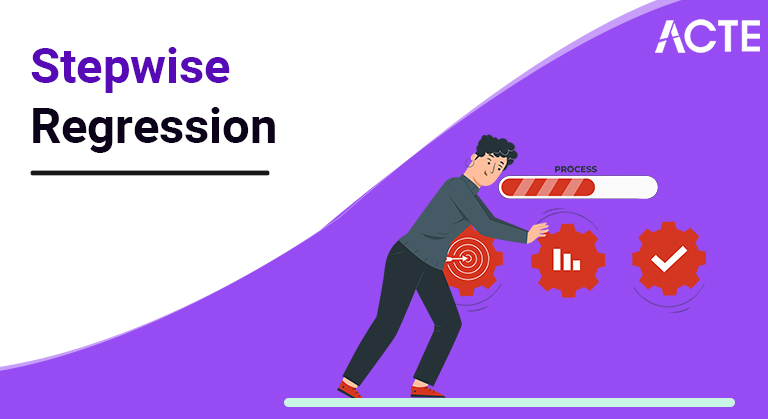
The ability to manage large amounts of potential predictor variables, fine-tuning the model to choose the best predictor variables from the available options. It’s faster than other automatic model-selection methods.
- What is Stepwise Regression?
- Types of Stepwise Regression
- Example
- Stepwise regression analysi
- How Stepwise Regression Works
- Advantages of stepwise regression include
- Why? regression
- Alternatives
- Criticism
- Conclusion
What is Stepwise Regression?
Step-by-step reconstruction is a step-by-step reconstruction of the retrospective model that involves the selection of independent variables to be used in the final model. It involves adding or subtracting dynamic annotations that may occur in sequence with a statistical value test after each multiplication. The availability of statistical software packages makes slower deprecation possible, even for models with hundreds of variables.
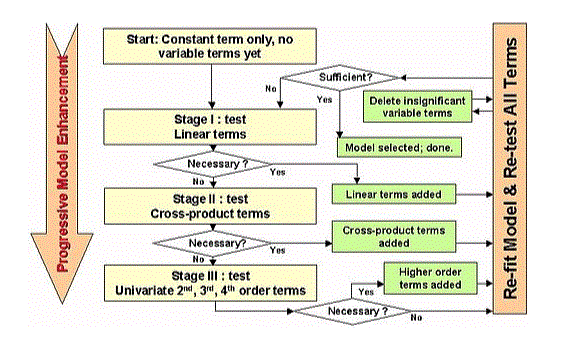
- Advanced choice starts with no flexibility within the model, examines every variable because it is another to the model, and so keeps those that are thought of statistically important — repetition the method till the results are prepared.
- Backlinking starts with a group of freelance variations, removes one at a time, and so checks to check if the output variation is statistically important.
- The 2-dimensional end may be a combination of the primary two ways that check that variables ought to be another or removed.
Types of Stepwise Regression:
The basic goal of stepwise retrieval, through a series of tests (e.g. F-tests, t-tests) is to get a group of freelance variables that have the foremost impact on dependent variables. This is often done by computerised repetition, that may be a method of achieving results or conclusions through perennial cycles or analysis cycles. Doing machine-driven tests with the assistance of mathematical software package packages has the advantage of saving time and limiting errors.
Stepwise retrospective is achieved by experimenting with one individual freelancer of your time and incorporating it into a retrospective model if it’s mathematically important or by incorporating all potential freelance variables within the model and eliminating those who aren’t statistically important. Some use a mix of each ways thus there are 3 ways to abate gradually:
Example:
An example of a gradual reversal of the regression method would be an attempt to understand industry energy consumption using variables such as operating time, machine age, labour size, external temperatures, and time of year. The model covers all the variables — and then each one is subtracted, one at a time, to determine which is mathematically important. Finally, the model may indicate that the time of year and the temperatures are the most important, which may suggest that the highest energy consumption in the industry is when the climate use is the highest.
Stepwise regression analysis:
Depression analysis, both direct and multivariate, is widely used in the world of economics and investment today. The idea is to discover patterns that existed in the past that may recur in the future. A simple line downturn, for example, may look at price and profit rates and stock returns over a period of years to determine whether stocks with lower P / E rates (independent variables) offer higher returns (dependable volatility). The problem with this approach is that market conditions are often changing and relationships that have existed in the past do not really exist in the present or in the future.
At the same time, the process of gradual reversal has many critics and there are even calls to stop using the method completely. Mathematicians point out a number of obstacles along the way, including adverse outcomes, inherent bias in the process itself, and the need for critical computer power to develop complex retrieval models over and over again.
