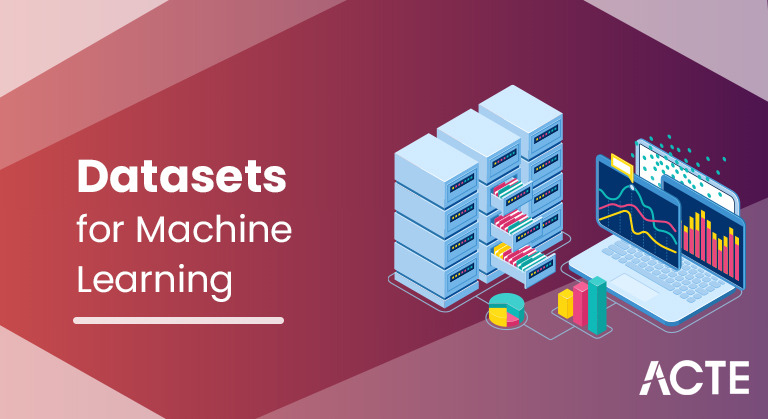
- Machine Learning usually dines like this magical tool, where you shuffle your data and cast the acquired understanding into projections. To do this however you ought to gather, clean, and integrate massive amounts of data.
- We will simplify your vitality today and supply you with an outline of the most useful sites where you can locate aggregated datasets for all purposes. From geographical data to corruption data the possible fields to check are engrossing.
- If you have ever done any data science-related classes or hackathons you presumably came across Kaggle. Kaggle is the world-leading platform for all Data Science associated programming.
- It also permits users to discover and post data sets, and more significant work and contend with other data-science people on how to extract value from them. If you are attempting to learn more about a typical type of problem and want to examine the learning with Data Scientists all about the world Kaggle is the site for you.
- For those of you who like to have a high-level summary Earth Data from Nasa is the right place. It features the presumably largest collection of geo-related datasets about the earth, climate, and water bodies.
- The datasets are delivered and developed by students and institutions around the world and are sure of the most elevated quality known in the individual fields. If you are looking for a project with a focus on time series or geospatial data, this certainly is the best location to start examining.
- The major tech giants feature datasets from all about the world in their open data registries. I made it a joint place because while they do not feature a large variety of datasets, they feature some seriously big datasets.
- Their knowledge in the cloud and big data storage comes in handy when creating such datasets unrestricted to the public. Currently, AWS features about 200 datasets and Azure around 20.
- These sites are the best if you are looking for a project in the Big Data realm and like to work with huge amounts of data.
- If you ever wonder what occurs to those that do not remark on their code well, the FBI crime data explorer power gives you a hint. Likely the biggest data collection around criminal, and noncriminal, law enforcement data. It features data from state-based offenses up to human traffic-related data.
- While this typically is a sad story it is also one of the most compelling types of data. If you are examining for a change and a new exciting project that is a little bit further, it certainly is a gold mine.
- Lionbridge is a business that delivers services about data collection, annotation, and warranty. Among other things, custom labeling conditions and what we are inquisitive in today are a combination of datasets you can find through their website.
- In their dataset section, they show you several pieces including different sources. Such as the ’11 Best Climate Change Datasets for Machine Learning and ‘The 50 Best Free Datasets for Machine Learning. Since they are a business built around datasets their suggestions are surely great.
- Most suitable place if you are examining for a comparison between specialized datasets.
- High quality is an important item to take into concern when you manage a dataset for a machine learning project. But what does this mean in practice? First of all, the data pieces should be appropriate to your goal. If you are developing a machine learning algorithm for an independent vehicle, you will have no appetite even for the best of datasets that consist of star photos.
- Furthermore, it’s essential to provide the details of data that are of good quality. While there are ways of cleansing the data and making it consistent and effortless before annotation and training methods, it’s best to have the data conform to a list of needed elements. For example, when building a facial recognition model, you will require the training photos to be of acceptable enough quality.
- Not only rate but quantity matters, too. It’s necessary to have enough data to train your algorithm correctly. There’s also a chance of overtraining an algorithm (known as overfitting) but it’s more possible you won’t get sufficiently high-quality data.
- There’s no ideal recipe for how much data you require. It’s always a good idea to get guidance from a data scientist. Experts with vast experience usually can roughly calculate the volume of the dataset you’ll require for a specific AI project.
