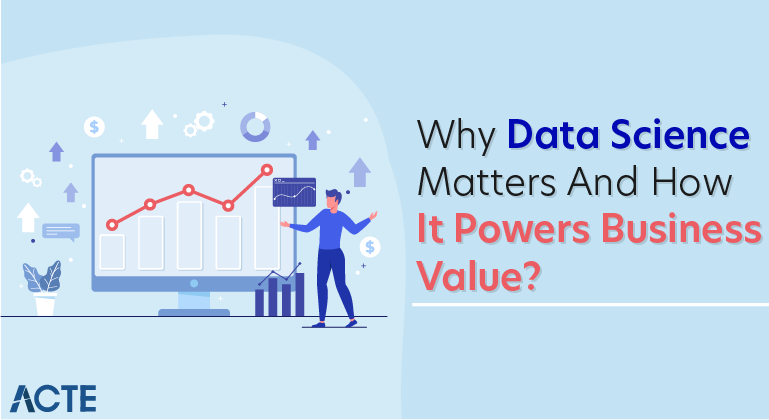
- There are massive opportunities here. The automation of extremely tough data science tasks such as preparing the data, and the empowering the analysts without coding experience to construct models, this helps in keeping business agile and innovative. Creating and automation for the data science life-cycle makes the experts find free time to address more interesting and innovative areas of the field. When there is a mixture of human intelligence along with technology and automation, this combination helps businesses extract greater value from data.
- Artificial Intelligence will be automating more than 50% of the data scientist activities by 2025 which will in turn ease the acute talent shortage. IBM provides Auto AI to automate data science and AI life-cycle management. Most of the time of the data scientist’s working time is spent in finding, cleaning and organizing data which amounts to about 80% of their time. This job is also one of the most promising job profiles in 2019 and 2020 and for the years to come in the future.
- The magnitude and diversity of social, mobile and device data, including new technologies and tools, data science today plays a broader role than ever before. Businesses consider data analytics and data science along with AI to be a technology-enabled strategy. For data science to be effective, its full life-cycle should support traditional analytics and also must work in concert with latest and modern applications. This implies that the data science practice must transform beyond routine, tedious tasks — as much 80% of a data scientist’s time is spent cleaning, shaping and moving data from place to place, often to feed machine learning. This leaves only a small percentage of their time to find patterns and trends, to build complex models, to predict and forecast, and to interpret results.
- Fortunately, there is relief. The latest development in modern data science is an Auto AI capability that automates the data preparation and modeling stages of the data science life-cycle. Now, not only can more data scientists use their specialized skills the way they were intended; but more businesses can benefit from data science, from prediction to optimization.
- Data Science has become a revolutionary technology that everyone seems to talk about. Hailed as the ‘sexiest job of the 21st century’, Data Science is a buzzword with very few people knowing about the technology in its true sense. While many people wish to become Data Scientists, it is essential to weigh the pros and cons of data science and give out a real picture. In this article, we will discuss these points in detail and provide you with the necessary insights about Data Science.
WHY DOES DATA SCIENCE MATTER?
Starting a tech company, building a good product, and gaining traction have become easier thanks to improved connectivity, declining cloud storage and computing costs, and the accessibility of distribution platforms for reaching target audiences. As a result, the time it takes a product to reach 100 million monthly active users has shortened dramatically, and continues to shrink today. For example, it took about 100 months for iTunes to reach 100 million monthly active users in 2003 and mere days for Pokemon Go to do the same. The chart below has some more good examples, starting with the telephone.
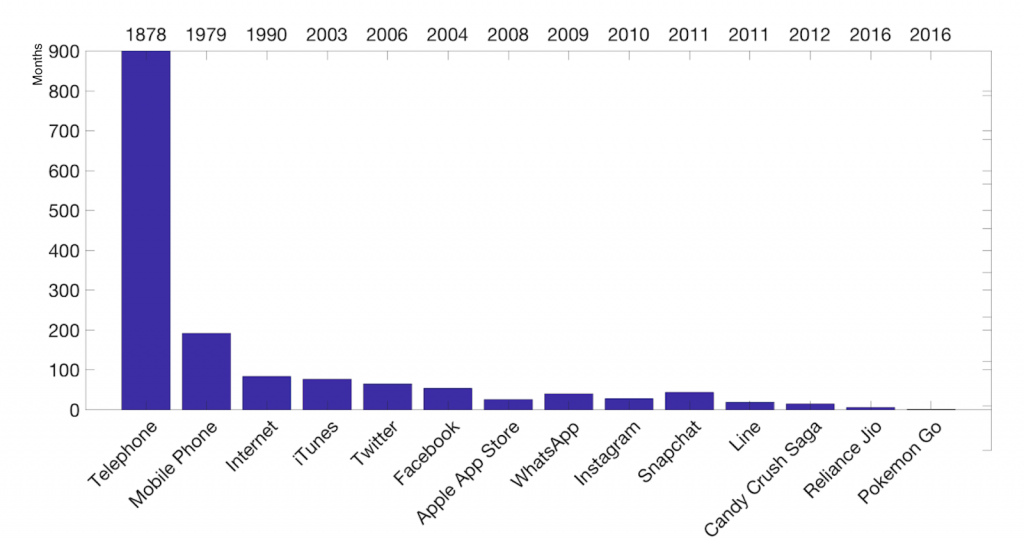
The combination of more products built, more Internet-connected devices bought, and increased time spent online has caused a spike in the volume of user interaction data. There has been a tremendous interest in mining this data and deriving key insights to help build great products. A company’s ability to compete is now measured by how successfully it applies analytics to vast, unstructured data sets across disparate sources to drive product innovation. Therefore, data scientists are in high demand, and that a team of smart data scientists can make or break a product.
