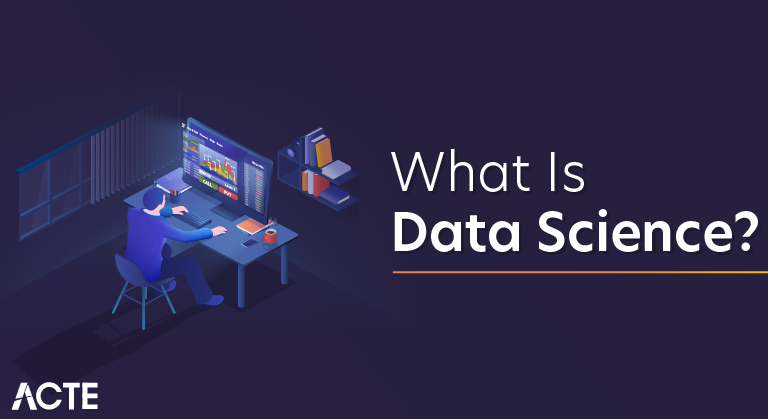
As the world entered the era of big data, the need for its storage also grew. It was the main challenge and concern for the enterprise industries until 2010. The main focus was on building framework and solutions to store data. Now when Hadoop and other frameworks have successfully solved the problem of storage, the focus has shifted to the processing of this data. Data Science is the secret sauce here. All the ideas which you see in Hollywood sci-fi movies can actually turn into reality by Data Science. Data Science is the future of Artificial Intelligence. Therefore, it is very important to understand what is Data Science and how it can add value to your business.
What is Data Science?
- Use of the term Data Science is increasingly common, but what does it exactly mean? What skills do you need to become a Data Scientist? What is the difference between BI and Data Science? How are decisions and predictions made in Data Science? These are some of the questions that will be answered further.
- First, let’s see what is Data Science. Data Science is a blend of various tools, algorithms, and machine learning principles with the goal to discover hidden patterns from the raw data. How is this different from what statisticians have been doing for years?
The answer lies in the difference between explaining and predicting.
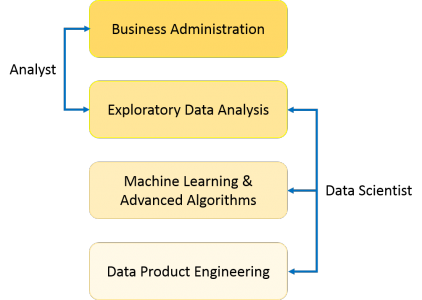
- As you can see from the above image, a Data Analyst usually explains what is going on by processing the history of the data. On the other hand, Data Scientist not only does exploratory analysis to discover insights from it, but also uses various advanced machine learning algorithms to identify the occurrence of a particular event in the future. A Data Scientist will look at the data from many angles, sometimes angles not known earlier.
- So, Data Science is primarily used to make decisions and predictions making use of predictive causal analytics, prescriptive analytics (predictive plus decision science) and machine learning.
- Predictive causal analytics – If you want a model which can predict the possibilities of a particular event in the future, you need to apply predictive causal analytics. Say, if you are providing money on credit, then the probability of customers making future credit payments on time is a matter of concern for you. Here, you can build a model which can perform predictive analytics on the payment history of the customer to predict if the future payments will be on time or not.
- Prescriptive analytics: If you want a model which has the intelligence of taking its own decisions and the ability to modify it with dynamic parameters, you certainly need prescriptive analytics for it. This relatively new field is all about providing advice. In other terms, it not only predicts but suggests a range of prescribed actions and associated outcomes.
The best example for this is Google’s self-driving car which I had discussed earlier too. The data gathered by vehicles can be used to train self-driving cars. You can run algorithms on this data to bring intelligence to it. This will enable your car to take decisions like when to turn, which path to take, when to slow down or speed up. - Machine learning for making predictions — If you have transactional data of a finance company and need to build a model to determine the future trend, then machine learning algorithms are the best bet. This falls under the paradigm of supervised learning. It is called supervised because you already have the data based on which you can train your machines. For example, a fraud detection model can be trained using a historical record of fraudulent purchases.
- Machine learning for pattern discovery — If you don’t have the parameters based on which you can make predictions, then you need to find out the hidden patterns within the dataset to be able to make meaningful predictions. This is nothing but the unsupervised model as you don’t have any predefined labels for grouping. The most common algorithm used for pattern discovery is Clustering.
Let’s say you are working in a telephone company and you need to establish a network by putting towers in a region. Then, you can use the clustering technique to find those tower locations which will ensure that all the users receive optimum signal strength.