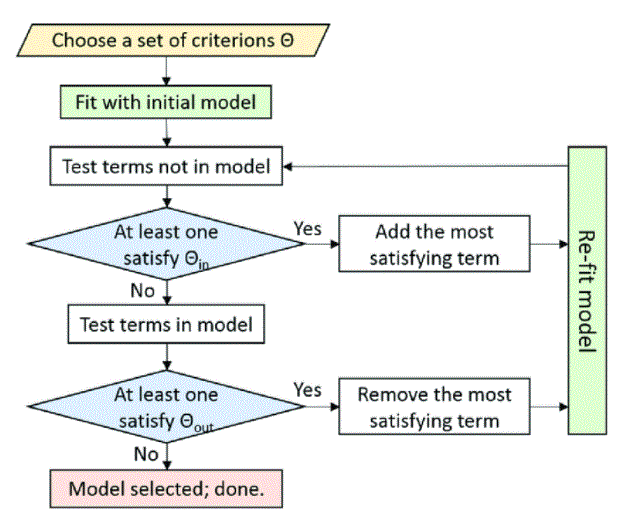
- Start experimenting with all out there predictors (the technique “Back :), delete one variable at a time because the retrospective model progresses.
- Use this technique if you’ve got a modest quantity of statement fluid and need to eliminate some.
- In every step, the variant with the lower “F-to-remove” values is faraway from the model. The “F-to-remove” statistics area unit as follows:
- The t values are calculated at the calculable constant of every variance within the model.
- T-statistic sq., creates “F-to-remove” figures.
- Start testing while not prophetical flexibility (“Forward” method), and add one at a time because the retrospective model progresses.
- If you’ve got an outsized set of prophetical variables, use this technique.
- The “F-to-add” statistics were created victimisation constant steps higher than, except that the system would calculate statistics for every non-model variance.
- Variables with terribly high “F-to-add” numbers are other to the model.
How Stepwise Regression Works:
Two ways that software package will try this is by step-by-step:
- Stepwise retrieval usually has many variables that may be present but very little data to measure coefficients in a meaningful way. Adding additional data is not very helpful, in that case.
- If two predictive variables in a model are closely related, only one can be modelled.
- R square values are usually very high.
- Adjusted r-squared values may be higher, and then immerse more as the model progresses. If this happens, find the variable added or removed when this happens and adjust the model.
- The F and chi-square tests calculated next to the output variant do not have that distribution.
- Estimated prices and confidence intervals are very small.
- P values are given without proper meaning.
- The recovery coefficients are biassed and the coefficients in some variables are very high.
- Collinearity is often a major problem. Excess collinearity may cause the system to lose the predictive variable in the model.
- Other variables (especially dummy variables) may be excluded from the model, where it is considered essential to be included. These can also be installed manually.
Advantages of stepwise regression include:
Ability to manage large amounts of potential variables, fine-tuning the model to select the best predictable variables of the available options. Faster than other default model selection methods. Observing the system for variable output or additions can provide important information about the quality of predictive variables. Although step-by-step recipes are popular, many mathematicians (see here and there) agree that they are full of problems and should not be used. Other problems include:
Why? regression:
This paper identifies specific problems by gradually retreating, notes criticism of mathematical methods by experts, suggests appropriate ways in which procedures can be used, and provides examples of how this can be done. Although the method of wise action has always been criticised by mathematicians, it is still widely used in literature. This paper proposes research scenarios where step-by-step retrieval may have an important function. Stepwise methods may be suitable for flexible testing. As the value of variability as a predictor is largely determined by other variables in the predictive model, the use of step-by-step methods can provide many reduced models where variability factors can be assessed.
As variables are found as positive predictions in different models, different variable predictive features in different models can be used to determine how variables work as predictions and can be used to develop theories or models that can be further evaluated. research. For smart methods to be used effectively, they must be used in accordance with the best sub-standard procedures and zero order correlations, standard set values must be changed, models must not be computer-selected, and, where possible, models must be modified. is produced from multiple subsets of data. (Contains 21 tables and 17 references.) (SLD)
Alternatives:
A widely used algorithmic program was 1st planned by Efroymson (1960). [10] this can be an automatic method of choosing a mathematical model in things wherever there’s an outsized variety of variables which will occur, and there’s no basic theory on which the model choice relies. The procedure is employed primarily for descent analysis, though the essential approach applies to several kinds of model choice. This can be completely different from forward selection. At every stage of the method, once a brand new add-on is added , a take a look at is performed to ascertain if a number of the variables is removed while not increasing the positive volume of the remaining squares (RSS). the method is terminated once the live is created (locally) to an outsized extent, or once the on the market improvement falls below a major price.