This increasing interest in mining data for insights has led product teams to use data to focus on four specific outcomes.
- Evaluate the health of the business: One of the key outcomes of product analysis is to evaluate the health of a product or a business. Once we have defined product success by the means of a goal and a metric, the next step is to monitor the metric to ensure that we are on track to hitting the goal. Tactically, analysts work on identifying outliers, understanding the drivers of changes in metrics, building dashboards / reports / visualizations etc.
- Ship the right products and features: Another very important role of analytics is to ensure that the right products and features get built. Many companies run numerous experiments and ship products after evaluating the results of these experiments. Typically data scientists help design experiments, identify data-informed hypotheses on phenomena, and guide product team on constant optimization of the product through the data insights.
- Forecast outcomes and Power production systems — Another role of data scientists is to build prototypes/models and power production systems using AI/ML. These data scientists train machine learning models of a phenomenon in order to forecast future expectations and trends.
- Set roadmap and strategy for the product: Deeper exploration and analysis of the user journey and phenomena generate actionable insights that ultimately result in setting roadmap and strategy for the product. Data-driven roadmap and strategy is one of the most important outputs of a world class product analytics team.
EVOLUTION OF DATA SCIENCE
Imagine a world in which a machine knows everything about you and can shop for you without even explicitly asking for it; knows the food you like and cooks for you; knows your choices and can make decisions for you and knows what is good for you and plans your life. This world is distant into the future and requires Artificial Intelligence to take over much of our lives. For us to make progress toward this dream, we need to become even more data-driven.
In a perfect world with perfect information and a complete understanding of all the drivers of your system and how they interact with each other, the two approaches would converge. In order to build a perfect model, the phenomenon under study needs to be completely understood; the relationship between the data and the phenomenon can be described by a perfect model (and associated rich feature set). In order to evolve to this level of perfection and also make progress in the interim, the world will need to continue to make progress on data-informed decision-making. i.e., we need to continue augmenting our decision making by other subjective measures that cannot easily be fully quantified yet. As we begin to have a deeper understanding of relationships between objects, more and more processes will get automated away and the future will be more data-driven than data-informed. However, data-informed decision making will continue to be extremely important for the next few decades and data-driven decision making will only improve with advancements from people who are data-informed.
It is most illustrative to understand the differences between data-informed decision making and data-driven decision making by means of examples.
- Setting goals. Good goals are measurable and quantifiable. Being able to identify and track goals will become increasingly data-driven. For example, Facebook’s tracking of its active users maybe completely automated. However, setting the right quarterly and annual goals for active users and revenue maybe only partly automated and will continue to be data-informed.
- Defining a roadmap and strategy. Establishing a roadmap and strategy is not quantitative and hence requires data-informed approaches. For example, by using data, a roadmap can be developed for increasing daily active usage by focusing on SMS notification. A good roadmap considers the relevant goals, the drivers of these goals, the levers that the product team has, and all of the courses of action that can be taken. Much of this is qualitative, so the process of building a roadmap and defining strategy is primarily data-informed.
- Forecasting outcomes. Forecasting outcomes is mostly data-driven. For example, figuring out whether or not to show a story to a user would require understanding multiple factors, including the probability of a user clicking or reading that story. Companies typically develop models, which are iterated on continuously, to forecast this specific outcome.
- Powering production systems. For companies like PayPal to identify fraudulent activity of any transaction, it is prohibitively expensive to do this manually for all transactions. As a result, they largely rely on machine learning to power their production systems and automate the calculation of the probability of a transaction being bad. Much of the decision-making that follows the evaluation of the probability is also automated. However, in areas where there are lower levels of confidence in the probability evaluations, the decision process could be data-informed.
How does Data Science Matter?
- Scientist of natural sciences collects data from experiments and observations that may affect the natural order of the cosmos. Likewise, researchers of behavioral and social sciences administer surveys and interviews to gather information about changes in human organizations, group behaviors, phenomena, customs, and traditions that may affect human civilization.
- In line with this, it appears that both natural and social sciences utilize data in order to advance in developing theories, improving the practices and producing innovations related in their fields of study. In other words, no field of study or human organization that does not need to use any data. As basic as this fact is, data science really matter because data gathered must make sense.
- It must be used to answer questions, make decisions and solve problems. Hence, no matter how big or small the chunk of gathered data, if it’s not extracted with insights it is useless at all.
How does Data Science Powers Up Business Value?
- Inasmuch as it is important for both natural and social sciences to apply data science, business development and entrepreneurship also needs this. Business organizations and companies need to collect data in the following forms and purposes. First, business owners and entrepreneurs must be able to identify their target market by determining the demand-supply gap that exists in the location where they intend to build a new business.
- This applies, most especially, to start-up companies. Furthermore, the demand-supply gap analysis is essential to them especially in planning to introduce new products or services.
- Secondly, once a company has begun operating, it needs a system that would facilitate transactions such as the placing of orders, payment acceptance, salary payment for employees and other transactions that keeps the fluidity of the business operations. Thus, the emergence of blockchain technology may be helpful in this particular area of the business. Thirdly, the volume of these transactions may also serve as data to evaluate whether your business is growing or not.
- The bullish or bearish performance in the number of sales, profits, and income of the employees are just some of the indicators. Finally, companies utilize vital data in order to project their stability in the long run and to determine what else is needed to innovate to keep up with the fast changing trends of the global market.
- In conclusion, data science really powers up business value. In fact, most of these big companies, if not all, spend millions of dollars just to ensure that everything is going smoothly. Because of this scientific process, it enables them to gain insights from collected raw data which can be used to improve and innovate various aspects of their businesses.