Let’s see how the proportion of above-described approaches differ for Data Analysis as well as Data Science. As you can see in the image below, Data Analysis includes descriptive analytics and prediction to a certain extent. On the other hand, Data Science is more about Predictive Causal Analytics and Machine Learning.
Business Intelligence (BI) vs. Data Science
- BI basically analyzes the previous data to find hindsight and insight to describe the business trends. BI enables you to take data from external and internal sources, prepare it, run queries on it and create dashboards to answer the questions like quarterly revenue analysis or business problems. BI can evaluate the impact of certain events in the near future.
- Data Science is a more forward-looking approach, an exploratory way with the focus on analyzing the past or current data and predicting the future outcomes with the aim of making informed decisions. It answers the open-ended questions as to “what” and “how” events occur.
This was all about what is Data Science, now let’s understand the lifecycle of Data Science.
A common mistake made in Data Science projects is rushing into data collection and analysis, without understanding the requirements or even framing the business problem properly. Therefore, it is very important for you to follow all the phases throughout the lifecycle of Data Science to ensure the smooth functioning of the project.
Lifecycle of Data Science
Here is a brief overview of the main phases of the Data Science Lifecycle:
- Phase 1—Discovery: Before you begin the project, it is important to understand the various specifications, requirements, priorities and required budget. You must possess the ability to ask the right questions. Here, you assess if you have the required resources present in terms of people, technology, time and data to support the project. In this phase, you also need to frame the business problem and formulate initial hypotheses (IH) to test.
- You can use R for data cleaning, transformation, and visualization. This will help you to spot the outliers and establish a relationship between the variables. Once you have cleaned and prepared the data, it’s time to do exploratory analytics on it. Let’s see how you can achieve that.
- Phase 3—Model planning: Here, you will determine the methods and techniques to draw the relationships between variables. These relationships will set the base for the algorithms which you will implement in the next phase. You will apply Exploratory Data Analytics (EDA) using various statistical formulas and visualization tools.
Let’s have a look at various model planning tools.
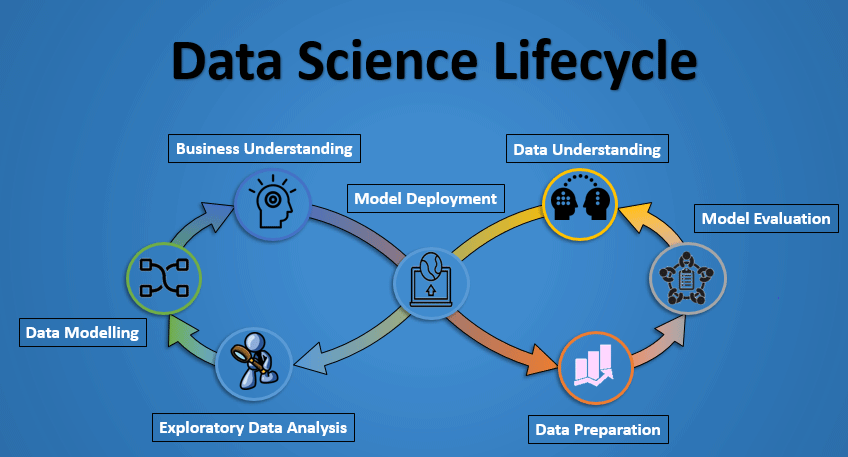
- R has a complete set of modeling capabilities and provides a good environment for building interpretive models.
- SQL Analysis services can perform in-database analytics using common data mining functions and basic predictive models.
- SAS/ACCESS can be used to access data from Hadoop and is used for creating repeatable and reusable model flow diagrams.
Although many tools are present in the market, R is the most commonly used tool.
- Now that you have got insights into the nature of your data and have decided the algorithms to be used. In the next stage, you will apply the algorithm and build up a model.
- Phase 4—Model building: In this phase, you will develop datasets for training and testing purposes. You will consider whether your existing tools will suffice for running the models or it will need a more robust environment (like fast and parallel processing). You will analyze various learning techniques like classification, association and clustering to build the model.
- Phase 5—Operationalize: In this phase, you deliver final reports, briefings, code and technical documents. In addition, sometimes a pilot project is also implemented in a real-time production environment. This will provide you a clear picture of the performance and other related constraints on a small scale before full deployment.
- Phase 6—Communicate results: Now it is important to evaluate if you have been able to achieve your goal that you had planned in the first phase. So, in the last phase, you identify all the key findings, communicate to the stakeholders and determine if the results of the project are a success or a failure based on the criteria developed in Phase 1.