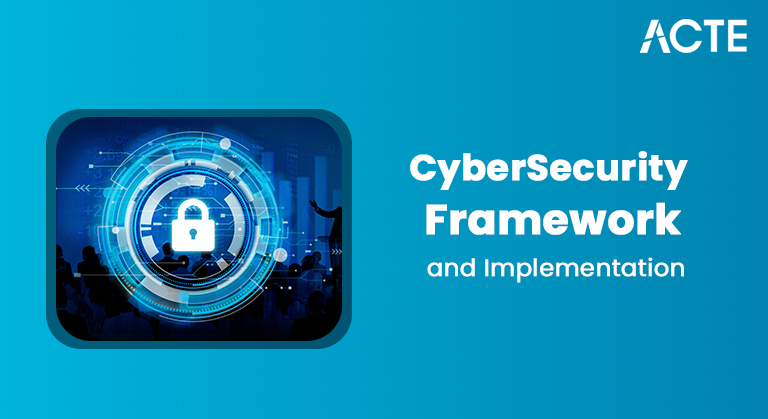
- Introduction to Data Science
- What is Artificial Intelligence
- What is the distinction between Artificial Intelligence and Machine Learning
- Machine Learning and Its Types
- What is Deep Learning
- Working on a Deep Neural Network
- Deep Learning vs Machine Learning
- Conclusion
Introduction to Data Science
Present-day international uses statistics as fuel; statistical technological know-how drives special technology toward automation. In other words, statistical technological know-how is the look at statistics. Students look at Data Science academics for novices of their toddler steps to visualize and examine statistics. We use diverse statistical methods to read and visualize many statistics. Thus, records are the coronary heart of Data Science, Deep Learning, Artificial Intelligence, and Machine Learning.
Eager to Acquire Your Data Science Certification? View The Data Science Course Offered By ACTE Right Now!
What is Artificial Intelligence?
“Artificial Intelligence is the imposition of human intelligence on robots or machines, especially PC systems. It includes a three-step process and is closely related to fields like Data Science Training, which provides the foundational skills for analyzing data and developing intelligent algorithms.
- Learning: The retrieval of statistics and constructing of regulations for education machines.
- Reasoning: Employing the built regulations to achieve correct results.
- Self-correction: Applying algorithms and strategies to enhance the overall performance and accuracy of the machines iteratively.
Artificial Intelligence includes diverse frameworks that may be hired for computations in deep neural networks. TensorFlow, Keras, PyTorch, Theano, Accord.NET, Spark MLlib, Scikit-learn, and Microsoft CNTK are famous frameworks.
What is the distinction between Artificial Intelligence and Machine Learning
Artificial Intelligence is a panoptic period below which Machine Learning and Deep Learning reside. The diagram below suggests the types of Artificial Intelligence through which we will effortlessly visualize the connections and variations between AI and Machine Learning. Let’s first recognize the types of Artificial Intelligence before discussing the varieties of Machine Learning and concluding the Artificial Intelligence vs. Machine Learning discourse, as understanding these concepts is essential in Data science Training.
Categories of Artificial Intelligence
Weak Artificial Intelligence: In vulnerable AI, a gadget’s response to a particular input is well-described. Here, we create a fixed set of policies for the gadget. It is then sure to respond to steps with limited policies. The supervised Machine Learning approach comes below Weak Artificial Intelligence.
Example: If we train an espresso gadget to offer a Cappuccino, it will put well-described sequences of actions. It will now not reply past the beyond of policies that we’ve pre-described.
Strong Artificial Intelligence: In robust AI, the algorithms and commands for a gadget are designed to give the gadget the potential to analyze itself from the given inputs and iteratively improve accuracy through experience.
Examples: Google Translate, Google Maps, AI Chatbots, advice engines, etc., are real-life examples of robust Artificial Intelligence.
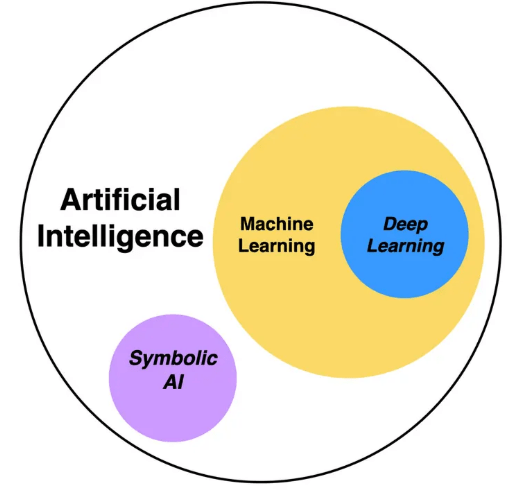
Machine Learning and Its Types
Machine Learning is a fraction of Artificial Intelligence that entails modeling algorithms; those algorithms inject skills into a gadget for performing awesome duties without being explicitly programmed. Before diving into the varieties of Machine Learning, if we speak approximately Machine Learning vs statistics, we’d believe the truth that visualization isn’t feasible without acting correct statistical analyses. With the assistance of statistics, we will draw beneficial insights from statistics and construct powerful Machine Learning fashions on the pinnacle of it.
Type of Machine Learning | Description | Example |
---|---|---|
Supervised Learning | Uses a labeled dataset with input and output parameters. Algorithms learn from this data to predict outputs for new, unseen inputs. | Weather forecasting: Predicts conditions using inputs like humidity, temperature, wind, atmospheric pressure, and precipitation. |
Unsupervised Learning | Displaying non-editable reference data. | Collaborative data like project updates or shared resources. |
Data Protection | No labeled data. Algorithms analyze and find patterns in the input data using techniques like clustering or dimensionality reduction. | Clustering fruits: Inputs like Apple, Banana, Orange are grouped based on features without prior labeling; identifies similarity to form clusters. |
Reinforcement Learning | Algorithms learn to make decisions by interacting with an environment, receiving rewards or punishments for their actions. | Maze game: A player receives −10 points for wrong moves and +10 for correct ones. Over time, the system learns the optimal path to escape the maze. |
What is Deep Learning?
Deep Learning is a subset of Machine Learning in which we construct algorithms that comply with a layered architecture. The layered architecture (deep neural community) in Deep Learning is stimulated by the human organic neural community. It is called a Deep Neural Network because the device is built with the assistance of a dense neural community. The system’s Learning is achieved by utilizing this deep neural community. The underneath photograph of Deep Learning vs Machine Learning suggests the distinction inside the mechanisms of each field.
Ready to Earn Your Data Science Certificate? View The Data Science Course Offered By ACTE Right Now!
Working on a Deep Neural Network
- Initially, we will offer the entered dataset to the neural community. Then, the enter records enter right into a neuron. The entry is extended with a corresponding weight cost. This is related to every entry. A weight cost is used to expose the power of the hyperlink among synthetic neurons.
- The final results of this augmentation keep transporting to the following layer and act because of the entry for that layer. This manner is iterated for each layer of the community.
- After that, on the output layer, the neural community yields a real cost for the regression task. For classification, the neural community offers each class the chance. Then, using the computation algorithms, all the neurons’ weights are updated iteratively.
Are You Considering Pursuing a Data Science Master’s Degree? Enroll For Data Science Master Course Today!
- Finally, while the corresponding values of the weights deliver the output close to the real, the neural community is thoroughly skilled. Interestingly, a completely skilled neural community can figure out an entity with extra performance compared with an ordinary neural community.
- In Machine Learning, we can educate the algorithms using a small number of records. But in Deep Learning, we need many records to comprehend a brand-new concept.
- Furthermore, Machine Learning provides a faster-skilled model, even as Deep Learning fundamentals fashions take a long time for schooling. The benefits of Deep Learning over Machine Learning are excessive accuracy and automatic characteristic selection.
- In Deep Learning, a neural community learns the choice of good-sized capabilities using itself. But in Machine Learning, we want to pick out the mod’s capabilities manually.
Set to Ace Your Microsoft Azure Job Interview? Check Out Our Blog on Data Science Interview Questions & Answer
Deep Learning vs Machine Learning
Machine Learning (ML) and Deep Learning (DL) are each subset of synthetic Intelligence. However, they vary in complexity, approach, and application. Machine Learning includes algorithms that permit computer systems to analyze styles from records and make predictions or choices without being explicitly programmed. It is based on records and calls for human intervention for characteristic extraction—that means that a records scientist should determine which capabilities or variables the version has to consider. Standard ML algorithms consist of choice trees, aid vector machines, and linear regression, and they may be broadly utilized in programs like junk mail detection, advice structures, and fraud detection.
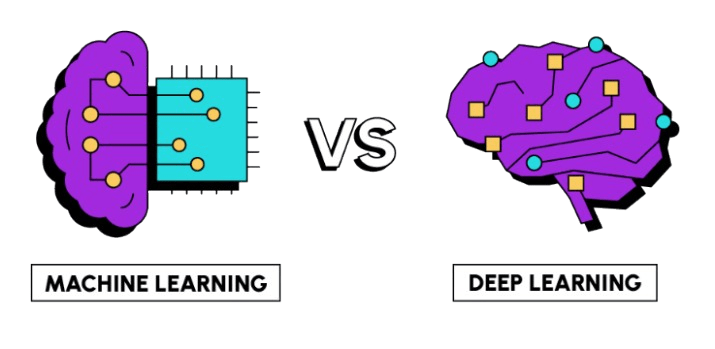
On the other hand, Deep Learning is a specialized department of the system getting to know that uses synthetic neural networks intense neural networks with many layers to robotically analyze representations from significant volumes of unstructured records like images, audio, and text. Getting to know fashions can carry out automated characteristic extraction and are relatively powerful at dealing with complicated obligations, including picture recognition, herbal language processing, and self-using automobile structures. However, deep getting-to-know generally calls for extra records and computing electricity compared to conventional system getting-to-know strategies. In summary, while system learning is appropriate for many predictive obligations and regularly works nicely with smaller, based datasets, deep Learning is ideal for big-scale, complicated troubles regarding unstructured records. Both tactics are valuable, and their selection depends on the unique use case, records availability, and computational resources.
Conclusion
In conclusion, Data Science, Machine Learning (ML), Deep Learning (DL), and Artificial Intelligence (AI) are interrelated fields. However, every has its specific scope and purpose. Artificial Intelligence is the broadest concept, targeted at developing machines that may mimic human Intelligence. Within AI, Machine Learning is a subset that permits structures to analyze from records and enhance through the years without being explicitly programmed. Deep Learning is a similar subset of ML that uses layered neural networks to technique significant quantities of unstructured records and remedy extra complicated troubles with better accuracy. On the other hand, data science is a multidisciplinary discipline that uses strategies from statistics, computer science, and domain knowledge to extract insights from data, and Data Science Training equips individuals with these essential skills. It regularly employs AI, ML, and DL strategies to investigate and interpret big datasets. In easy terms, AI is the goal (clever machines), ML is one of the gears to acquire it, DL is an extra superior model of that tool, and Data Science is the wider exercise that makes use of all of them, alongside different strategies, to make records-pushed choices.