- Alas, it is not acceptable to collect your dataset and make certain it coordinates to all the elements we’ve detailed above. There is one more additional step you ought to take before starting the training of your ML model: a study of the dataset.
- Some topics range from mirthful to horrifying about how greatly an ML algorithm depends on the detailed analysis of its dataset. One of such cases told by Martin Goodson, a guru of data science, shows the story of a hospital that chose to cut medicine costs for pneumonia patients. The positively accurate neural network that was built founded on the clinic data could select the patients with a low risk of creating difficulties. These patients could just take antibiotics at home without the necessity to visit the hospital.
- Managing a dataset for your AI project might seem like an effortless task that can be done in the environment while you pour most of your time and aids in making the machine learning model. However, as training shows, time and time again, marketing with data might take most of your time due to the very scale that this assignment might grow to. For this explanation, it’s important to understand what a dataset in machine learning is, how to address the data, and what features a good dataset has.
- A dataset in machine learning is, rather simply, a collection of data pieces that can be ministered by a computer as a single unit for analytic and prediction objectives. This means that the data gathered should be made livery and comprehensible for a machine that doesn’t see data the exact way humans do. For this, after gathering the data, it’s necessary to preprocess it by washing and finishing it, as well as annotate the data by adding significant tags legible by a computer.
Introduction to Datasets for Machine Learning:
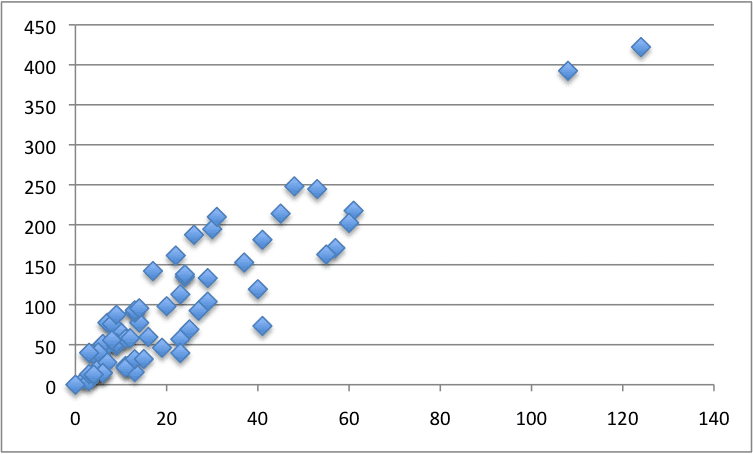
Google’s Datasets Search Engine:
As with Google’s core product, you can effortlessly search for the datasets utilizing text. Further, you can filter the query by date, data format, and usage privileges. The datasets on this website are content from real-life datasets supplied by businesses for a price to free to use datasets for individual projects. If you are examining for a wonderful overview of all datasets unrestricted without any clear rules google is the best place to start.
Kaggle Datasets:
Earth Data:
Amazon and Microsoft Datasets, Azure and AWS
FBI Crime Data Explorer
Data World
A group that is rarely noted is the Data world. It’s remarkably comparable to the Google dataset search engine. What I however find very friendly about this performance is the search depth, when joining a query it does not only show the dataset itself but also subfiles that power includes the desired data. This can of course be extremely helpful when examining secondary data such as demographics and geographic location collections. If you are examining for a dedicated website that has data in its name, Data World comes highly suggested.
CERN Open Data Portal
The European Organization for Nuclear Research(CERN) discovered close to Geneva has made many of their excellent research data unrestricted to the public. CERN’s Open Data portal is fascinating. They organized and made public over two petabytes of data on the most diminutive thing possible, particle physics. This is one of Europe’s most prestigious examination institutions, and their data quality on particle collisions can’t be met by anyone.
Lionbridge AI Datasets:
UCI Machine Learning Repository:
The University of California, Irvine holds over 550 datasets which are free for you to use. I discover this website to be extremely attractive for educational pursuits since it offers filtering by the situation. So classification, regression, and clustering, you can readily find a dataset that would work well with the technologies that you are presently studying. Apart from learning how to educate individuals their team certainly knows a lot about Machine Learning datasets and how to consider them.