One of the biggest issues with swiftness is that it searches an outsized space for attainable models. it’s thus susceptible to overloading information. In alternative words, bit-by-bit retrieval can typically be far better suited to the sample than getting into new information outside the sample. Severe cases are known once models have gained the worth of operational statistics by random numbers. [11] This drawback is decreased if the condition for adding (or removing) a special one is powerful enough. vital|a crucial|a vital|A very important} line within the sand is what is thought of as a Bonferroni point:
That however important a positive false positive ought to be supported by luck alone. On the mathematical scale t, this happens around }} \ sqrt , wherever p is the variety of predictors. Sadly, this suggests that the majority variables holding signals won’t be enclosed. This phone becomes a good trade between the foremost complete and therefore the missing signals. If we tend to take into account the chance of outstanding termination, then applying this obligation is going to be at intervals the two \ log p} two \ log p} features of the simplest risk issue. from now on reductions can find yourself with a better risk of inflation.
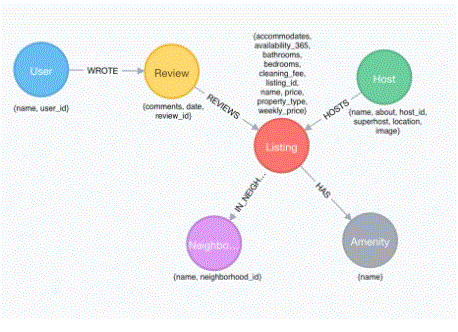
- Stepwise retrieval procedures are a unit employed in data processing, however they’re moot. many points of criticism are raised.
- The take a look at itself is biassed because it is predicated on a similar knowledge. Sir Geoffrey Wilkinson and Dallal (1981) calculated the share constant of most constant of imitation and showed that the last retrospective regression obtained, meaning by the F method being the foremost necessary at zero.1%, was really solely necessary at five-hitter.
- When estimating the degree of freedom, the experimental variable price from the simplest equation elite is also smaller than the entire variety of ultimate variable models, which causes the equation to seem higher than it’s once adjusting the r2 price of the degree price. of freedom. it’s necessary to think about what percentage degrees of freedom are applied to all or any models, not simply to calculate the number of freelance variables within the ensuing equity.
- Created models may be abundant and less complicated for real knowledge models.
- Such criticisms supported the restrictions of the link between the model and therefore the method and therefore the knowledge set accustomed to live it, an area typically handled by confirmatory the model in AN freelance knowledge set, as within the PRESS method.
Criticism:
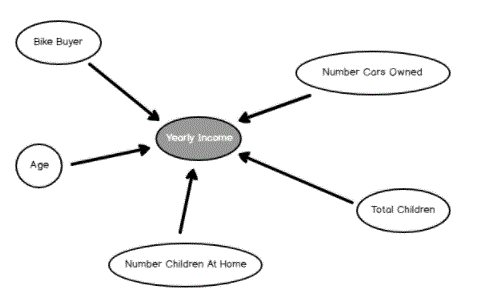
- The gradual retrospective is a method of inserting retrospective models in which predictable variable selections are performed by default. In each step, a variant is considered to add or subtract from a set of descriptive variables based on a specific subject. Typically, this takes the form of forwarding, backward, or integrated sequences of F-tests or t-tests.
- The common practice of inserting the last selected model followed by reporting measurements and intervals without the confidence to consider the model-building process has led to a call for a complete suspension of the modelling model or at least a guarantee. The model of uncertainty is well documented.
- This example from engineering, demand, and adequacy is usually determined by the F test. For further consideration, when planning a test, computer simulation, or scientific survey to collect data for this model, one must remember the number of parameters, P, in order to estimate and adjust the sample size accordingly. In the variable K, P = 1 (Start) + K (Phase I) + (K2 – K) / 2 (Phase II) + 3K (Phase III) = 0.5K2 + 3.5K + 1. In K < 17, efficiency The test design exists for this type of model, the Box-Behnken design, [9] extended by vertical or incorrect axial points minute (2, (int (1.5 + K / 4)) 1/2), and and point (s) at the beginning. There are some very efficient designs, which require a few runs, even in K> 16.The main ways to slow down are:
- Advance selection, which includes starting without flexibility in the model, checking the addition of each variable using the equation determination method of the selected model, adding flexibility (if any) to your input provides the most significant mathematical improvement, and repeats this process until there is no statistically significant improvement.
- Back-to-back, which includes starting with all types of candidates, checking the removal of each variant using the selected model equity indicator, derecognition (if any) your loss provides a statistically significant decrease in model equity, and repeats this process until it is no more. alternatives can be removed without loss of mathematical equity.
- Completion of two-dimensional, a combination of the above, testing in each step to insert a different or extracted.
Conclusion: