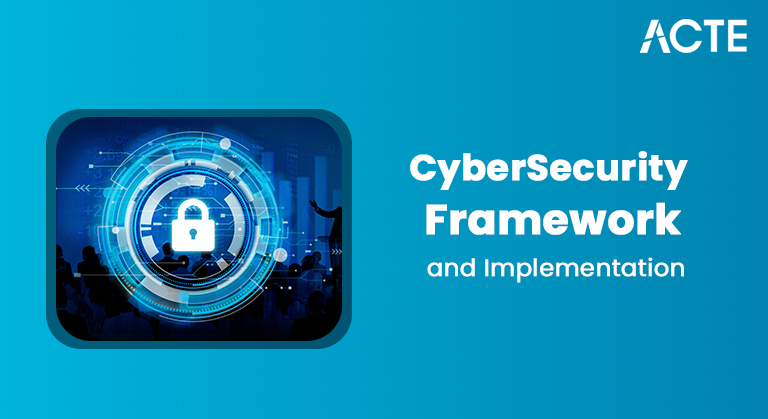
- Introduction
- Definition and Overview
- How Generative AI Works
- Applications of Generative AI
- Generative AI vs. Traditional AI
- Generative Adversarial Networks (GANs)
- Variational Autoencoders (VAEs)
- Generative AI in Image Creation
- Generative AI in Music and Audio
- Generative AI in Video and Animation
- Conclusion
Introduction
Generative Artificial Intelligence is one of the most exciting and rapidly growing fields in modern technology. Unlike traditional AI systems that simply analyze or predict based on input data, generative AI creates completely new content by learning from patterns found in existing data.This content can include anything from realistic images and human-like text to original music compositions, lifelike videos, and even Data Science Training . At its core, generative AI is powered by advanced machine learning models such as transformers, Generative Adversarial Networks, and Variational Autoencoders, all of which enable machines to simulate creativity and originality. These capabilities are not only reshaping the way we interact with technology but are also redefining what is possible across industries like entertainment, healthcare, marketing, education, and more. As these tools become more accessible and powerful, generative AI is opening the door to a new era of innovation, where machines collaborate with humans to enhance creativity and productivity in unprecedented ways.
Eager to Acquire Your Data Science Certification? View The Data Science Course Offered By ACTE Right Now!
Definition and Overview
Generative AI is a category of artificial intelligence models that create new content by learning patterns from existing data. Unlike traditional AI primarily focuses on classification and prediction, generative AI produces original content, such as text, images, music, and videos. It leverages neural networks and deep learning techniques to generate outputs that resemble human-created content. Popular generative AI models include GPT (for text), DALL·E (for images), and Jukebox (for music). Generative AI is transforming industries by enabling automated content creation, personalized experiences, and creative innovations, making it a revolutionary force in AI technology.
How Generative AI Works
Generative AI models use deep learning algorithms to analyze vast datasets and generate new content. The process involves:
- Training Phase: The model is trained on large datasets, learning the patterns, structures, and styles present in the data.
- Generation Phase: After training, the model uses the learned patterns to create new, original content based on prompts or random inputs.
- Refinement Phase: Generative AI uses feedback loops and fine-tuning techniques to improve content accuracy and coherence.
Key Machine Learning Techniques include transformer models, recurrent neural networks (RNNs), and convolutional neural networks (CNNs), which enhance content generation capabilities.
Applications of Generative AI
- Content Creation: Automated generation of articles, blogs, and marketing copy, improving productivity.
- Image and Video Generation: Creation of realistic images, deepfake videos, and digital art.
- Music and Audio Production: AI-powered tools generate music compositions, soundtracks, and voiceovers.
- Healthcare: Generative models simulate medical images for research and help discover drugs.
- Gaming and Entertainment: AI creates characters, environments, and storylines, enhancing gaming experiences.
- Generative AI is reshaping creative, technical, and scientific fields by automating complex tasks.
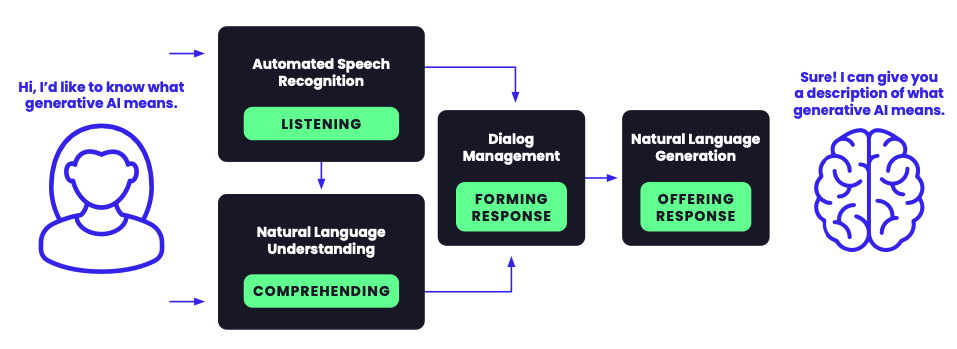
Generative AI vs. Traditional AI
Generative AI differs significantly from traditional AI in its functionality and purpose
- Traditional AI: Focuses on classification, prediction, and decision-making based on existing data. It follows strict rules and produces deterministic outputs.
- Generative AI: Creates new content by learning from data patterns. It produces creative and diverse outputs.
While traditional AI is used for Data Analysis, recommendation systems, and fraud detection, generative AI powers content creation, design, and simulation.
Generative Adversarial Networks (GANs)
Generative Adversarial Networks (GANs) are a key architecture in generative AI. They consist of two neural networks:
- Generator: Creates fake content based on the training data.
- Discriminator: Evaluates the generated content and distinguishes it from accurate data.
The generator continuously improves by trying to fool the discriminator, resulting in highly realistic outputs. GANs are widely used in Data science Training, as well as in:
- Image synthesis (e.g., generating realistic faces).
- Deepfake creation (e.g., altering facial expressions in videos).
- Data augmentation (e.g., creating synthetic datasets for model training).
- Encoding: Compressing input data into a more miniature, latent representation.
- Decoding: Reconstructing the original content from the latent space, introducing randomness to generate new content.
- Content Writing: Automating the creation of articles, blog posts, and reports.
- Conversational AI: Powering chatbots and virtual assistants with natural language capabilities.
- Language Translation: Enabling high accuracy of real-time language translation.
- Text Summarization: Generating concise summaries from large documents.
- Art and Design: Creating original digital artwork, logos, and illustrations.
- Marketing and Advertising: Generating custom visuals for ad campaigns.
- Fashion and Retail: Designing virtual products and clothing prototypes.
- Medical Imaging: Generating synthetic X-rays and MRI scans for training purposes.
- Deepfake Videos: Generating realistic face swaps and synthetic speech.
- Automated Animation: Creating animated characters and motion graphics.
- Video Editing: AI-powered tools automate color correction, transitions, and special effects.
- Marketing Videos: AI-generated videos for product promotions and explainer content.
Excited to Obtaining Your Data Science Certificate? View The Data Science Training Offered By ACTE Right Now!
Variational Autoencoders (VAEs)
Variational Autoencoders (VAEs) are another generative AI architecture that focuses on:
VAEs excel in image generation, style transfer, and anomaly detection. They produce outputs with smooth variations and are widely used in artistic and creative applications.
Generative AI in Text Generation
Generative AI models, such as GPT-4, Claude, and LLaMA, transform text generation by producing coherent and contextually relevant content. These models are used for:
Interested in Pursuing Data Science Master’s Program? Enroll For Data Science Master Course Today!
Generative AI in Image Creation
Generative AI redefines image creation through tools like DALL·E, MidJourney, and Stable Diffusion, which generate highly realistic and creative images from text prompts.
Applications include:
Generative Artificial Intelligence significantly reduces manual design efforts and promotes creative experimentation.
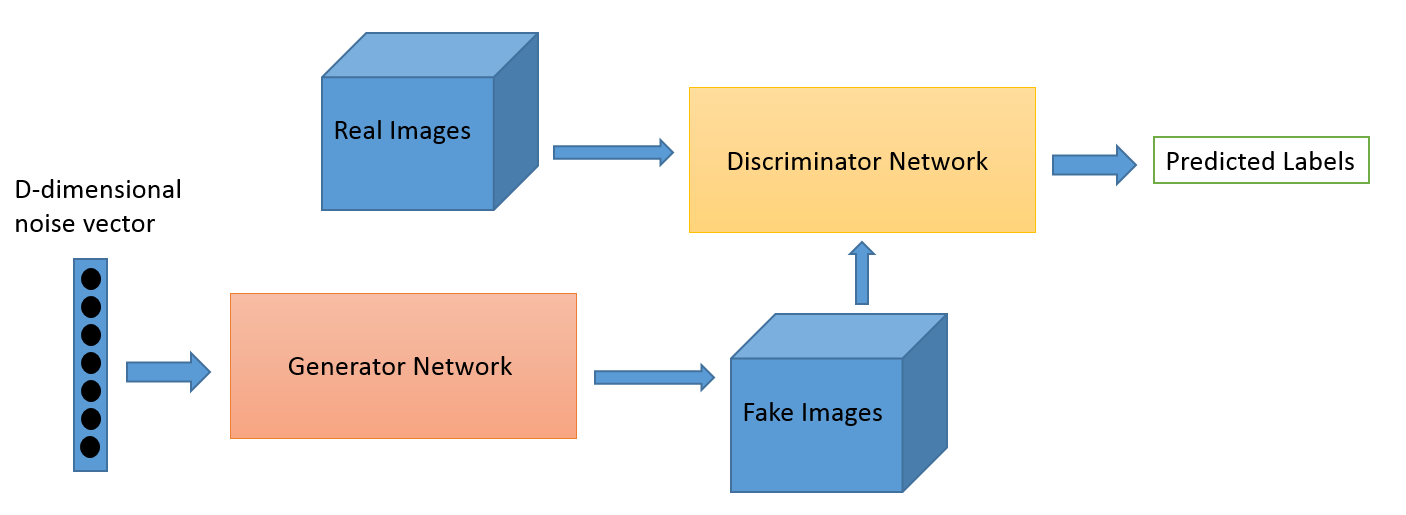
Generative AI in Music and Audio
Generative AI models like OpenAI’s Jukebox and Google’s MusicLM create music compositions and audio effects. These models generate melodies, lyrics, and soundtracks with impressive realism. Applications include Music Composition: Automating the creation of background scores and jingles. Voice Cloning: Generating synthetic voiceovers for advertisements and virtual assistants. Sound Design: Creating custom sound effects for films and games. Generative AI in music offers innovative tools for artists and content creators.
Preparing for a Data Science Job Interview? Check Out Our Blog on Data Science Interview Questions & Answer
Generative AI in Video and Animation
Generative AI, combined with Data Science, transforms video and animation production through tools like Runway ML, Synthesia, and Pika Labs, which create realistic video content from text or images.
Applications include:
Conclusion
Generative AI is more than just a technological breakthrough—it is a powerful creative partner that is transforming industries and redefining what machines can do. By learning from vast datasets and applying complex models, generative AI can produce content that is unique, personalized, and increasingly indistinguishable from human-made creations. From writing and illustration to music, video, and product design, the possibilities are expanding at an incredible pace. However, as with any powerful tool, it comes with important responsibilities. The challenges related to misinformation, intellectual property, data privacy, Data Science Training, and ethical use require thoughtful solutions and clear regulations. As the technology continues to evolve, its role in society will grow deeper, influencing how we communicate, create, and experience the world. The future of generative AI holds immense promise, offering tools that not only make our work easier but also help us explore new forms of expression and innovation.