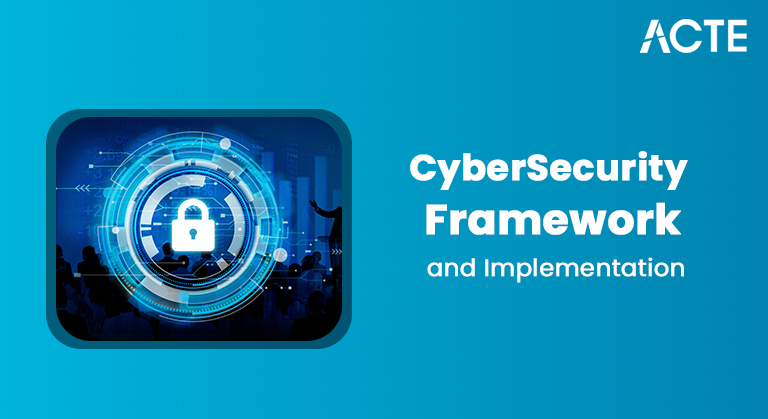
- Introduction
- Open-source AI Models (LLaMA, Mistral, etc.)
- Commercial AI Chatbots (Claude, Gemini, Copilot)
- GPT-based vs. Transformer-based Models
- AI Models for Different Use Cases
- Cost and Subscription Plans Comparison
- Performance Benchmarks
- Customization and Fine-tuning Options
- Offline AI Chatbot Solutions
- Conclusion
Introduction
As conversational AI becomes an integral part of how we work, learn, and interact with technology, the demand for capable and customizable AI chat assistants is growing rapidly. While ChatGPT has set a high bar for performance and usability, it’s far from the only option. A wide range of alternatives, ranging from open-source models like LLaMA and Mistral to commercial offerings such as Claude, Gemini, and Copilot, provide diverse capabilities tailored to different use cases, including Data Science Training. Whether you’re a developer seeking full control through open-source solutions, or a business looking for enterprise grade performance and integration, understanding the AI chatbot landscape is essential. This guide explores the leading ChatGPT alternatives across several dimensions, including architecture, cost, customization, and future potential helping you make informed decisions based on your specific goals and constraints.
Eager to Acquire Your Data Science Certification? View The Data Science Course Offered By ACTE Right Now!
Open-source AI Models (LLaMA, Mistral, etc.)
Open-source AI models are freely available for public use, allowing developers to modify and deploy them in various applications. LLaMA (Large Language Model Meta AI) by Meta and Mistral are popular open-source models for text generation and comprehension tasks. These models offer flexibility, enabling organizations to customize and fine-tune them based on their needs. Leveraging Machine Learning Techniques, they continuously improve performance by learning from data and adapting to specific tasks. Open-source AI promotes transparency, collaboration, and innovation by allowing the community to contribute to improvements. Additionally, they are ideal for companies seeking cost-effective and customizable AI solutions without vendor lock-in.
Commercial AI Chatbots (Claude, Gemini, Copilot)
Commercial AI chatbots, such as Claude (Anthropic), Gemini (Google), and Copilot (Microsoft), are proprietary models designed for high-performance conversational AI.
- Claude: Known for its safety and ethical considerations, offering reliable outputs for enterprise applications.
- Gemini: Offers multimodal capabilities (text, image, audio) with Google ecosystem integration.
- Copilot: Integrated with Microsoft 365, enhancing productivity with document and workflow automation. These chatbots provide enterprise-grade support, security, and regular updates, making them suitable for business and commercial use cases. Powered by advanced Artificial Intelligence algorithms, they can understand complex queries, deliver contextual responses, and automate a wide range of customer interactions.
- GPT-based models (e.g., GPT-4, GPT-3.5) are designed for natural language generation and excel in creative content writing, conversation, and text-based applications.
- Transformer-based models: Include a broader range, such as BERT, T5, and Roberta, optimized for text classification, sentiment analysis, and language understanding.
- GPT models focus on generative capabilities, while transformer models are widely used for language comprehension and processing tasks.