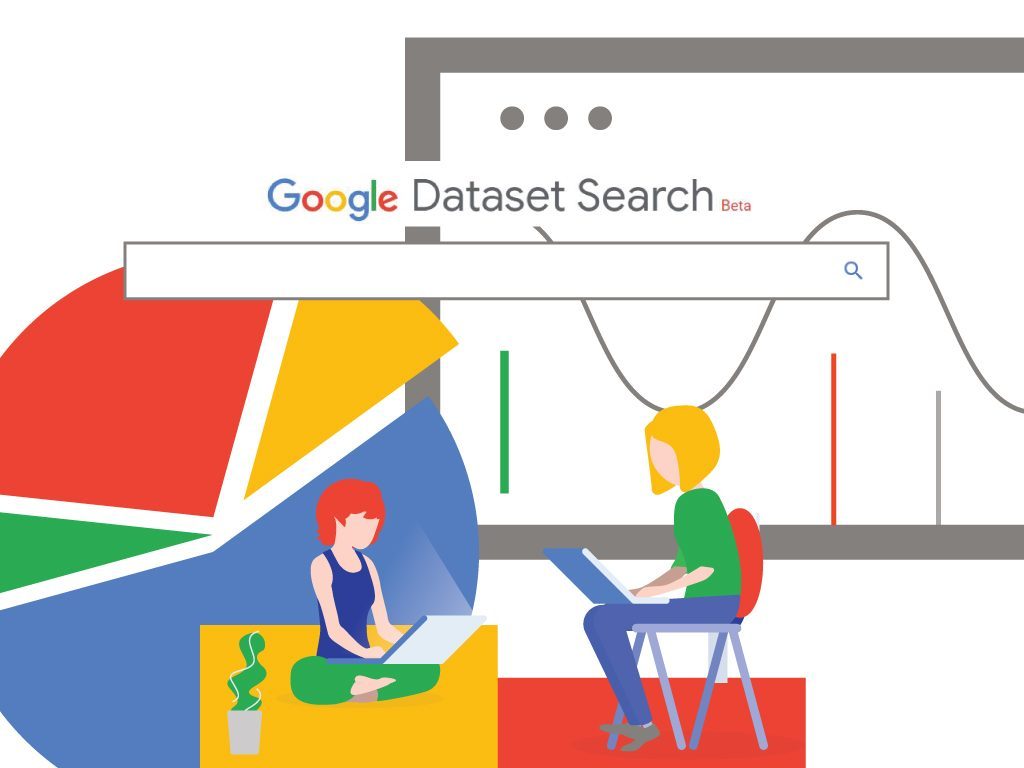
Components of the Data: How to Create Yourself a Good Dataset for a Machine Learning Project?
Basic data is a good place to begin but you apparently cannot just shove it into a machine learning algorithm and expect it offers you invaluable insights into your customers’ behaviors. There are quite a few actions you ought to take before your dataset becomes functional.
Collect: The first item to do when you’re examining a dataset is decide on the seeds you’ll be utilizing to manage the data. Usually, there are three types of seeds you can select from: the freely available open-source datasets, the Internet, and the generators of artificial data.
Preprocess: There’s a guide in data science that every professional experienced adheres to. Start by replying to this question: has the dataset you’re operating been used before? If not, consider this dataset is bad.
Annotate: After you’ve provided your data is pure and appropriate, you also ought to make sure it’s legible for a computer to process. Machines do not comprehend the data the exact way humans do (they aren’t able to give the same importance to the images or words as we).
