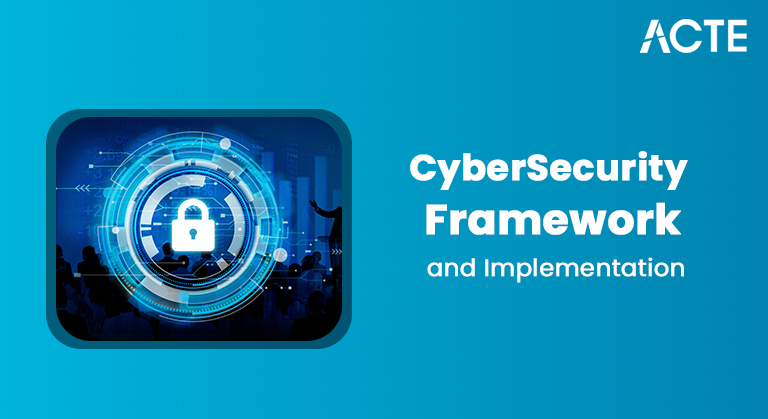
- Introduction
- What is Data Science?
- What is Data Analytics?
- Differences Between Data Science and Data Analytics
- Core Skills: Data Science vs. Data Analytics
- Tools Used in Data Science and Data Analytics
- Processes in Data Science
- Processes in Data Analytics
- Conclusion
Introduction
In today’s ever-evolving world, data has become a cornerstone of decision-making in nearly every industry. As organizations seek to harness the power of their data, the fields of Data Science and Data Analytics have emerged as pivotal forces. Both disciplines share common ground in that they rely on data to extract valuable insights, but they serve distinct roles and employ different methodologies. Understanding the differences between Data Science and Data Analytics is crucial for anyone looking to pursue a career in these fields, as well as for businesses determining which approach best suits their needs. While Data Science, which often involves Data Science Training, is a broad, multifaceted field focused on leveraging advanced techniques such as machine learning, artificial intelligence, and predictive modeling to extract complex insights and build models for future predictions, Data Analytics is more focused on examining historical data to help organizations make informed decisions. The key difference lies in the scope and the methodologies applied in each field. This article will delve into the core concepts of Data Science and Data Analytics, explore their processes, compare the skills required for each, and identify the tools that help professionals in these domains succeed. We’ll also examine career prospects, salary comparisons, and the future trajectory of both fields to help you determine which path may be right for you.
Eager to Acquire Your Data Science Certification? View The Data Science Course Offered By ACTE Right Now!
What is Data Science?
Data technology is a multidisciplinary discipline that uses several techniques, algorithms, and structures to extract precious insights from each prepared and unstructured statistic. Statistical approaches, programming, and gadgets gaining knowledge are used to remedy complicated, demanding situations and bring statistics-pushed predictions. To forecast destiny tendencies, statistics scientists regularly paint large numbers of statistics, extracting and cleansing them earlier than gadgets to gain knowledge of fashions. Examples of statistics technology merchandise, which are based on the Basics of Data Science, include Google Maps, which calculates the time it’ll take you to reach your location, and Netflix, which recommends films based on your viewing history
Data Science Process
- Data Collection: Statistics are collected using various sources, such as databases, APIs, and net scraping.
- Data Exploration: Data scientists use exploratory statistics evaluation (EDA) to search for tendencies and abnormalities inside the statistics.
- Model Development: To offer predictions, gadgets gaining knowledge of fashions are created.
- Model Deployment: The fashions make real-time, statistics-pushed picks.
What is Data Analytics?
Data analytics involves reading statistics to discover actionable insights that help agencies make knowledgeable decisions. Its predominant objectives are commonly analyzing ancient statistics and generating reviews that inform operational and strategic picks. Statistics Analytics uses several statistical tools, regularly together with statistics visualization applications like Tableau and Power BI, to discover trends and styles in statistics. For instance, an enterprise may use income records and consumer evaluations to improve its products and services.
Data Analytics Process
- Data collection: Gathering essential statistics from more than a few sources, such as files, databases, and surveys.
- Data cleansing: It prepares statistics for evaluation through cleansing and organizing.
- When evaluating exploratory statistics, analysts look for essential trends, correlations, and insights in the data.
- Data visualization: This is the process of displaying the outcomes graphically, such as dashboards and reviews, to assist stakeholders in making decisions that can be supported.
- Web Scraping and Data Collection There are various records. As they are sent from multiple sources inside the Dwithine manner, they are processed using Datusing, internet scraping, and APIs.
- Data Management After collecting the Data, specialists focus on dealing with and organizing the records (Data Management). This consists of sports that include record integration, record transformation, record cleaning, and managing lacking values.
- Exploratory Data Analysis (EDA) EDA is a device that Data Scientist use to examine record traits and locate patterns, anomalies, and correlations. This step uses record visualization software and different massive statistical strategies to gain insights.
- Model Development The records scientists lay out a descriptive or predictive version primarily based on gadgets gaining knowledge of algorithms and statistical strategies. It includes evaluating, educating, choosing the best version, and developing functions to make better predictions.
- Model Deployment The records scientists lay out a descriptive or predictive version primarily based on gadgets gaining knowledge of algorithms and statistical strategies. It includes evaluating, educating, choosing the best version, and developing functions to make better predictions.
Excited to Obtaining Your Data Science Certificate? View The Data Science Training Offered By ACTE Right Now!
Differences Between Data Science and Data Analytics
Data science is a vast subject that specializes in extracting significant insights from based and unstructured information through the use of superior strategies, system studying, synthetic intelligence, and predictive modeling. It entails information collection, cleaning, processing, and using complicated algorithms to discover hidden styles and trends. Data scientists focus on constructing fashions that assist in forecasting and decision-making, frequently requiring deep programming, data, and arithmetic know-how. Data Analytics, on the other hand, is a unique subset of information technological know-how that specializes in studying historical information to discover trends, styles, and insights that assist in making commercial enterprise decisions. It offers based information and uses statistical strategies, information visualization, and commercial enterprise intelligence gear. Data analysts commonly paint with gear like SQL, Excel, and visualization software programs like Tableau or Power BI to generate reviews and dashboards that guide operational and strategic decisions. While each field involves running with information, information technology is more research-orientated and entails predictive modeling, while information analytics is more targeted at deciphering present information for actionable insights.
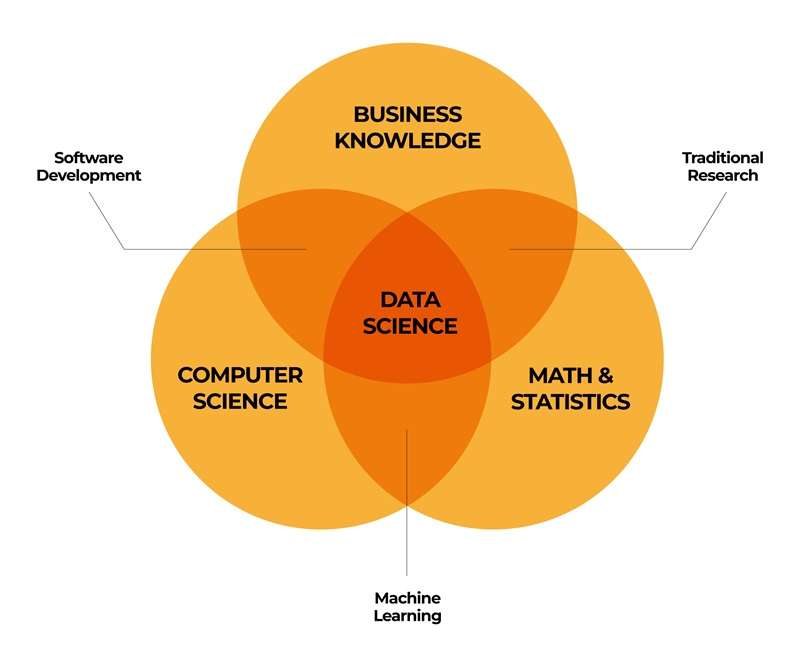
Core Skills: Data Science vs. Data Analytics
We have long gone through some LinkedIn activity descriptions for Data Scientists and Data Analysts. Below is the simple ability requirement schema accompanied through principal organizations:
Data Scientist Skills
An information scientist calls for various talents that integrate technical understanding, analytical thinking, and area know-how. One of the middle talents is programming, with scalability in languages like Python Programming, R, or SQL, which is critical for information manipulation, evaluation, and constructing system studying fashions. Additionally, data and arithmetic play an essential function in knowledge information distributions, probability, and speculation testing, which assist in making information-pushed decisions.
Data Analyst Skills
An information analyst desires a sturdy basis in information manipulation and querying, with scalability in SQL to extract and manipulate massive datasets efficiently. They additionally require an understanding of Excel and spreadsheets, which are typically used for information cleaning, evaluation, and reporting. The statistical assessment know-how is also essential for deciphering information trends, acting speculation testing, and making information-pushed decisions.
Tools Used in Data Science and Data Analytics
Data Science Tools Data technological know-how includes a lot of gear for records processing, gaining knowledge, and visualization. Python and R are the most broadly used programming languages because of their massive libraries like Pandas, NumPy, Scikit-learn, and TensorFlow for record manipulation and gaining knowledge. Jupyter Notebook and Google Colab are famous environments for writing and trying out code. Big records gear, which includes Apache Hadoop and Spark, efficiently assists in handling massive datasets. Cloud systems like AWS, Google Cloud, and Azure offer scalable computing energy for Data science Training, model education, and deployment. Data visualization tools like Matplotlib, Seaborn, and Plotly permit powerful presentation of insights.
Data Analytics Tools Data analytics is based on gear for querying, visualization, and commercial enterprise intelligence. SQL is essential for extracting and dealing with dependent records from databases. Excel is usually used for records cleaning, analysis, and reporting. BI gear like Tableau, Power BI, and Looker assist in creating interactive dashboards and reports. Analysts use SPSS, SAS, or Python (with Pandas and NumPy) for statistical analysis. Additionally, Google Analytics is broadly used to study Internet, advertising, and marketing records. Unlike records technological know-how gear, which regularly focuses on predictive modeling, analytics gear emphasizes fashion identification, reporting, and real-time records monitoring.
Interested in Pursuing Data Science Master’s Program? Enroll For Data Science Master Course Today!
Processes in Data Science
Preparing for a Data Science Job Interview? Check Out Our Blog on Data Science Interview Questions & Answer
Processes in Data Analytics
Data Collection The first step of the records analytics procedure is Data Collection from numerous sources. This can also encompass records extraction from flat files, databases, or APIs. Other techniques for collecting records include surveys and guided record entry.
Data Cleaning It largely involves preparing records for evaluation and cleaning. This activity is especially done with the aid of records analysts. This generally offers lacking values, elimination of duplicates, standardization of formats, and solving inconsistencies in records.
Exploratory Data Analysis and KPI Configuration In an exploratory records evaluation procedure, records analysts search for patterns, trends, or even correlations within the records and for traits of the records set. They additionally set up specific indicators, called key overall performance indicators (KPIs), to tune a particular metric applicable to an investigation’s goals.
Analyzing Data with Power BI and Tableau Power BI and Tableau are technologies utilized by records analysts to perform complete records evaluation, growing visualizations and reviews, and dashboards, which can assist them in creating insightful reviews. Such dynamic and interactive visualizations can help make selections based on records.
Creating Presentations for Communicating Findings to Stakeholders Data analysts create reviews and displays to successfully communicate their understandings and consequences to the control teams, customers, and different stakeholders. At this point, the important findings are outlined, illustrations are provided, and beneficial guidelines grounded in the evaluation are made.
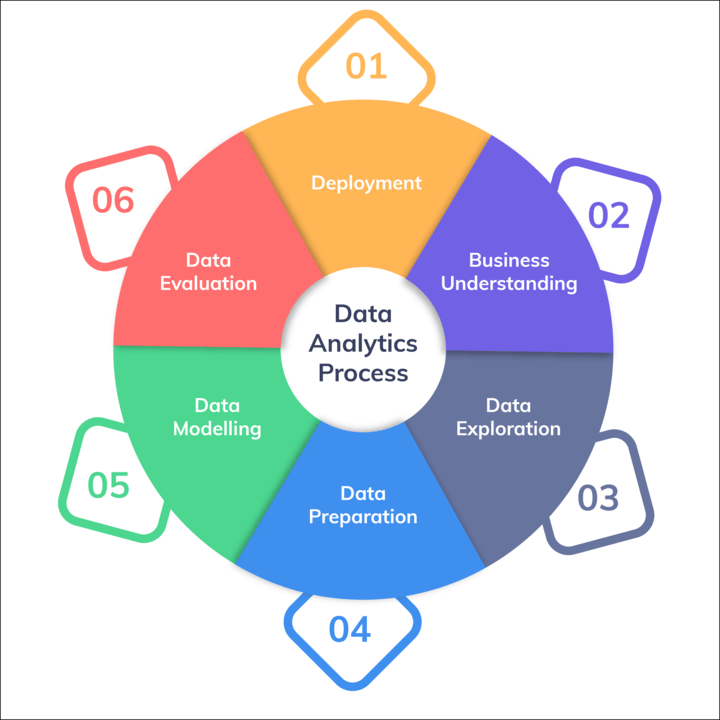
Conclusion
In today’s data-driven environment, where businesses and organizations are inundated with vast amounts of information, data analytics and data science play distinct yet equally essential roles in extracting valuable insights and driving decision-making. While data analytics is primarily focused on examining historical data to identify trends, patterns, and anomalies, its primary goal is to optimize operations, improve efficiency, and inform immediate business decisions. Through methods like descriptive analytics, statistical analysis, and data visualization, analysts can present businesses with a clearer picture of past performance and present actionable recommendations. On the other hand, Data Science Training goes beyond just examining existing data and instead focuses on solving complex problems and predicting future outcomes. Leveraging techniques such as machine learning, artificial intelligence, and predictive modeling, data scientists are able to identify hidden patterns, develop automated decision-making models, and make data-driven forecasts that can guide strategic decisions. The field is more exploratory, involving the creation of new algorithms and data models to address challenges and identify opportunities that were previously out of reach. In essence, while data analytics is more focused on historical data analysis to improve operational efficiency and support real-time decision-making, data science is instrumental in driving innovation, solving complex problems, and shaping future strategies through predictive analysis and advanced technology. Both fields are critical in helping organizations leverage data effectively, ensuring long-term growth and a competitive edge in a constantly evolving market.