- Text Generation: GPT-4, Claude – for content creation, summarization, and conversation.
- Multimodal AI: Gemini – processing text, image, and audio inputs.
- Customer Support: Copilot and custom GPT deployments for chatbots and virtual assistants.
- Data Analysis: AI models like Code Interpreter assist in data processing and analysis.
- Creative Content: AI tools for image, music, and video generation (e.g., DALL·E, Stable Diffusion).
- Choosing the right model depends on specific use cases and performance needs. Production System in AI, factors like scalability, latency, and integration with existing infrastructure also play a critical role in model selection.
- AI chatbots and models vary significantly in pricing and subscription plans.
- Open-source models: Free or low-cost, with infrastructure expenses for deployment.
- Commercial models: Subscription plans with tiered pricing.
- ChatGPT Plus: $20/month for GPT-4 access.
- Claude Pro: $20/month for faster performance.
- Copilot for M365: Included in Microsoft 365 plans, with business-tier pricing.
- Commercial models offer better performance, reliability, and support, while open-source models are more cost-effective for tech-savvy users.
- Open-source models: Easily fine-tuned with custom datasets, offering flexibility.
- Commercial models: Provide API-level customization and advanced fine-tuning (e.g., GPTs in ChatGPT).
- Low-code tools: Platforms like Hugging Face allow developers to fine-tune models with minimal coding.
- Customization ensures that AI outputs align with specific business goals, enhancing productivity and accuracy.
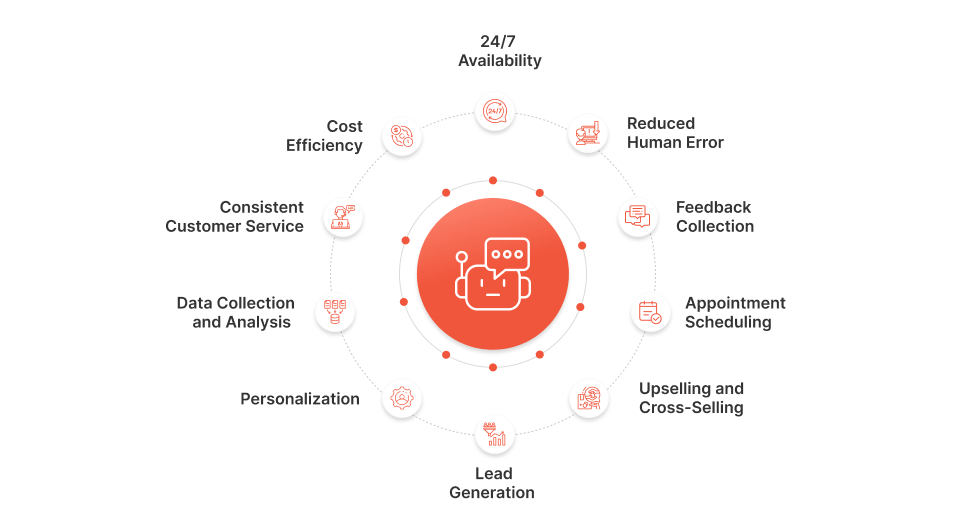
GPT-based vs. Transformer-based Models
While both GPT and Transformer models are based on transformer architecture, they differ in application:
Excited to Obtaining Your Data Science Certificate? View The Data Science Training Offered By ACTE Right Now!
AI Models for Different Use Cases
Role-based prompting assigns the AI a specific persona or Role, guiding it to respond with expertise or empathy. For example:
Interested in Pursuing Data Science Master’s Program? Enroll For Data Science Master Course Today!
Cost and Subscription Plans Comparison
Performance Benchmarks
AI performance is evaluated using benchmarks and key metrics such as accuracy, relevance, speed, and memory handling. Through Data science Training, models like GPT-4 and Gemini have achieved excellence in contextual accuracy and coherence, making them strong choices for natural language understanding and generation. Claude stands out with its faster response times, particularly in commercial settings. For multimodal tasks involving text, images, or audio, Gemini leads with its seamless processing capabilities. Additionally, GPT-4 supports larger context windows, which is advantageous for long-form content and complex conversations. Overall, benchmarking AI models helps organizations identify the most efficient and suitable solutions based on their specific needs.
Preparing for a Data Science Job Interview? Check Out Our Blog on Data Science Interview Questions & Answer
Customization and Fine-tuning Options
Customization and fine-tuning enable organizations to adapt AI models to their unique requirements:
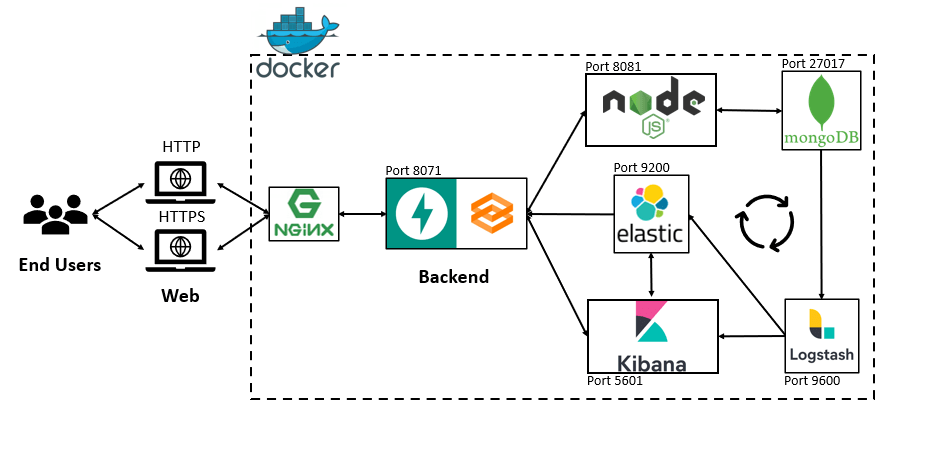
Offline AI Chatbot Solutions
Offline AI chatbots operate independently of internet connectivity, making them highly suitable for privacy-sensitive, secure, or remote environments where data protection and low latency are critical. Models like Meta’s LLaMA can be locally deployed on personal devices or enterprise infrastructure to enable offline text generation and interaction. Similarly, private GPT models such as GPT-J and GPT-Neo allow organizations to create powerful AI-driven assistants without exposing data to external servers. For larger-scale needs, companies often implement on-premise AI solutions, running models on internal servers to maintain strict control over data flow, security policies, and compliance standards. These implementations typically follow an AI Project Cycle that includes problem definition, data collection, model development, testing, deployment, and continuous monitoring for improvement. These offline AI deployments not only offer enhanced privacy and data sovereignty but also enable faster response times and full customization of model behavior—making them a compelling option for organizations prioritizing control, confidentiality, and performance.
Conclusion
As the AI landscape rapidly evolves, users and organizations have a growing array of chatbot and assistant models to choose from. From open-source innovations like LLaMA and Mistral to powerful commercial solutions such as Claude, Gemini, and Copilot, each option offers distinct advantages based on use case, customization needs, and budget. Open-source models prioritize flexibility, transparency, and cost-efficiency, making them ideal for developers and businesses seeking control and adaptability. Commercial AI chatbots, on the other hand, deliver high performance, robust support, and seamless integration with enterprise ecosystems—perfect for teams prioritizing reliability, ease of use, and Data Science Training. When selecting the right AI solution, factors like performance benchmarks, subscription costs, security, ethical considerations, and multimodal capabilities must be weighed carefully. Additionally, the ability to customize and fine-tune models is becoming essential for aligning AI behavior with specific organizational goals. Looking ahead, the future of AI chat assistants promises even more powerful, personalized, and multimodal experiences. As models become more context-aware and capable of operating across platforms including AR/VR and offline environments AI will continue to transform how we communicate, create, and work. Ultimately, the best AI assistant is the one that aligns with your technical, operational, and ethical priorities whether you’re building with open-source tools or leveraging cutting-edge commercial platforms.