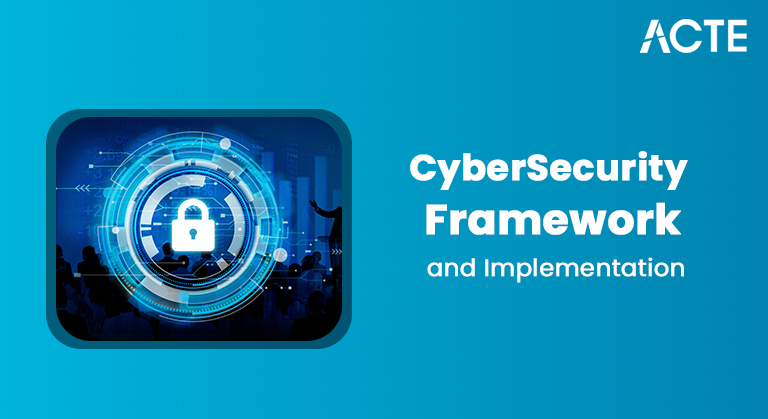
- Definition and Importance of Data
- Types of Data
- Data Storage and Databases
- Data Processing and Transformation
- Applications of Data in Various Industries
- Big Data and Its Significance
- Future of Data-Driven Technologies
- Conclusion
Definition and Importance of Data
Data is a collection of facts, figures, measurements, or observations used to analyze and derive meaningful insights. It can take many forms, such as numbers, text, images, and audio, and plays a critical role in today’s digital age. Data has become an invaluable asset that fuels decision-making, drives automation, and fosters innovation across various industries. Its importance lies in its ability to support business intelligence and analytics, helping organizations make informed decisions based on trends and patterns. Additionally, data is key to improving operational efficiency by streamlining processes and identifying areas for optimization, a fundamental aspect of Data Science Training. In fields like scientific research and technological advancements, data enables breakthroughs by providing the foundation for experimentation and hypothesis testing. It also enhances decision-making by offering data-driven insights that inform strategies and reduce uncertainty. Moreover, data is essential for automation and artificial intelligence applications, allowing machines to learn, adapt, and optimize tasks. It plays a significant role in customer relationship management and marketing strategies, helping businesses understand customer preferences and tailor their approaches. Ultimately, data provides a solid foundation for both predictive and prescriptive analytics, guiding future actions and outcomes.
Are You Interested in Learning More About Data Science? Sign Up For Our Data Science Course Training Today!
Types of Data
- Semi-Structured Data: Partially organized data that does not conform to a strict schema but contains metadata. Examples include XML, JSON, and NoSQL databases.
- Unstructured Data: Is data without a predefined format, making it harder to process. Examples include emails, social media posts, images, videos, and audio files, which are key areas of focus in Data Analytics.
- Structured Data: Organized and stored in databases with a predefined schema, such as relational databases (SQL). Examples include customer records, sales transactions, and employee databases.
- Metadata: Data that provides information about other data, often used to describe the structure, content, or context of data. Examples include file properties, database schema descriptions, and tags in images.
- Real-Time Data: Data that is continuously generated and updated in real time, often used for applications requiring immediate insights or actions. Examples include live stock market feeds, sensor data, and GPS tracking.
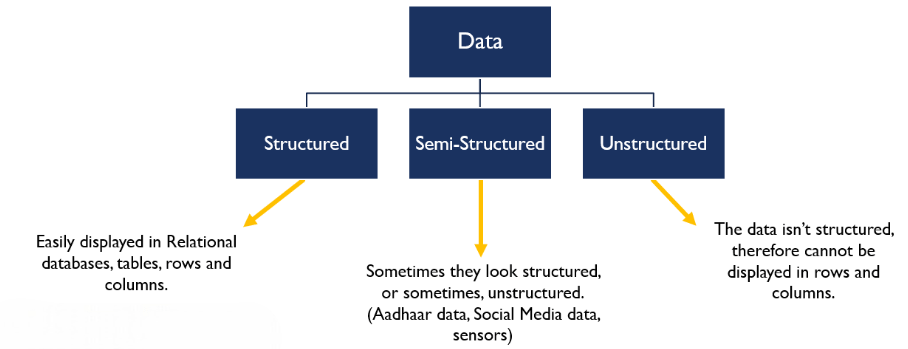
Data Storage and Databases
- Cloud Storage provides scalable, on-demand data storage services over the internet. Popular services include Amazon S3, Google Cloud Storage, and Microsoft Azure.
- Backup and Recovery are essential for ensuring data safety and integrity. Regular backups protect data from loss due to hardware failures, cyber-attacks, or human error.
- Data Storage refers to the process of saving and organizing data for easy access, retrieval, and management. It is essential for businesses and organizations to maintain large volumes of data efficiently.
- Databases are structured collections of data, stored and accessed electronically, playing a crucial role in Data Management in Organizations.
- Relational Databases (RDBMS) use tables to store data in rows and columns, with predefined relationships between the tables. Examples include SQL databases like MySQL, PostgreSQL, and Oracle.
- Data Warehouses store large volumes of historical data and are optimized for analytical queries. They integrate data from multiple sources, allowing businesses to make data-driven decisions.
- NoSQL Databases offer more flexible storage models and are designed to handle unstructured or semi-structured data. They are ideal for handling large-scale, dynamic, and distributed data. Examples include MongoDB and Cassandra.
- Veracity: The quality and accuracy of Big Data can vary. Ensuring data reliability is essential to avoid making incorrect decisions based on flawed information.
- Velocity: Big Data is generated at high speed, with real-time data streaming in from sources like online activity, sensors, and devices. This rapid influx requires immediate processing to derive timely insights.
- Variety: Big Data comes in various formats, including text, images, videos, and audio. Managing this diverse range of data requires advanced tools and techniques for integration and analysis.