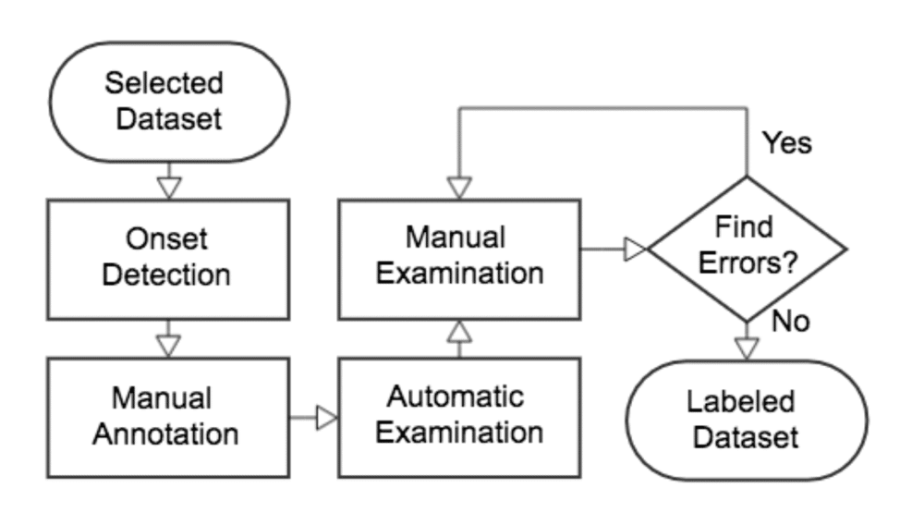
The Features of a Proper, High-Quality Dataset in Machine Learning:
Quality of a Dataset: Relevance and Coverage
Acceptable Amount of a Dataset in Machine Learning:
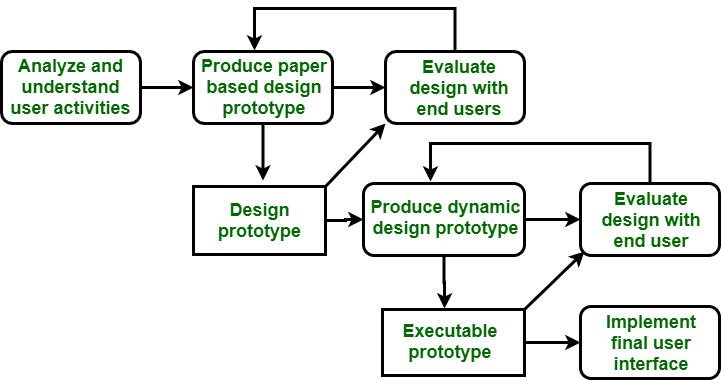
Before Deploying, Analyze Your Dataset:
Datasets for General Machine Learning:
In this context, “general” is directed to as Relapse, Classification, and Clustering with relational data:
Wine Quality – Effects of red and white Vinho Verde wine selections from the north of Portugal. The purpose here is to sport wine quality established on some physicochemical tests.
Credit Card Default – Forecasting credit card default is a practical use for machine learning. This dataset contains payment history, demographics, credit, and default data.
US Census Data – Clustering based on demographics is a tested and tested way to conduct market analysis as well as segmentation.
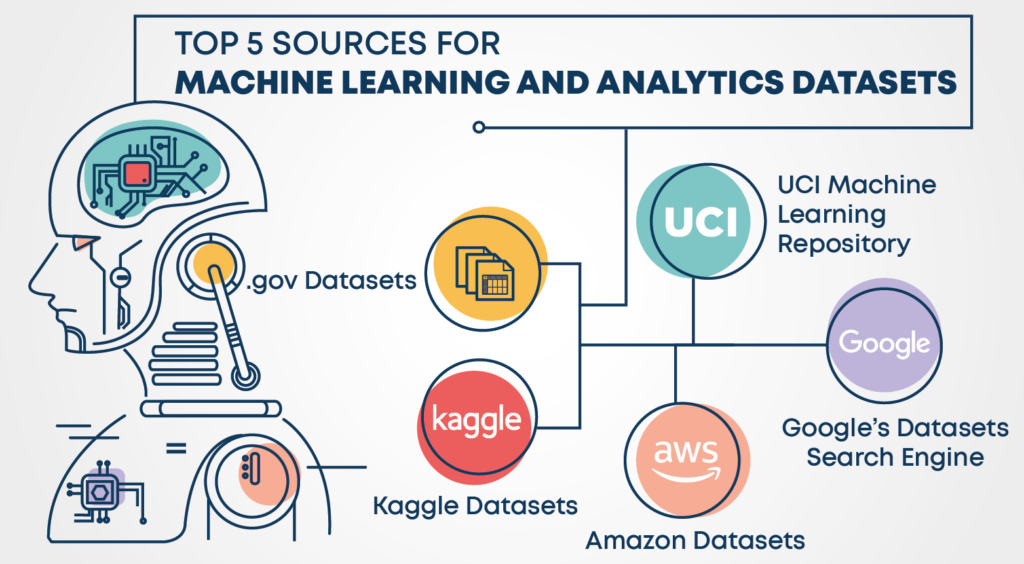
In Conclusion: What You Must to Know Regarding Datasets in Machine Learning: