational skills in SQL, Excel, and basic reporting tools.
Do You Want to Learn More About Data Science? Get Info From Our Data Science Course Training Today!
Mid-Level Data Analyst Roles
After accumulating experience in entry-level roles, data analysts often progress to mid-level positions where they are tasked with handling more complex and strategic data-related responsibilities. At this stage, professionals are expected to possess a more comprehensive understanding of data analytics processes, including advanced data cleaning, sophisticated data visualization, and the use of powerful analytical tools and programming languages. Their work typically involves developing dynamic dashboards and comprehensive reports tailored to both internal and external stakeholders, enabling data-driven decision-making across various departments. Mid-level analysts are also responsible for conducting advanced statistical analysis and predictive modeling to uncover deeper insights and trends. Additionally, they are expected to interpret complex data patterns and translate their findings into actionable business recommendations that support organizational goals. In the course of their work, they often need to understand different types of data, such as categorical and continuous, and concepts like What is Nominal Data to ensure accurate analysis and reporting. These professionals frequently collaborate with cross-functional teams such as marketing, operations, and finance to understand diverse business needs and ensure that their analyses are aligned with strategic objectives. Common job titles at this level include Data Analyst, Business Intelligence Analyst, and Marketing Analyst, depending on the industry and focus area. Moreover, mid-level analysts often take on mentorship responsibilities, guiding junior analysts, providing feedback, and overseeing the quality and accuracy of their work. This stage not only deepens technical and analytical skills but also introduces leadership and project management experience, preparing analysts for potential transition into senior or specialized roles within the data domain.
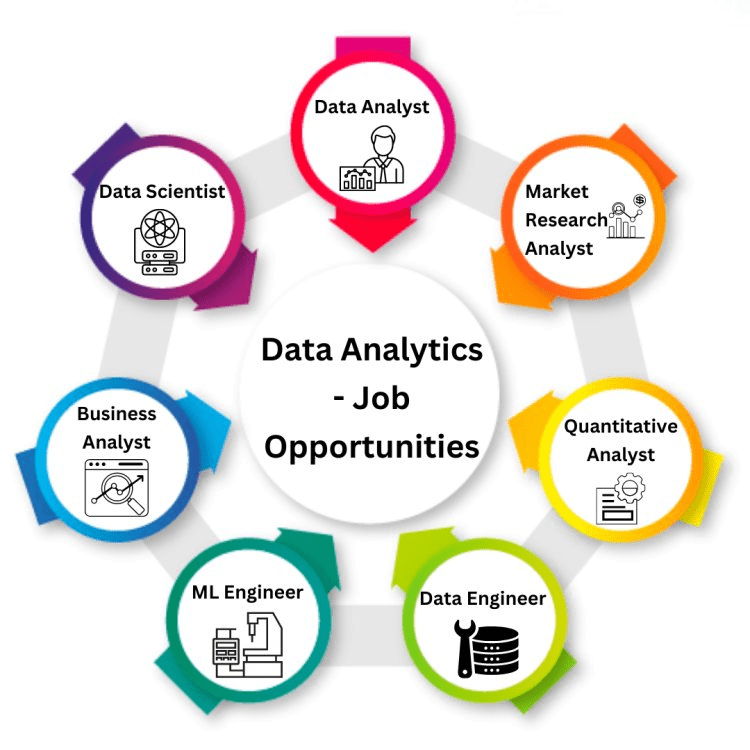
Senior-Level Data Analyst Roles
Senior data analysts possess deep technical and business expertise. At this level, they are not only expected to conduct advanced data analysis but also to manage projects, lead teams, and provide strategic insights to top management. Senior analysts often work on high-priority projects that require specialized knowledge of business operations, industry trends, and advanced analytics techniques.
Responsibilities include:- Leading the development of data strategies and systems.
- Conducting predictive modeling and advanced analytics.
- Advising on data governance and best practices.
- Managing and mentoring teams of data analysts.
- Communicating complex findings to non-technical stakeholders. Common titles for senior roles include:
- Senior Data Analyst
- Senior Business Intelligence Analyst
- Analytics Manager
At the senior level, analysts may start shifting their focus to leadership roles within their organization, combining technical skills with managerial responsibilities.
Transitioning to Data Scientist or Data Engineer
Data analysts often choose to transition into more specialized roles within the data field, such as data scientist or data engineer, especially when seeking to deepen their technical expertise or expand their career opportunities. These roles build upon the foundational skills of a data analyst but demand a more advanced understanding of specific technologies and methodologies. Many professionals prepare for this shift through Data Science Training which provides the necessary knowledge in areas like machine learning algorithms, statistical modeling, and programming languages such as Python or R. Transitioning to a data scientist role typically requires designing and implementing complex models and predictive algorithms to uncover hidden patterns and generate actionable insights from vast and often unstructured datasets. These models support strategic decisions and drive innovation across various industries. On the other hand, data engineers focus on the architecture and systems that enable effective data usage. Their responsibilities include building, optimizing, and maintaining data infrastructure that supports collection, processing, and storage, often using big data technologies and cloud platforms such as Hadoop, Apache Spark, AWS, and Google Cloud. They ensure data pipelines are scalable, efficient, and reliable, playing a key role in maintaining data quality and availability. Both paths require a higher level of technical competence than typical data analyst positions and offer excellent opportunities for career advancement, including leadership roles, increased earning potential, and participation in innovative, large-scale projects within the data science and engineering landscape.
Want to Pursue a Data Science Master’s Degree? Enroll For Data Science Masters Course Today!
Key Tools: SQL, Excel, Python, and Power BI
Data analysts use a variety of tools to help with the analysis, visualization, and interpretation of data. Some key tools include:
- SQL (Structured Query Language): SQL is the standard language for querying relational databases. It allows analysts to retrieve, filter, and aggregate data.
- Excel: Despite the rise of more specialized tools, Excel remains one of the most commonly used tools for data analysis. Its ability to handle large datasets, perform calculations, and visualize data makes it indispensable for entry-level and mid-level analysts.
- Python: Python is increasingly used for data analysis due to its versatility and power. With libraries such as Pandas, NumPy, and Matplotlib, Python allows analysts to perform complex data manipulations, statistical analysis, and create data visualizations.
- Power BI: Power BI is a business intelligence tool developed by Microsoft that enables data analysts to create interactive reports and dashboards. Its integration with Excel and SQL databases makes it particularly useful for analysts in business and finance.
- Entry-Level: $50,000 – $70,000 per year.
- Mid-Level: $70,000 – $100,000 per year.
- Senior-Level: $100,000 – $130,000 per year.
Industry-Specific Data Analyst Roles
Data analysis plays a critical role across nearly every industry, which has led many data analysts to specialize in specific sectors based on their interests or the needs of the market. This specialization allows analysts to develop domain-specific knowledge and deliver more targeted insights. In the healthcare industry, data analysts are essential for enhancing patient care, reducing operational costs, and ensuring compliance with regulatory standards. They work extensively with patient records, clinical data, and healthcare trends to identify patterns that can lead to better outcomes and more efficient practices. In the finance sector, data analysts focus on assessing financial risk, forecasting market behavior, and refining investment strategies. Their work often involves handling large datasets, applying statistical methods, and utilizing financial modeling techniques to inform high-stakes decisions. Within marketing, analysts are responsible for interpreting consumer behavior, tracking campaign performance, and fine-tuning marketing efforts. They use tools like web analytics, customer databases, and A/B testing to provide actionable insights that help businesses reach their target audiences effectively. In the retail industry, data analysts examine sales trends, customer purchasing behavior, and inventory performance to enhance pricing strategies, manage stock efficiently, and improve the overall customer experience. By leveraging point-of-sale systems and customer feedback, retail analysts help companies maximize profitability and stay competitive. Additionally, Artificial Intelligence in India is increasingly influencing how data analysts approach tasks in various sectors, creating new opportunities for innovation. These examples illustrate how the core skills of a data analyst can be applied in diverse ways depending on the industry’s specific challenges and objectives, making specialization an attractive and valuable path for professionals in this field.
Freelance and Remote Opportunities
With the growth of remote work, data analysts now have more opportunities to work in flexible environments, either as freelancers or in fully remote positions. Freelance data analysts collaborate with clients from various industries to analyze datasets, create reports, and deliver insights that support business decisions. These roles offer significant flexibility, including the ability to choose projects, set schedules, and work with clients from around the world. Remote data analyst positions also provide the chance to gain diverse experience and build a global portfolio. Popular freelance platforms like Upwork, Fiverr, and Freelancer make it easier for analysts to connect with companies seeking both short-term and long-term project support, offering a valuable entry point for those looking to work independently.
Salary Trends and Career Growth
Salaries for data analysts vary based on experience, location, and industry. Here is a general idea of salary trends for data analysts:
The demand for data analysts is expected to grow steadily, driven by the increasing need for data-driven decision-making across industries. The Bureau of Labor Statistics (BLS) projects a 25% growth in the employment of data scientists and mathematicians between 2019 and 2029.
Future Scope and Emerging Trends
The future of data analysis is highly promising, as more organizations depend on data-driven strategies to guide their decision-making processes. As the field evolves, several key trends are shaping the landscape and redefining the role of data analysts. One significant trend is the integration of artificial intelligence and machine learning into data analysis, allowing analysts to manage more complex datasets and automate repetitive tasks, thereby improving both efficiency and insight generation. Another major development is the growing reliance on big data technologies; as businesses generate vast amounts of information, data analysts are increasingly expected to work with tools such as Hadoop and Apache Spark to process and analyze large-scale datasets effectively. Cloud computing is also becoming central to the profession, with platforms like AWS, Google Cloud, and Microsoft Azure enabling scalable data storage and powerful analytics solutions that support collaboration and remote work. In parallel, there is an increasing emphasis on data governance and ethics, with rising concerns about data privacy and security pushing analysts to adhere to stricter regulations and ethical standards. To meet these evolving demands, many professionals are turning to Data Science Training to enhance their expertise in analytics, cloud technologies, and responsible data practices. Overall, the career outlook for data analysts remains strong, with growing opportunities across industries such as finance, healthcare, marketing, and technology. Those who continue to upskill are well-positioned to advance into senior roles or pivot into related areas like data science or data engineering, paving the way for long-term career growth.