- Data Analytics: Big Data analytics allows businesses and organizations to uncover hidden patterns, correlations, and trends that drive decision-making, innovation, and competitive advantage, particularly in the realm of Data Science in Finance.
- Impact: Big Data plays a transformative role across industries, enabling advancements in healthcare, finance, marketing, and more by providing deeper insights into customer behavior, operational efficiency, and market trends.
- Definition of Big Data: Big Data refers to vast, complex datasets that are too large or intricate to be processed using traditional data management tools. It includes structured, semi-structured, and unstructured data from various sources.
- Volume: One of the defining characteristics of Big Data is its sheer volume. It encompasses massive amounts of information generated daily, such as from social media, sensors, or business transactions, requiring specialized tools for storage and analysis.
To Explore Data Science in Depth, Check Out Our Comprehensive Data Science Course Training To Gain Insights From Our Experts!
Data Processing and Transformation
Raw data is often incomplete, inconsistent, or redundant, necessitating processing and transformation before it can be analyzed effectively. Data cleaning is one of the first steps, where duplicates are removed, errors are corrected, and missing values are handled to ensure the data is accurate and reliable. Data normalization follows, where data is structured to minimize redundancy and ensure consistency, particularly in large datasets with multiple variables, a key step in Classification in Data Mining. Data aggregation helps by summarizing raw data into more manageable and insightful forms, often by grouping or combining data points to reveal patterns or trends. Next, data transformation is performed to convert raw data into a suitable format for analysis, such as converting categorical data into numerical values for machine learning models.
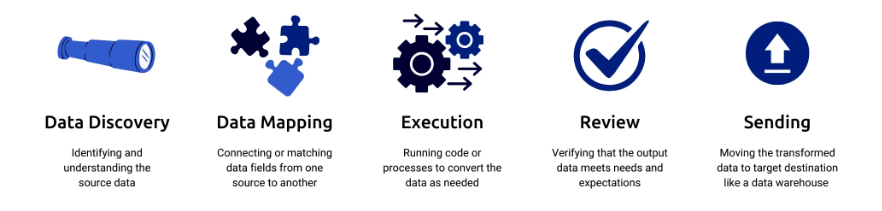
The ETL (Extract, Transform, Load) process plays a key role in moving data from various sources into a centralized data warehouse, making it easier to analyze and derive insights. Lastly, feature engineering involves creating new features from raw data that can enhance the performance of machine learning models. Together, these techniques ensure that raw data is transformed into high-quality, actionable insights.
Applications of Data in Various Industries
Data is a crucial element across various industries, driving innovation and enhancing decision-making processes. In healthcare, data plays a vital role in patient diagnosis, personalized treatment plans, and medical research, improving health outcomes. In finance, data is used for fraud detection, risk assessment, and analyzing stock market trends, enabling more informed financial decisions. The retail sector leverages data to analyze customer behavior, optimize inventory management, and power recommendation systems, enhancing customer experiences and driving sales, all key topics covered in Data Science Training. In manufacturing, data is utilized for predictive maintenance, ensuring equipment reliability, and optimizing supply chains for cost-efficiency. Education benefits from data through personalized learning experiences and student performance analysis, helping educators tailor instruction to individual needs. Government agencies use data for policy-making, census analysis, and urban planning, enabling efficient allocation of resources and improving public services. In agriculture, data is used for yield prediction, soil analysis, and weather forecasting, supporting sustainable farming practices. Lastly, in transportation, data aids in traffic monitoring and route optimization, ensuring smoother and more efficient travel. Across all these sectors, data drives advancements and supports better decision-making.
Are You Considering Pursuing a Master’s Degree in Data Science? Enroll in the Data Science Masters Course Today!
Big Data and Its Significance
Future of Data-Driven Technologies
The future of data is driving technological advancements across multiple fields, reshaping how we collect, process, and leverage information. Artificial Intelligence (AI) and Machine Learning (ML) are evolving through data-driven learning, with AI models continuously improving as they process more data, enabling smarter, more efficient systems. Edge computing is enhancing real-time decision-making by processing data closer to its source, reducing latency and improving response times, which is especially crucial for applications like autonomous vehicles and smart cities. In the realm of data security, blockchain technology is making strides in ensuring data integrity through decentralized systems, providing secure and transparent ways to track data without the risk of tampering, which is one of the Top 8 Data Science Applications. The Internet of Things (IoT) and smart devices are further expanding real-time data collection, facilitating automation and predictive maintenance in industries like healthcare, manufacturing, and logistics. Quantum computing is poised to revolutionize data processing capabilities by solving complex problems much faster than traditional computers. Lastly, augmented analytics, powered by AI, is automating data analysis and generating insights, empowering businesses to make data-driven decisions quickly and with greater precision. Together, these innovations are transforming industries and setting the stage for a more connected, efficient, and intelligent future.
Are You Preparing for Data Science Jobs? Check Out ACTE’s Data Science Interview Questions & Answer to Boost Your Preparation!
Conclusion
Data is the backbone of the digital economy, shaping virtually every aspect of modern life. It drives innovation, fuels business growth, and supports critical industry decision-making. Effective data management ensures that data is accurate, accessible, and secure, paving the way for improved decision-making and technological advancements. As the volume of data continues to surge, organizations must prioritize key considerations such as security, privacy, and ethics to fully harness its potential, a central focus in Data Science Training. Protecting sensitive information and complying with privacy regulations are essential to building trust and avoiding risks. Additionally, ethical data practices, including transparency and fairness, are crucial to ensuring that data is used responsibly and in a way that benefits all stakeholders. Organizations can unlock new opportunities, enhance operational efficiency, and stay competitive by managing data effectively in an increasingly data-driven world.